SimCLR-Inception: An Image Representation Learning and Recognition Model for Robot Vision.
Pattern Recognition: 7th Asian Conference, ACPR 2023, Kitakyushu, Japan, November 5–8, 2023, Proceedings, Part I(2023)
摘要
Effective feature extraction is a key component in image recognition for robot vision. This paper presents an improved contrastive learning-based image feature extraction and classification model, termed SimCLR-Inception, to realize effective and accurate image recognition. By using the SimCLR, this model generates positive and negative image samples from unlabeled data through image augmentation and then minimizes the contrastive loss function to learn the image representations by exploring more underlying structure information. Furthermore, this proposed model uses the Inception V3 model to classify the image representations for improving recognition accuracy. The SimCLR-Inception model is compared with four representative image recognition models, including LeNet, VGG16, Inception V3, and EfficientNet V2 on a real-world Multi-class Weather (MW) data set. We use four representative metrics: accuracy, precision, recall, and F1-Score, to verify the performance of different models for image recognition. We show that the presented SimCLR-Inception model achieves all the successful runs and gives almost the best results. The accuracy is at least 4 % improved by the Inception V3 model. It suggests that this model would work better for robot vision.
更多查看译文
关键词
recognition model,image representation learning,vision,simclr-inception
AI 理解论文
溯源树
样例
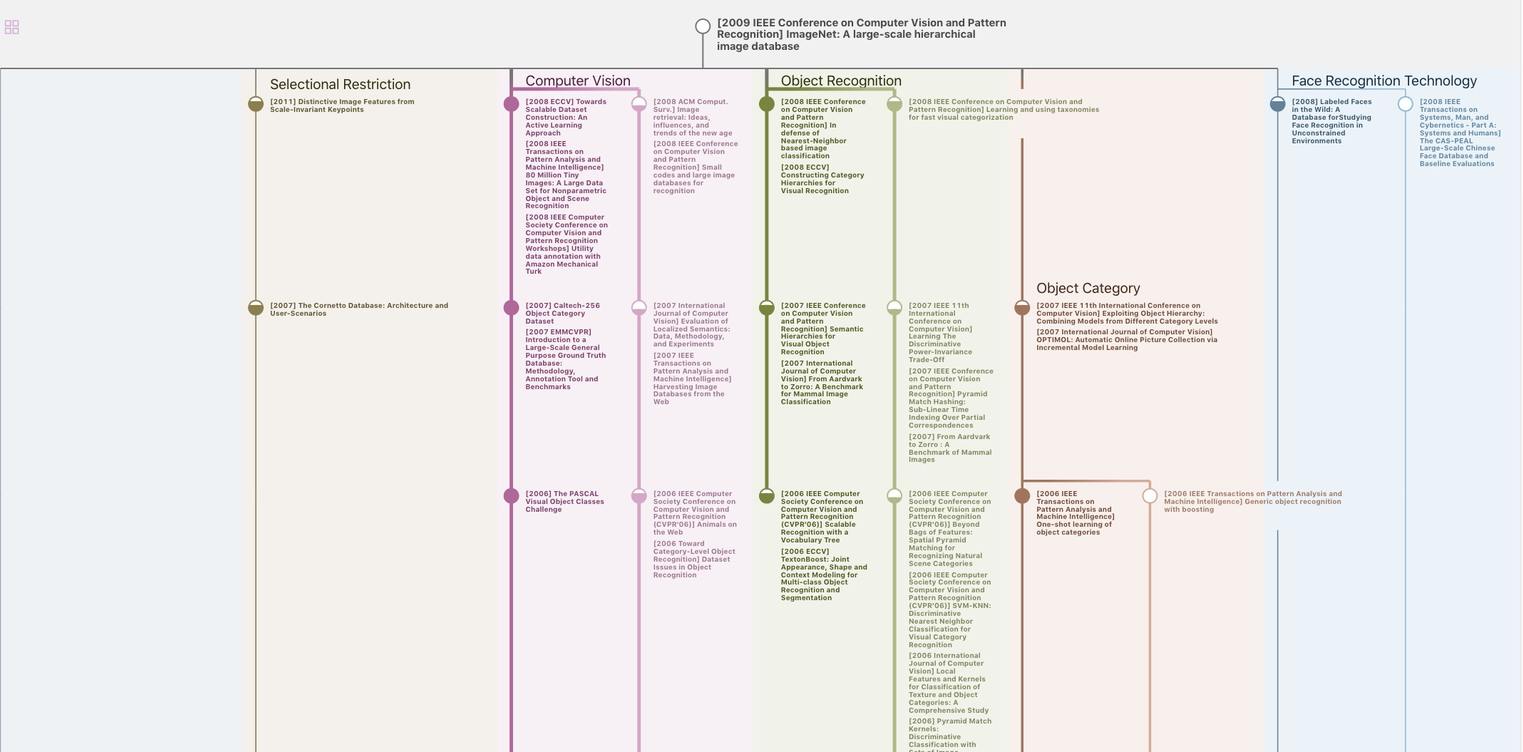
生成溯源树,研究论文发展脉络
Chat Paper
正在生成论文摘要