Relational Graph Attention-Based Deep Reinforcement Learning: An Application to Flexible Job Shop Scheduling with Sequence-Dependent Setup Times.
Learning and Intelligent Optimization: 17th International Conference, LION 17, Nice, France, June 4–8, 2023, Revised Selected Papers(2023)
摘要
This paper tackles a manufacturing scheduling problem using an Edge Guided Relational Graph Attention-based Deep Reinforcement Learning approach. Unlike state-of-the-art approaches, the proposed method can deal with machine flexibility and sequence dependency of the setup times in the Job Shop Scheduling Problem. Furthermore, the proposed approach is size-agnostic. We evaluated our method against standard priority dispatching rules based on data that reflect a realistic scenario, designed on the basis of a practical case study at the Dassault Systèmes company. We used an industry-strength large neighborhood search based algorithm as benchmark. The results show that the proposed method outperforms the priority dispatching rules in terms of makespan, obtaining an average makespan difference with the best tested priority dispatching rules of 4.45% and 12.52%.
更多查看译文
关键词
flexible job shop scheduling,deep reinforcement learning,reinforcement learning,attention-based,sequence-dependent
AI 理解论文
溯源树
样例
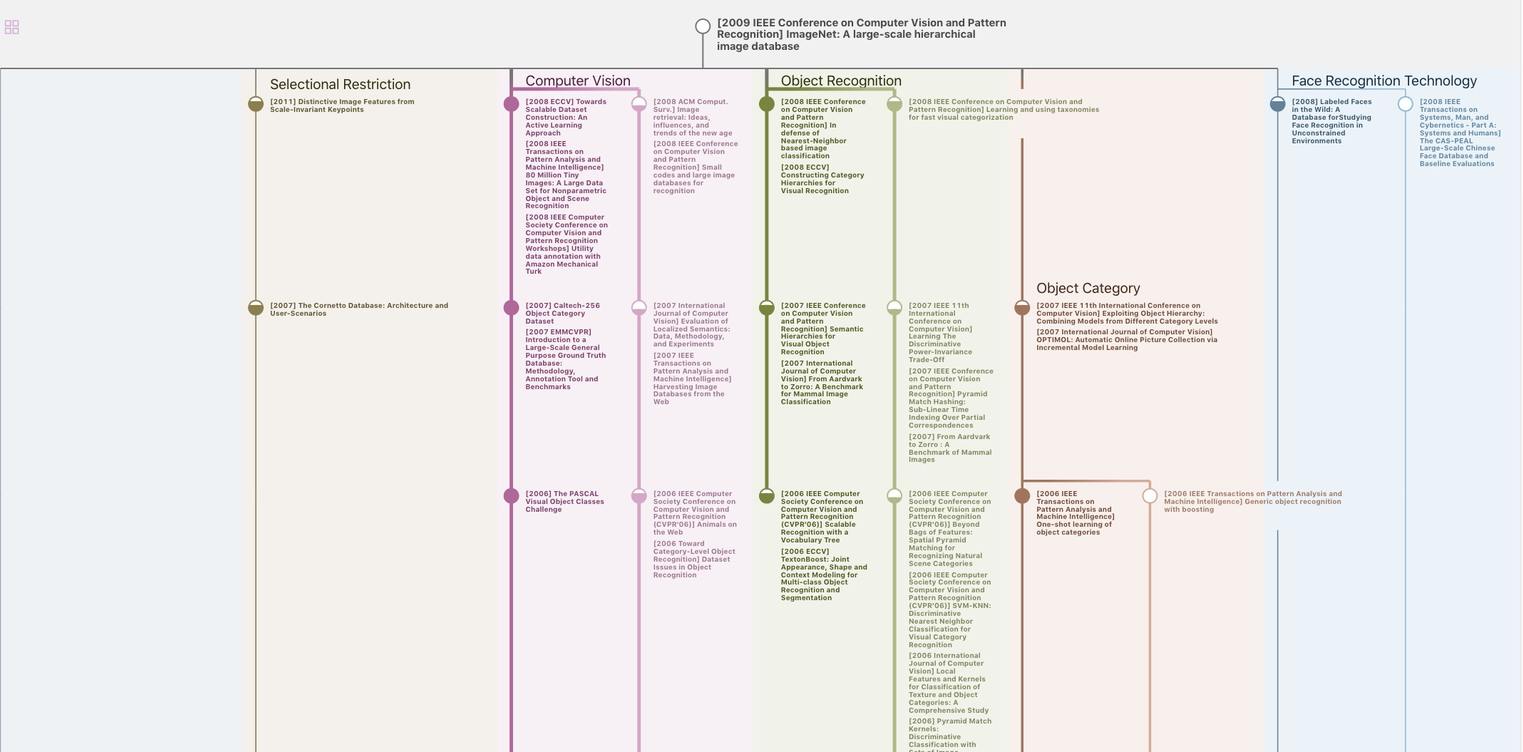
生成溯源树,研究论文发展脉络
Chat Paper
正在生成论文摘要