Empirical Exploration of Open-Source Issues for Predicting Privacy Compliance.
Advances in Conceptual Modeling: ER 2023 Workshops, CMLS, CMOMM4FAIR, EmpER, JUSMOD, OntoCom, QUAMES, and SmartFood, Lisbon, Portugal, November 6–9, 2023, Proceedings(2023)
摘要
In the last decade, privacy has gained a significant interest in software and information systems engineering mainly due to the emergence of privacy regulations, including the General Data Protection Regulation (GDPR). However, checking privacy compliance is challenging and depends on many factors, such as the programming language and the software architecture, as well as the underlying regulation. In this exploratory research, we aim to study whether positive discussions on privacy-related issues in Open-Source Software (OSS) environments can predict privacy compliance of the software. Such predictions are beneficial in different scenarios, including in software reuse. Our main contribution will lie in conceptually modeling and understanding the relations between privacy compliance and positive discussions of privacy-related OSS issues. The research comprises three parts: (1) identifying privacy-related issues using supervised machine learning techniques; (2) improving the identification of privacy-related issues utilizing ontologies; and (3) identifying the sentiment of privacy-related issues and analyzing relations to privacy compliance. This paper describes the design and results of part 1, as well as the design of parts 2 and 3.
更多查看译文
关键词
privacy compliance,open-source
AI 理解论文
溯源树
样例
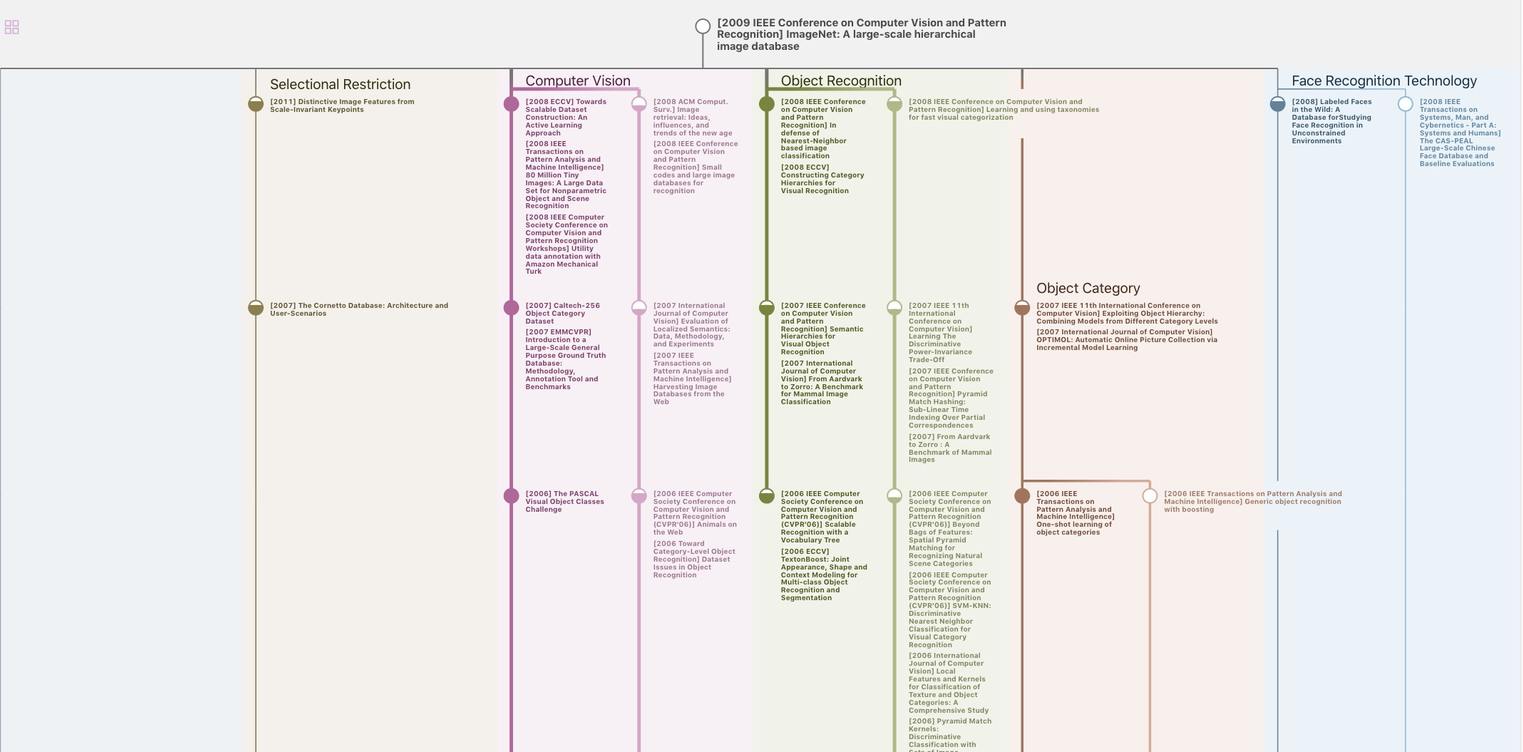
生成溯源树,研究论文发展脉络
Chat Paper
正在生成论文摘要