Interaction of Generalization and Out-of-Distribution Detection Capabilities in Deep Neural Networks
ARTIFICIAL NEURAL NETWORKS AND MACHINE LEARNING, ICANN 2023, PART X(2023)
摘要
Current supervised deep learning models are shown to achieve exceptional performance when data samples used in evaluation come from a known source, but are susceptible to performance degradations when the data distribution is even slightly shifted. In this work, we study the interaction of two related aspects in this context: (1) out-of-distribution (OOD) generalization ability of DNNs to successfully classify samples from unobserved data distributions, and (2) being able to detect strictly OOD samples when observed at test-time, finding that acquisition of these two capabilities can be at odds. We experimentally analyze the impact of various training data related texture and shape biases on both abilities. Importantly, we reveal that naive outlier exposure mechanisms can help to improve OOD detection performance while introducing strong texture biases that conflict with the generalization abilities of the networks. We further explore the influence of such conflicting texture bias backdoors, which lead to unreliable OOD detection performance on spurious OOD samples observed at test-time.
更多查看译文
关键词
Deep neural networks,generalization,out-of-distribution detection,outlier exposure,texture and shape bias
AI 理解论文
溯源树
样例
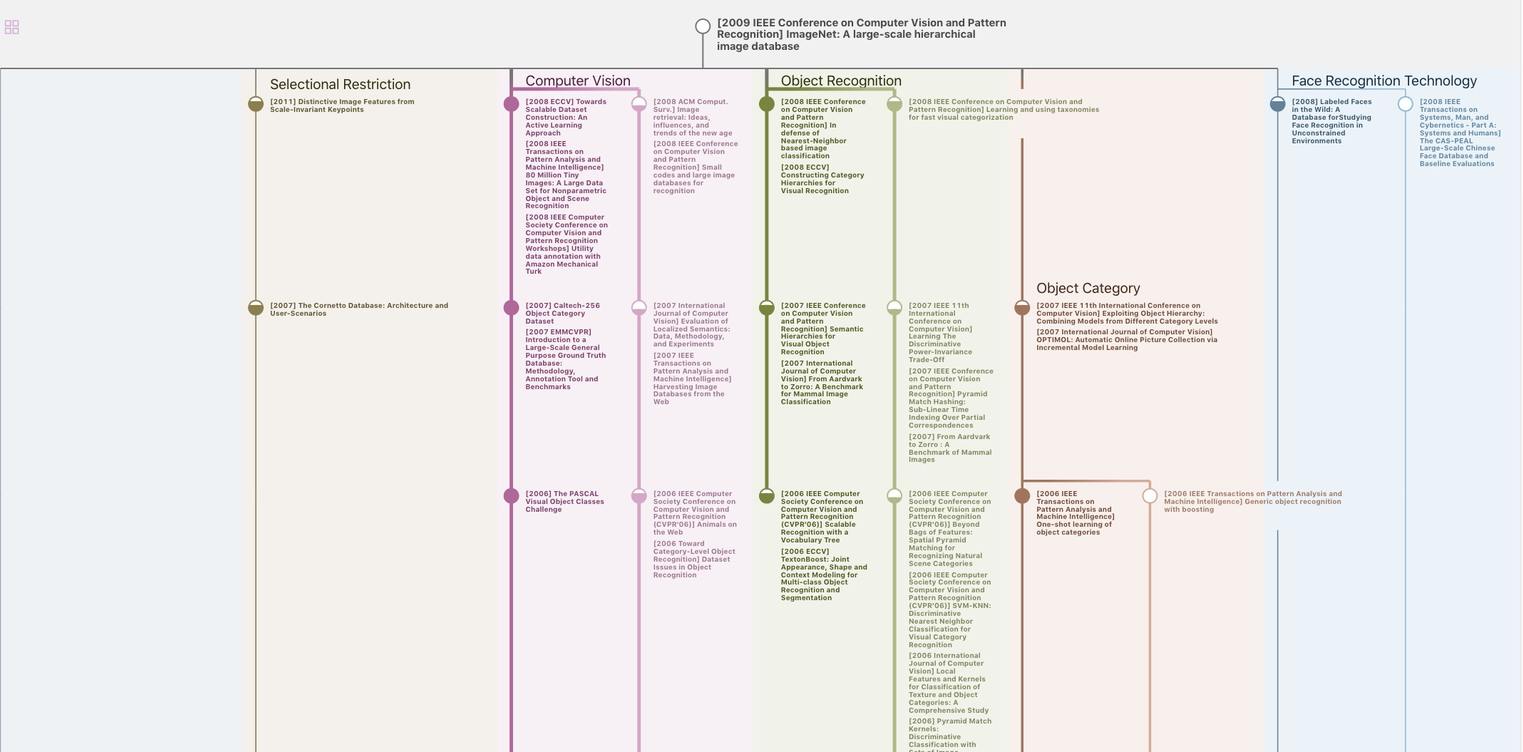
生成溯源树,研究论文发展脉络
Chat Paper
正在生成论文摘要