Extended km-Anonymity for Randomization Applied to Binary Data
2023 20th Annual International Conference on Privacy, Security and Trust (PST)(2023)
摘要
Various models for evaluating anonymity have been proposed so far. Among them, k-anonymity is widely known as a typical anonymity measure, which guarantees that at least k individuals in a database have the same values. However, it is difficult to create highly useful anonymized data satisfying k-anonymity for high-dimensional data because of the curse of dimensionality. To overcome the problem, several approaches relaxing k-anonymity have been proposed, such as k(m)-anonymity and sigma-k(m)-anonymity. Unfortunately, they can only evaluate deterministic anonymization methods. We propose Pk(m)-anonymity, a variant of k(m)-anonymity, and prove that k(m)-anonymity and Pk(m)-anonymity are equivalent in a deterministic privacy mechanism. This suggests that our Pk(m)-anonymity is an extension of k(m) anonymity. Also, we propose a k(m)-anonymization method for binary data, unlike the previous approaches for non-binary data. The success probability and utility of the proposed method are examined with the number of attributes as a parameter. Our experiments show that the "curse of dimensionality" does not occur up to a dimensionality of 45 and that usefulness does not deteriorate in the range of dimensionality from 10 to 40.
更多查看译文
关键词
k(m)-anonymity,Pk-anonymity,privacy mechanism,binary data
AI 理解论文
溯源树
样例
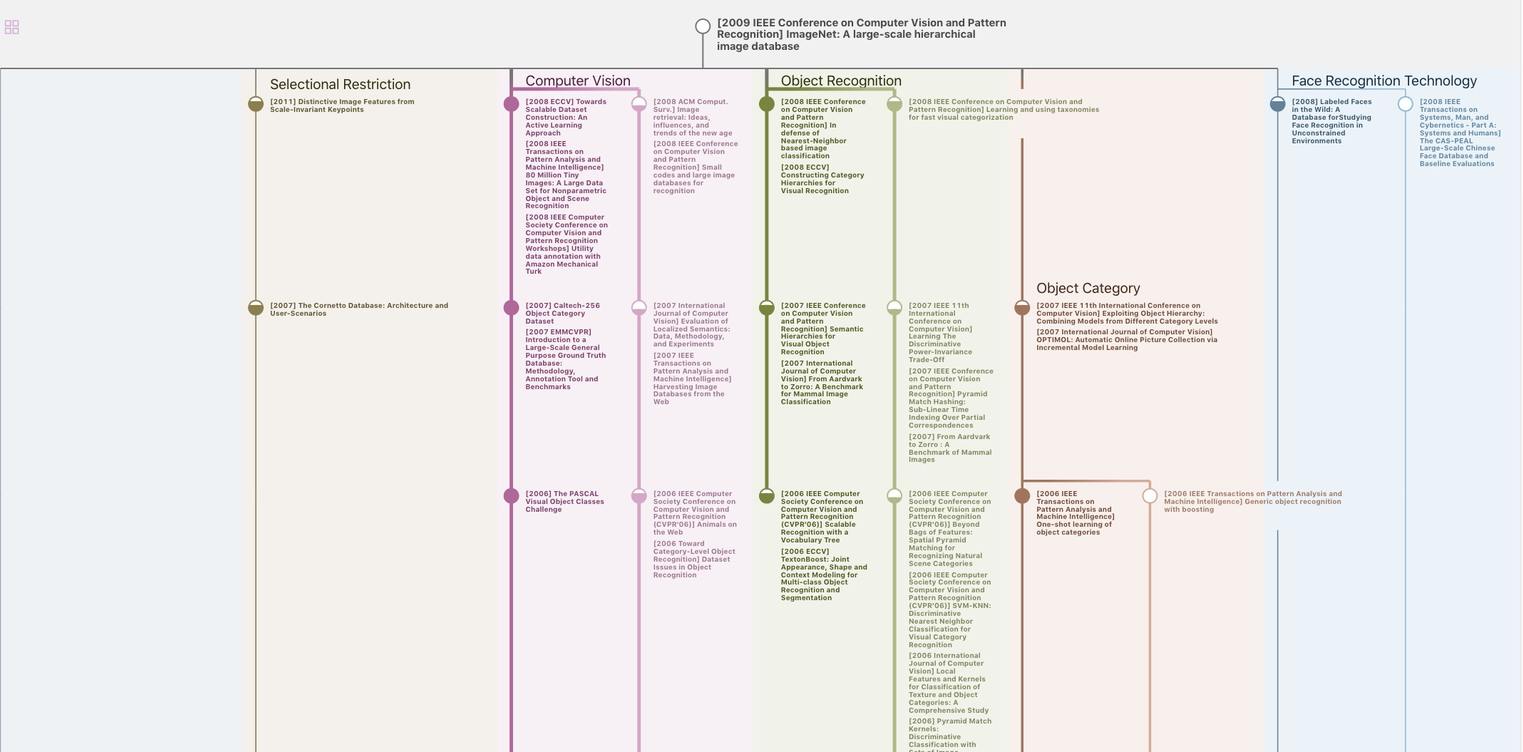
生成溯源树,研究论文发展脉络
Chat Paper
正在生成论文摘要