Residual Cascade CNN for Detection of Spatially Relevant Objects in Agriculture: The Grape-Stem Paradigm
COMPUTER VISION SYSTEMS, ICVS 2023(2023)
摘要
Computer vision is becoming increasingly important in agriculture, as it can provide important insights and lead to better informed decisions and reduce costs. However, working on the agriculture domain introduces important challenges, such as adverse conditions, small structures and lack of large datasets, hindering its wide adoption on multiple cases. This work presents an approach to improve the performance of detecting challenging small objects, by exploiting their spatial structure under the hypothesis that they are located close to larger objects, which we define as anchor. This is achieved by providing feature maps from the detections of the anchor class to the network responsible for detecting the secondary class. Thus, the secondary class object detection is formulated as a residual problem on top of the anchor class detection, benefiting from an activation bias close to the anchor object spatial locations. Experiments on the grape-stem and capsicum-peduncle cases, demonstrate increased performance against more computationally expensive baselines, resulting in improved metrics at 37% of baseline FLOPs.
更多查看译文
关键词
instance segmentation,CNN,agriculture,grape,stem
AI 理解论文
溯源树
样例
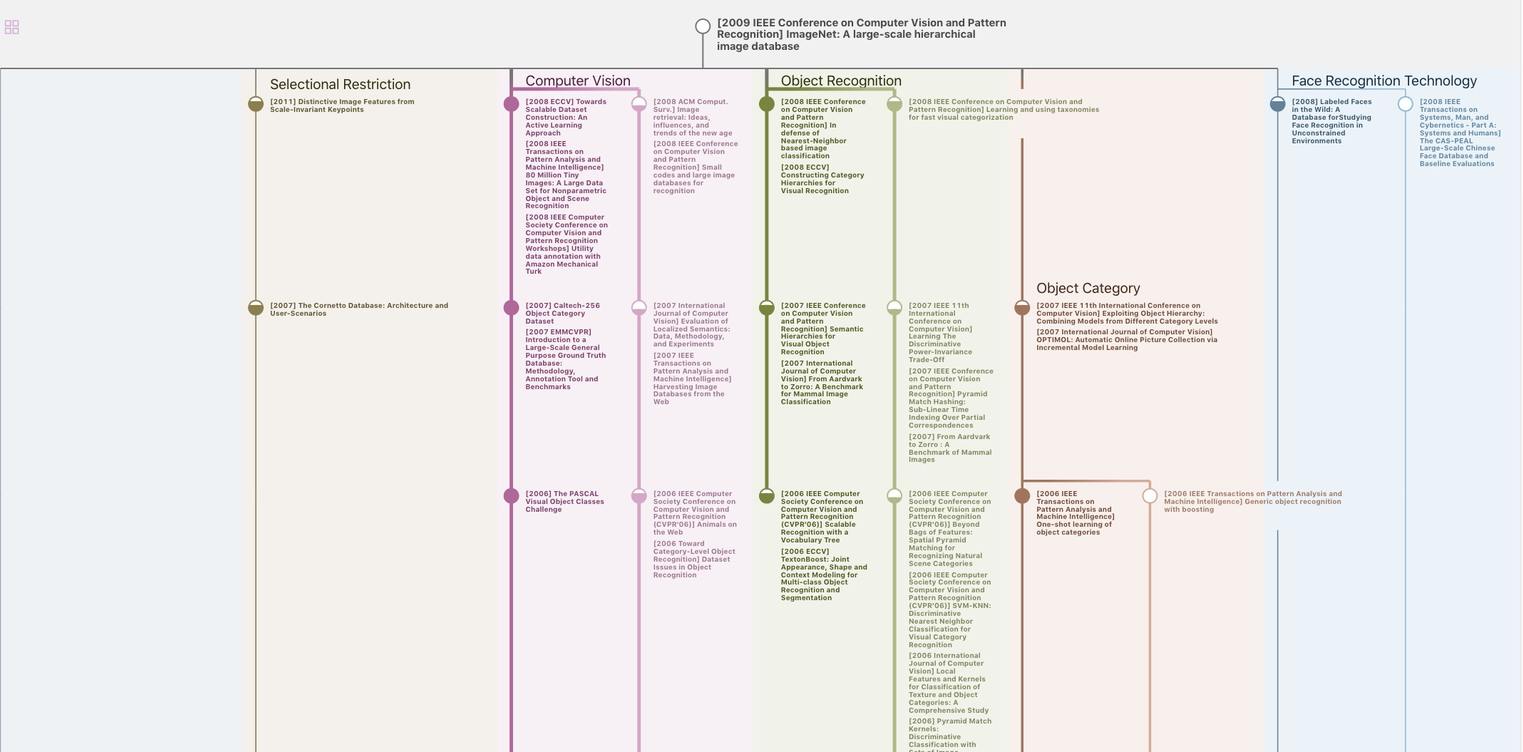
生成溯源树,研究论文发展脉络
Chat Paper
正在生成论文摘要