On Learning When to Decompose Graphical Models.
Learning and Intelligent Optimization: 17th International Conference, LION 17, Nice, France, June 4–8, 2023, Revised Selected Papers(2023)
摘要
Decomposition is a well-known algorithmic technique for Graphical Models. It is commonly believed that such a technique is cost-effective for instances with low width. In this paper, we show on a large data set of real-life inspired instances that this is not the case. To better understand this result, we narrow our study and consider k -tree instances where the width is well controlled and get similar results. Finally, we show that by adding a few simple features and using simple Machine Learning models we can predict the convenience to decompose with an accuracy of more than 85 % , which produces time reductions in standard benchmarks of nearly 90 % .
更多查看译文
关键词
decompose graphical models,learning
AI 理解论文
溯源树
样例
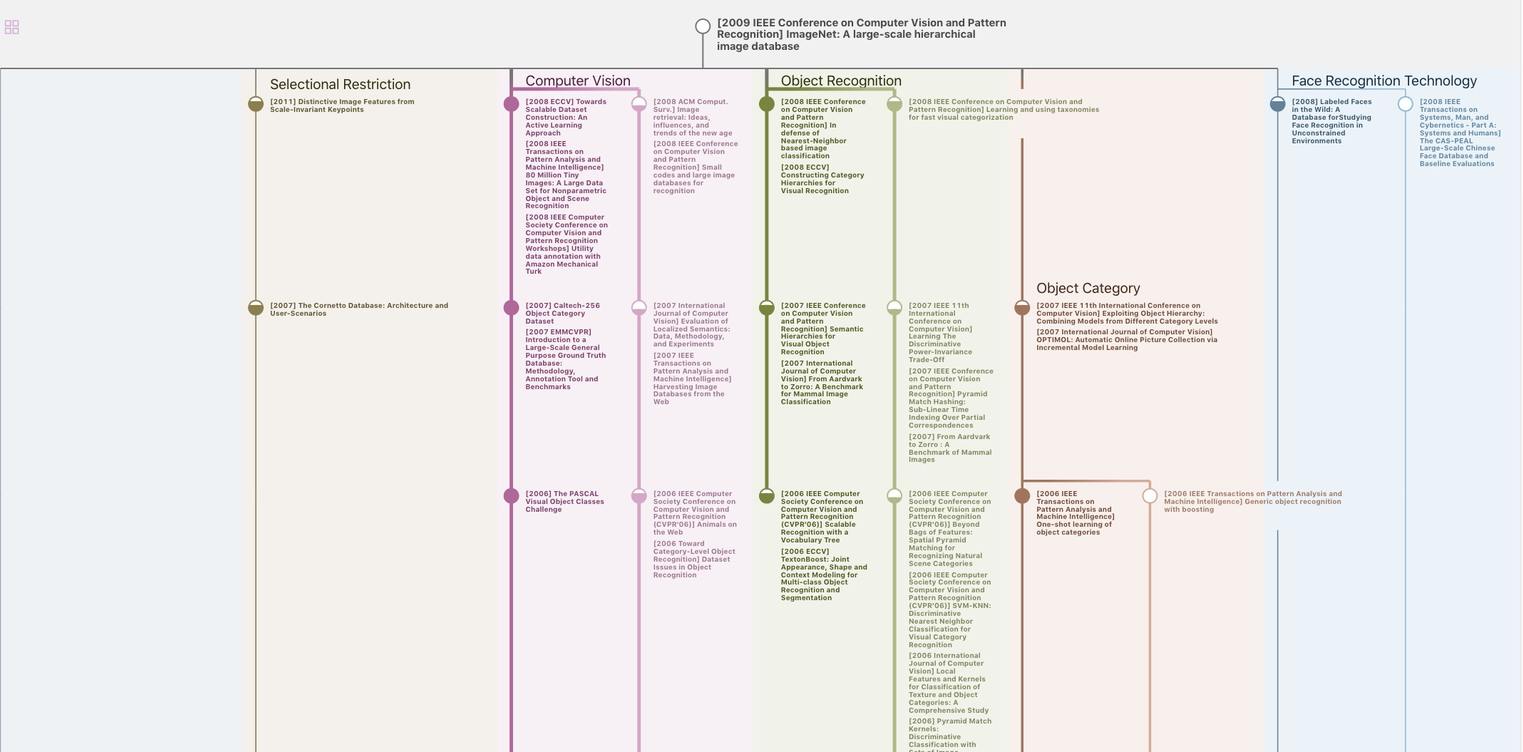
生成溯源树,研究论文发展脉络
Chat Paper
正在生成论文摘要