Investigating the Impact of Image Quality on Endoscopic AI Model Performance
APPLICATIONS OF MEDICAL ARTIFICIAL INTELLIGENCE, AMAI 2023(2024)
摘要
Virtually all endoscopic AI models are developed with clean, high-quality imagery from expert centers, however, the clinical data quality is much more heterogeneous. Endoscopic image quality can degrade by e.g. poor lighting, motion blur, and image compression. This disparity between training, validation data, and real-world clinical practice can have a substantial impact on the performance of deep neural networks (DNNs), potentially resulting in clinically unreliable models. To address this issue and develop more reliable models for automated cancer detection, this study focuses on identifying the limitations of current DNNs. Specifically, we evaluate the performance of these models under clinically relevant and realistic image corruptions, as well as on a manually selected dataset that includes images with lower subjective quality. Our findings highlight the importance of understanding the impact of a decrease in image quality and the need to include robustness evaluation for DNNs used in endoscopy.
更多查看译文
关键词
DNN,Image degradation,Endoscopy,Robustness
AI 理解论文
溯源树
样例
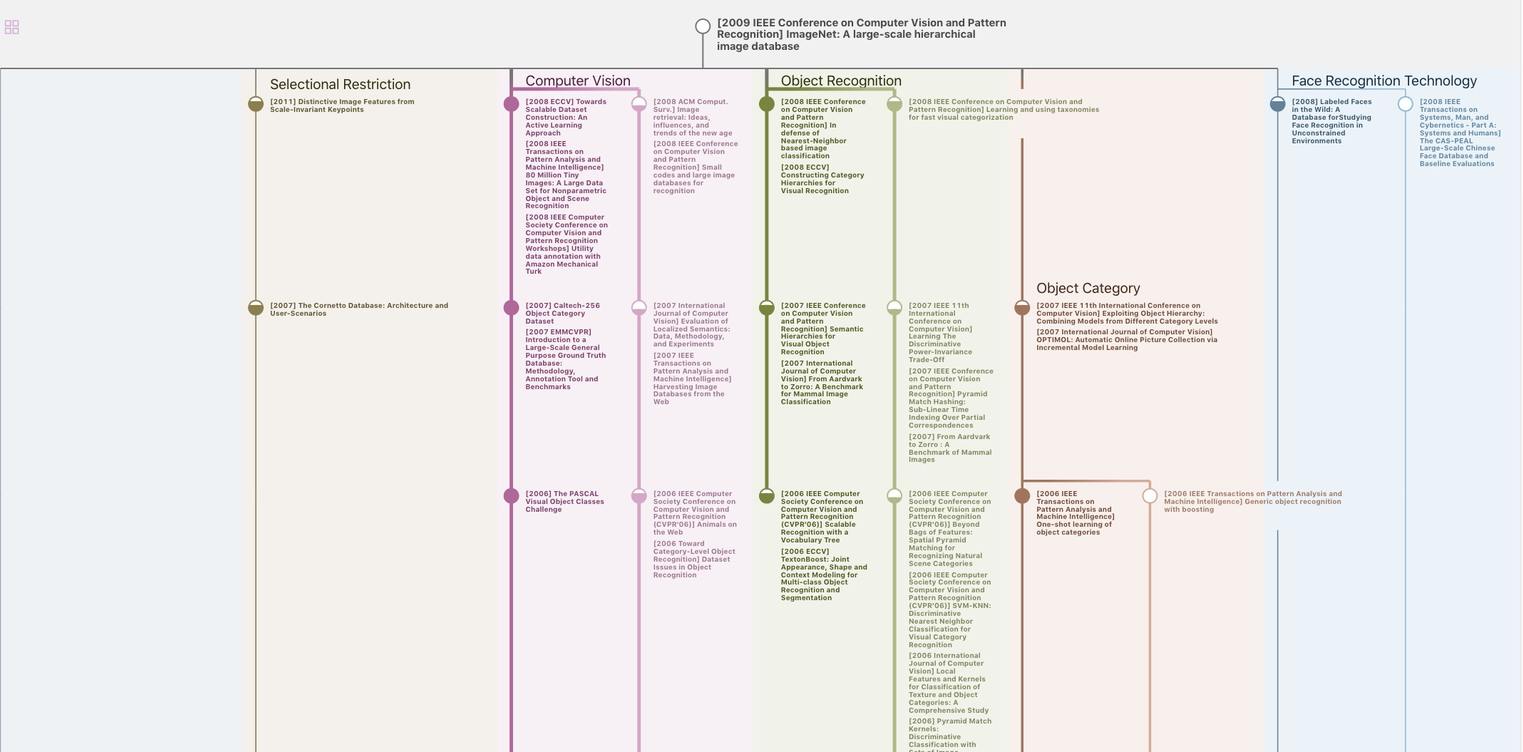
生成溯源树,研究论文发展脉络
Chat Paper
正在生成论文摘要