Comparing Transformer-Based Machine Translation Models for Low-Resource Languages of Colombia and Mexico
ADVANCES IN SOFT COMPUTING, MICAI 2023, PT II(2024)
摘要
This paper offers a comparative analysis of two state-of-the-art machine translation models for Spanish to Indigenous languages of Colombia and Mexico, with the aim of investigating their effectiveness and limitations under low-resource conditions. Our methodology involved aligning verse pairs text using the Bible for twelve Indigenous languages and constructing parallel datasets for evaluation using BLEU and ROUGE metrics. The results demonstrate that transformer-based models can deliver competitive performance in translating from Spanish to Indigenous languages with minimal configuration. In particular, we found the Opus-based model obtained the best performance in 11 of the languages in the test set but, the Fairseq model performs competitively in scenarios where training data is more scarce. Additionally, we provide a comprehensive analysis of the findings, including insights into the strengths and limitations of the models. Finally, we suggest potential directions for future research in low-resource language translation, specifically in the context of Latin American indigenous languages.
更多查看译文
关键词
Low-resource languages,Machine translation,Indigenous languages
AI 理解论文
溯源树
样例
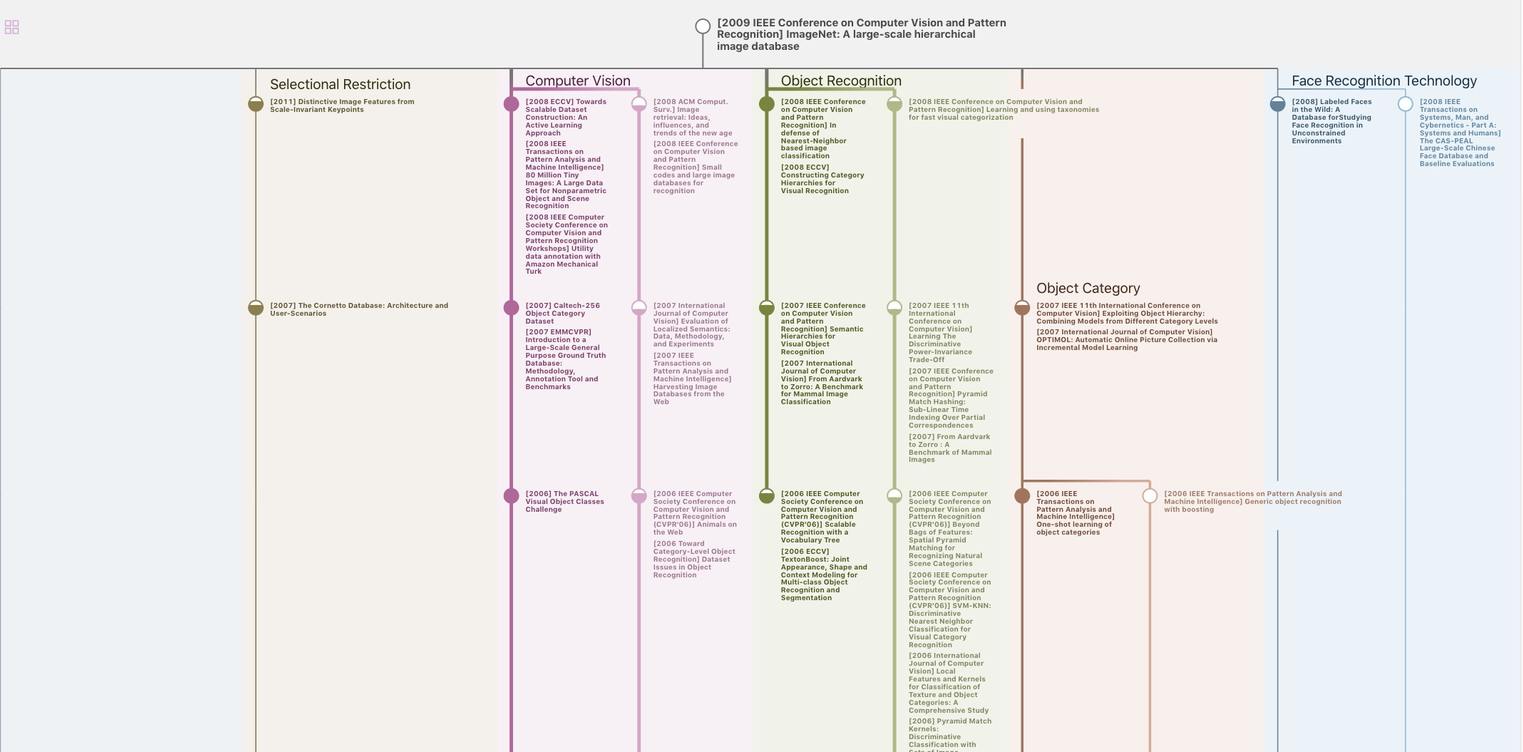
生成溯源树,研究论文发展脉络
Chat Paper
正在生成论文摘要