Empirical Study on the Effect of Residual Networks on the Expressiveness of Linear Regions
ARTIFICIAL NEURAL NETWORKS AND MACHINE LEARNING, ICANN 2023, PART X(2023)
摘要
Residual networks have achieved success across various industries. Currently, the research on the working mechanism of residual networks mainly focuses on shallow sub-networks, while knowledge about many other aspects remains limited. Deep neural networks based on the ReLU (Rectified Linear Unit) activation function partition the input space into piecewise linear regions, and thus, for a residual network with ReLU activation, the number of linear regions can quantify its expressive power. In this paper, we first visualize the linear regions of residual networks in two dimensions to understand how the number of linear regions evolves in residual networks. Moreover, we aim to compare the actual expressive power and input representation capabilities of residual networks by analyzing the number of linear regions in two-dimensional inputs between residual networks and non-residual networks. Our research findings indicate that, under consistent external parameters and conditions, residual networks generally exhibit stronger linear regions expression and input representation capabilities than non-residual networks in most cases.
更多查看译文
关键词
Residual networks,Linear regions,Expressiveness
AI 理解论文
溯源树
样例
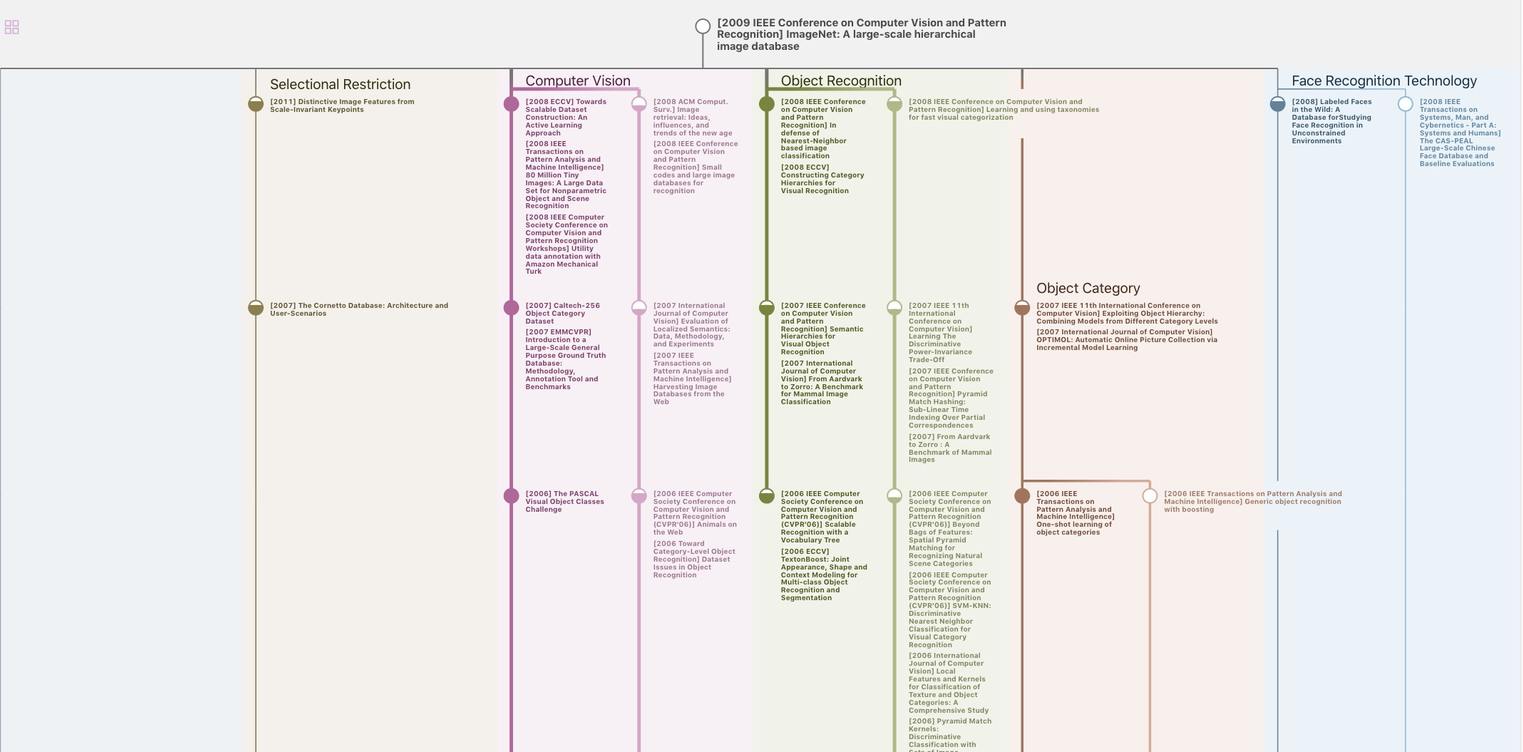
生成溯源树,研究论文发展脉络
Chat Paper
正在生成论文摘要