Registration-Propagated Liver Tumor Segmentation for Non-enhanced CT-Based Interventions.
Image and Graphics : 12th International Conference, ICIG 2023, Nanjing, China, September 22–24, 2023, Proceedings, Part IV(2023)
摘要
Intra-operative non-enhanced computed tomography (CT) has grown as a prominent tool in interventional surgeries for liver tumor by offering a real-time, more precise imaging guidance over its pre-operative enhanced counterpart as well as intra-operative ultrasound. Though deep learning has demonstrated promising performance in various medical image tasks, the challenges for liver tumor segmentation in the context of intra-operative non-enhanced CT remain due to the scarcity of accurate pixel-level-labeled tumor data and low visibility of tumor without contrast enhancement. In this paper, based on a pre-assumption of consistent relative spatial relation between liver and liver tumor at pre- and intra-surgical stages, we propose a two-step (segmentation - registration) framework to propagate liver tumor segmentation mask from pre-operative enhanced CT to corresponding intra-operative non-enhanced CT via nonrigid registration. A M ulti- s cale B ridged s patial attention block embedded in the popular “U-shaped” network architecture termed MsBs-Unet is introduced to better accommodate variations of tumor during segmentation. We show that MsBs-Unet uniformly achieves superior performances against other baselines on liver and liver tumor segmentation task in terms of Dice score and HD95 metrics on our in-house data containing a cohort of intra-operative non-enhanced CT scans and Medical Segmentation Decathlon task03 liver tumor dataset respectively. Final registration results were evaluated via tumor landmarks matching on 20 in-house intra-subject paired CT scans for both intensity and feature-based methods. Specifically for the latter, mean target registration error is 3.3 mm, and the overall intra-operative process takes around 48 s per case. The resulting tumor segmentation show gratifying promise of applying our framework for non-enhanced CT-based liver intervention.
更多查看译文
关键词
liver,tumor,registration-propagated,non-enhanced,ct-based
AI 理解论文
溯源树
样例
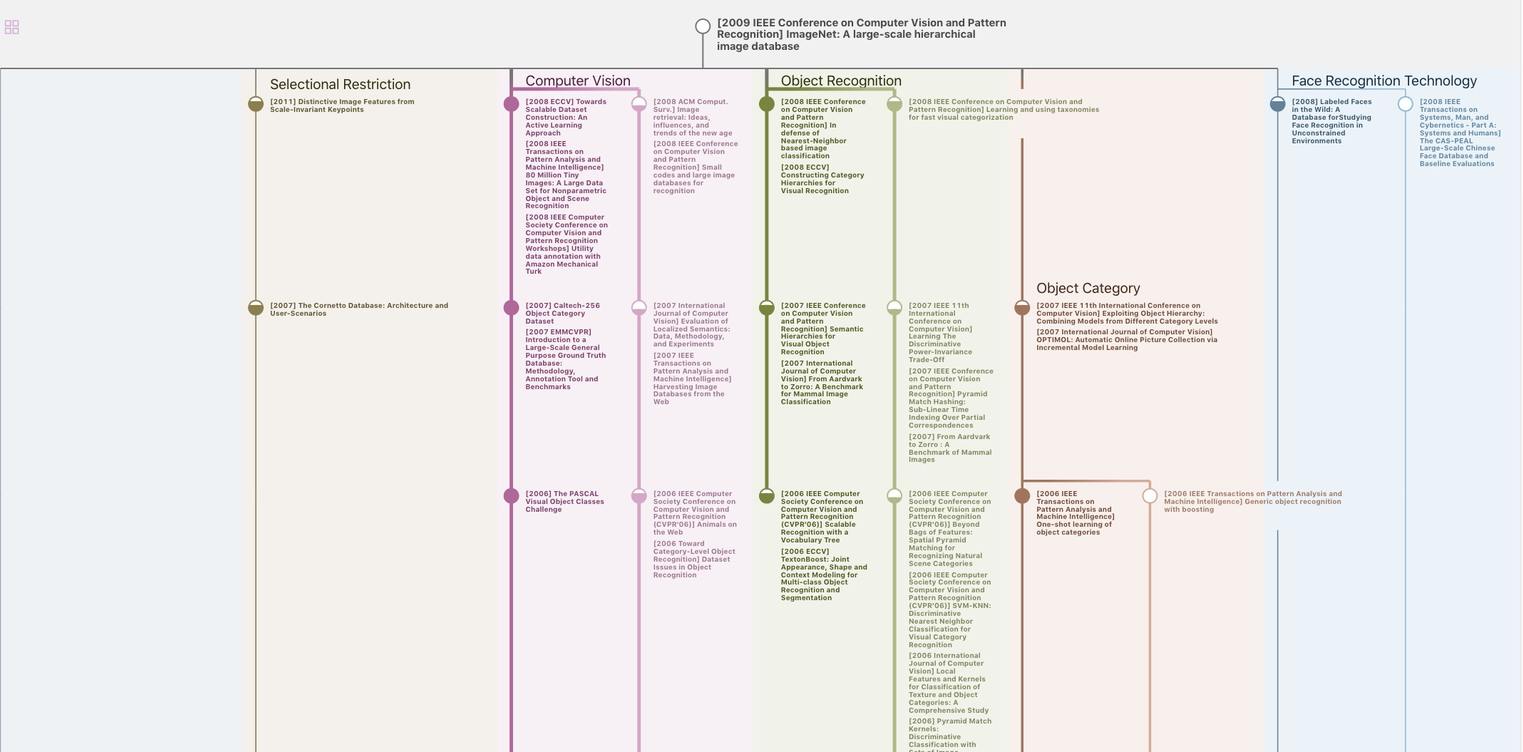
生成溯源树,研究论文发展脉络
Chat Paper
正在生成论文摘要