Comparative Analysis of ARIMA, LSTM, and XGBoost for Very Short-Term Photovoltaic Forecasting
2023 15TH SEMINAR ON POWER ELECTRONICS AND CONTROL, SEPOC(2023)
摘要
Photovoltaic (PV) forecasting is required to optimize grid management and boost renewable energy integration. This paper presents a comparative analysis of three forecast models, autoregressive integrated moving average (ARIMA), eXtreme Gradient Boosting (XGBoost), and long short-term memory (LSTM) for very short-term PV forecasting applied to two real datasets. The main objective is to evaluate the complexity, performance, and accuracy of these algorithms in predicting very short-term PV power based only on the historical PV time series data. Furthermore, an additional study incorporates weather and calendar features for the LSTM model to examine their impact on forecasting quality. The performance of the models is evaluated in terms of root mean squared error (RMSE) and mean absolute error (MAE). Feature selection and hyperparameter optimization are also considered. The results highlight the strengths and limitations of each forecasting model in accurately capturing very short-term PV variations and show that XGBoost had the most accurate forecasting metrics for both datasets for univariate models. The research outcomes provide valuable insights for energy system operators, grid managers, and renewable energy stakeholders to make informed decisions regarding grid stability and efficient integration of PV generation.
更多查看译文
关键词
ARIMA,LSTM,photovoltaic power,very-shortterm forecasting,XGBoost
AI 理解论文
溯源树
样例
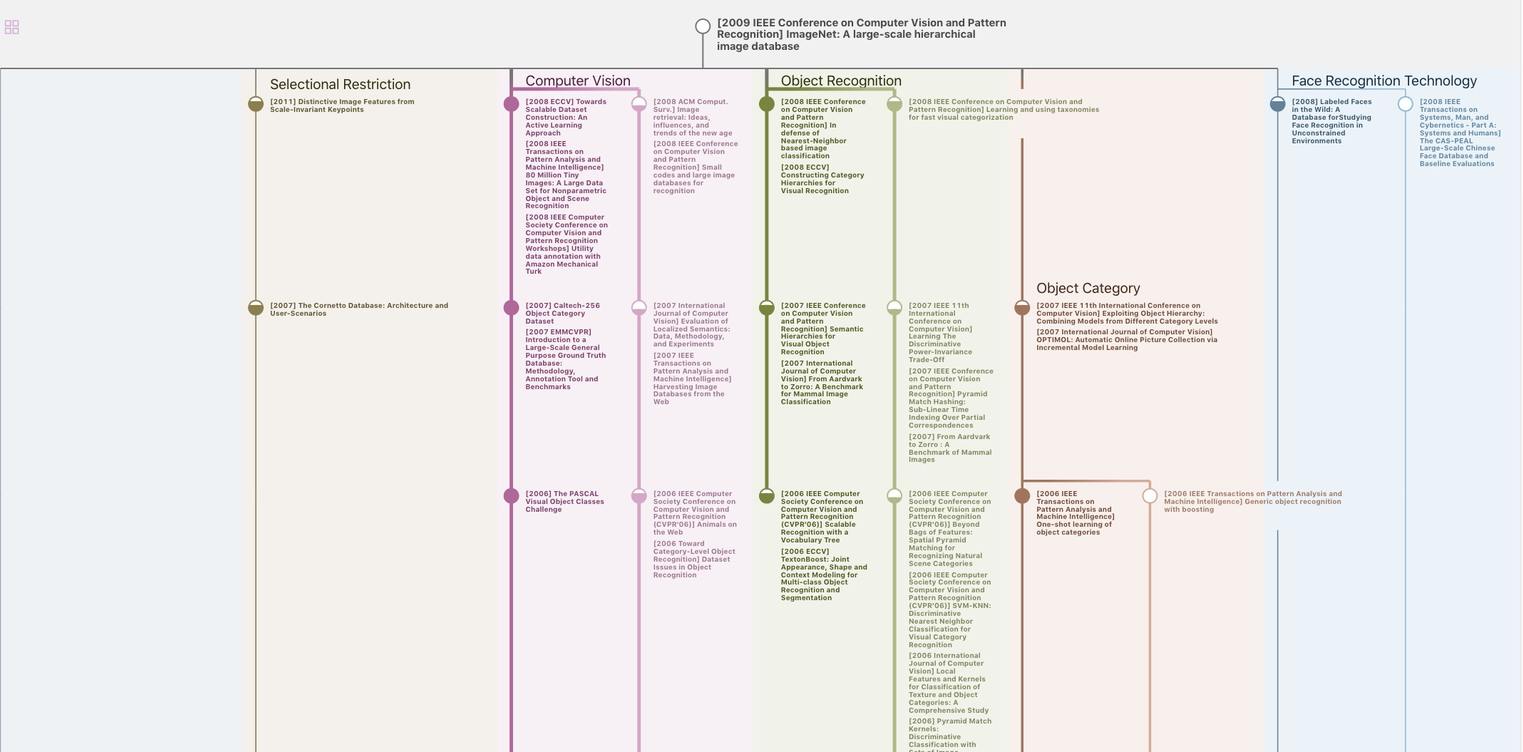
生成溯源树,研究论文发展脉络
Chat Paper
正在生成论文摘要