Malicious Domain Detection Based on Self-supervised HGNNs with Contrastive Learning
ARTIFICIAL NEURAL NETWORKS AND MACHINE LEARNING, ICANN 2023, PT III(2023)
摘要
The Domain Name System (DNS) facilitates access to Internet devices, but is also widely used for various malicious activities. Existing detection methods are mainly classified into statistical feature-based methods and graph structure-based methods. However, highly hidden malicious domains can bypass statistical feature-based methods, and graph structure-based methods have limited performance in the case of extremely sparse labels. In this paper, we propose a malicious domain detection method based on self-supervised HGNNs with contrastive learning, which can make full use of unlabeled domain data. Specifically, we design a hierarchical attention mechanism and a cross-layer message passing mechanism in the encoder for discovering more hidden malicious domains. Then, we construct a node-level contrastive task and graph-level similarity task to pre-train high-quality domain representations. Finally, we classify domains by fine-tuning the model with a small number of domain labels. Extensive experiments are conducted on the real DNS dataset and the results show that our method outperforms the state-of-the-art methods.
更多查看译文
关键词
Malicious Domain Detection,Self-supervised Learning,Contrastive Learning
AI 理解论文
溯源树
样例
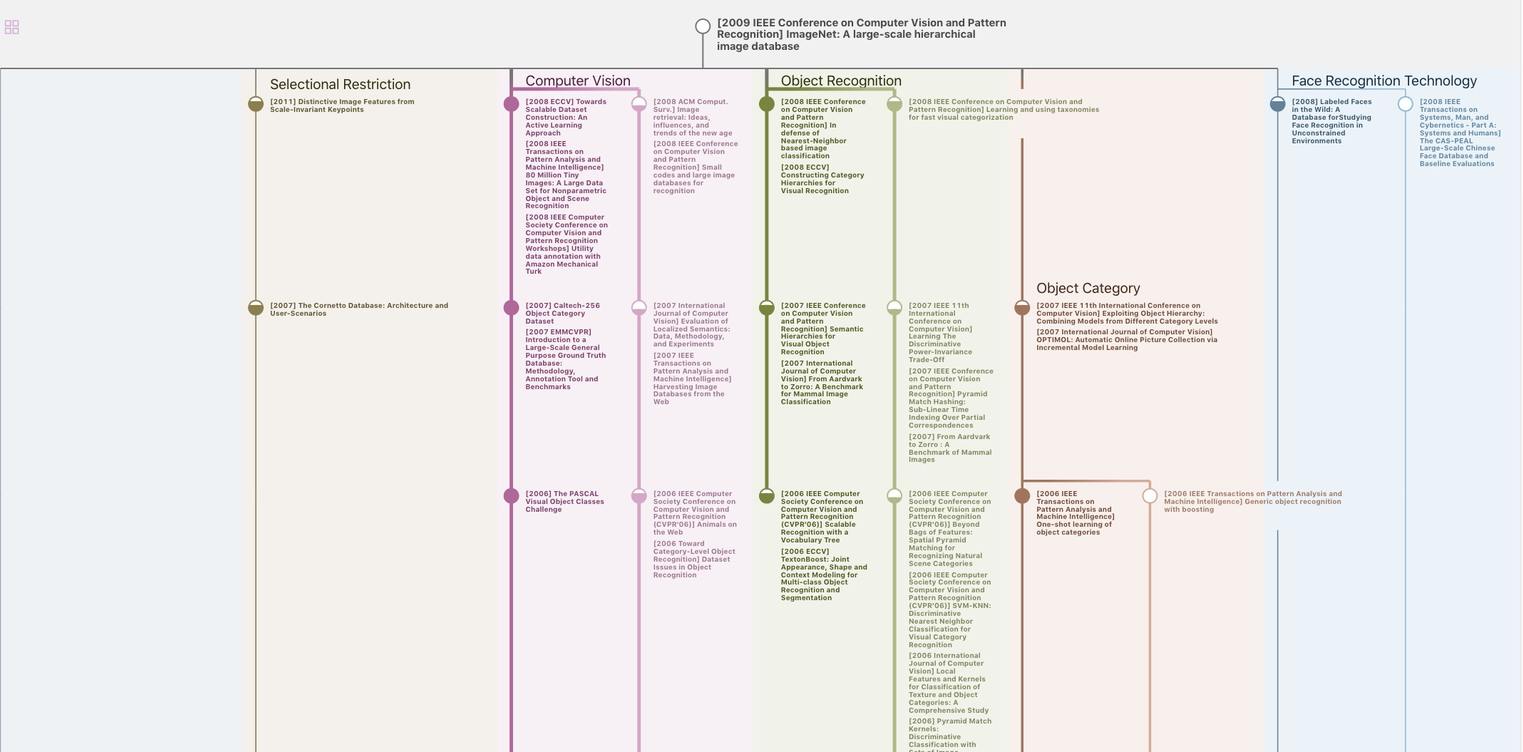
生成溯源树,研究论文发展脉络
Chat Paper
正在生成论文摘要