Temporal Attention Signatures for Interpretable Time-Series Prediction
ARTIFICIAL NEURAL NETWORKS AND MACHINE LEARNING, ICANN 2023, PT VI(2023)
摘要
Deep neural networks have become a staple in time-series prediction due to their remarkable accuracy. However, their internal workings often remain elusive. Significant advancements have been made in the interpretability of these networks, with attention mechanisms and feature maps being notably effective for image classification by highlighting the crucial data points. While human observers can readily confirm the significance of features in image classification, the interpretability of time-series data and its modeling remains challenging. To address this, we put forth an innovative approach that unifies temporal attention and visualization as a blend of recurrent neural networks, self-attention, and general attention. This synergy results in the generation of temporal attention signatures, akin to image attention heat maps. Temporal attention not only enhances prediction accuracy beyond that of recurrent networks alone but also demonstrates that varying label classes yield distinct attention signatures. This observation indicates that neural networks focus on different sections of time-series sequences contingent on the prediction target. We conclude with a discussion on the practical implications of this novel approach, including its applicability to model interpretation, sequence length selection, and model validation. This leads to more accurate, robust, and interpretable models, instilling greater confidence in their results.
更多查看译文
关键词
Neural Networks,Deep Learning,Attention Mechanisms,Time-Series,Model Interpretability
AI 理解论文
溯源树
样例
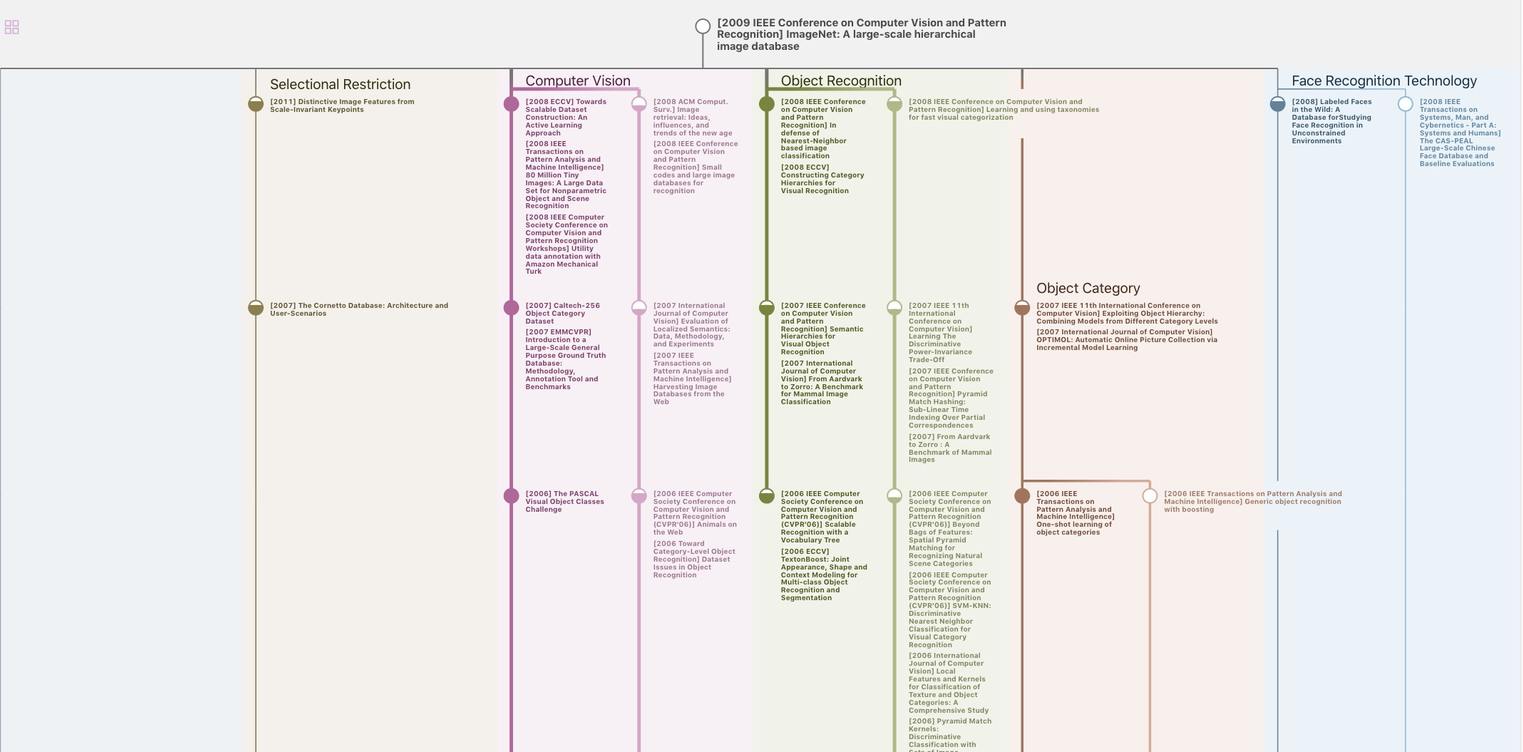
生成溯源树,研究论文发展脉络
Chat Paper
正在生成论文摘要