A Novel Encoder and Label Assignment for Instance Segmentation
ARTIFICIAL NEURAL NETWORKS AND MACHINE LEARNING, ICANN 2023, PT VI(2023)
摘要
SparseInst, a recent lightweight instance segmentation network, achieves better balance between efficiency and precision. However, the information contained in the single-layer features output by the encoder is not rich enough and the label assignment strategy leads to imbalance between positive and negative samples. In order to further improve the instance segmentation performance, we propose LAIS network including a novel feature encoding module and a Multi-Step Hungarian matching strategy (MSH). By combining multi-scale feature extraction and inter-layer information fusion, the encoder outputs features with more detailed and comprehensive information. By performing multiple rounds of one-to-one Hungarian matching, MSH eliminates the imbalance and duplication during the sample allocation. Experiments show that LAIS is more accurate than SparseInst without significantly increasing parameters and GFLOPs. In particular, LAIS reached 33.8% AP on the COCO val, 1.0% higher than SparseInst when using the same ResNet-50 Backbone.
更多查看译文
关键词
Instance Segmentation,Single-Output,Feature Extraction,Label Assignment
AI 理解论文
溯源树
样例
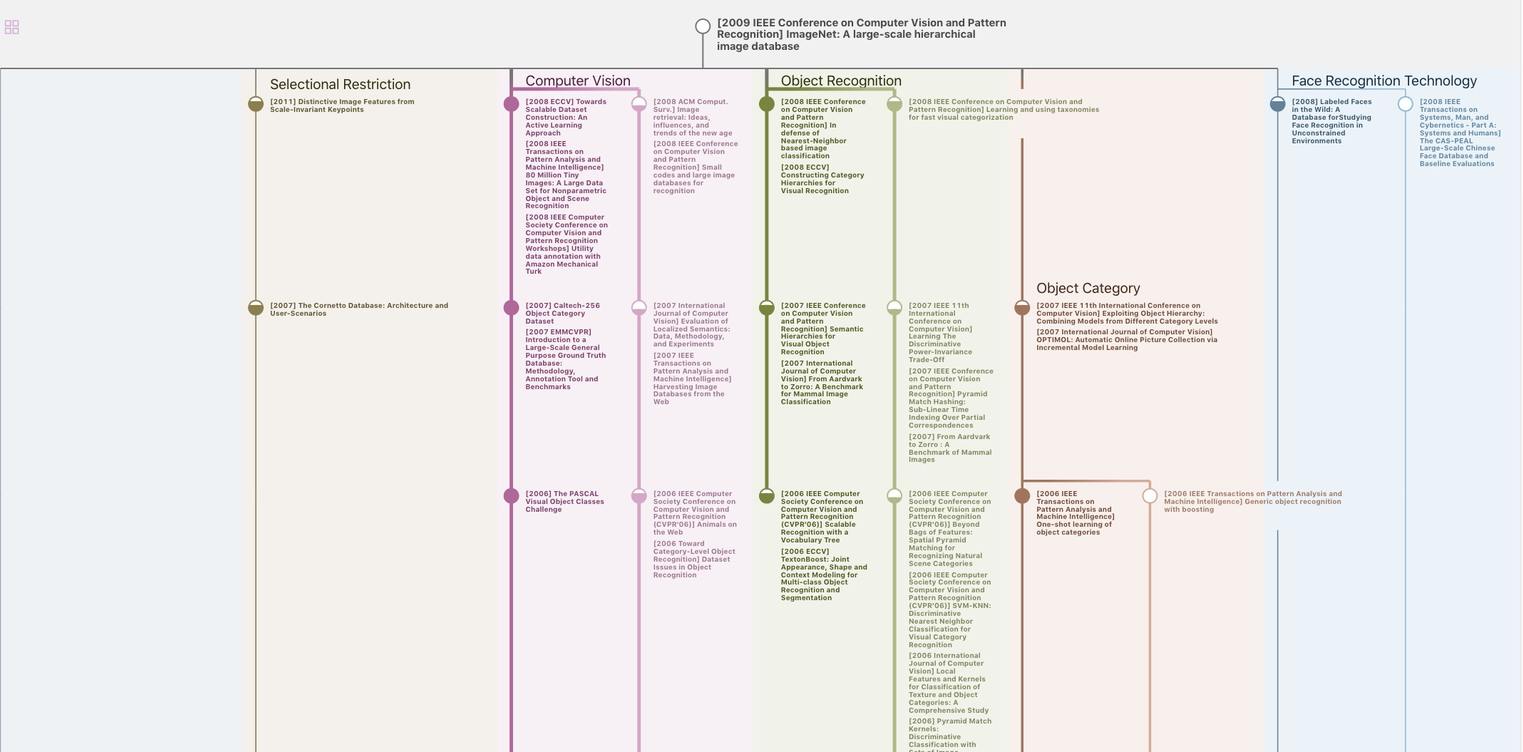
生成溯源树,研究论文发展脉络
Chat Paper
正在生成论文摘要