An Instance-based Transfer Learning Approach, Applied to Intrusion Detection
2023 20TH ANNUAL INTERNATIONAL CONFERENCE ON PRIVACY, SECURITY AND TRUST, PST(2023)
摘要
To detect malicious activities in the network, Intrusion Detection Systems (IDS) are deployed. One way to build IDS is through Machine Learning (ML) techniques. Using ML techniques for building IDS models has a few shortcomings. The performance of these models is affected when new attacks emerge, such as zero-day attacks. That is because the traditional techniques assume that training and testing data come from the same distribution, therefore, when new attacks emerge, the underlying distribution changes also and affects the performance of the model. Also, the attack samples of new attacks may be scarce. In this paper, we present a solution to train an IDS model, where scarce data is available, using an instance-based Transfer Learning (TL) approach. This approach allows for increasing the sample size in the Target Domain by using similar instances from a related Source Domain. We conducted our experiments using the UNSW-NB15 dataset and the obtained results are appealing. Indeed, we obtained 92.5%, 88.4%, 86.5%, and 86.8%, using the widely used performance metrics Accuracy, Recall, Precision and F1-Score, respectively. These results are obtained even though the distribution difference between the Source and Target Domains is significantly higher, as measured with the Maximum Mean Discrepancy (MMD) metric.
更多查看译文
关键词
Intrusion detection system,transfer learning,machine learning,instance-based,TrAdaBoost,maximum mean discrepancy
AI 理解论文
溯源树
样例
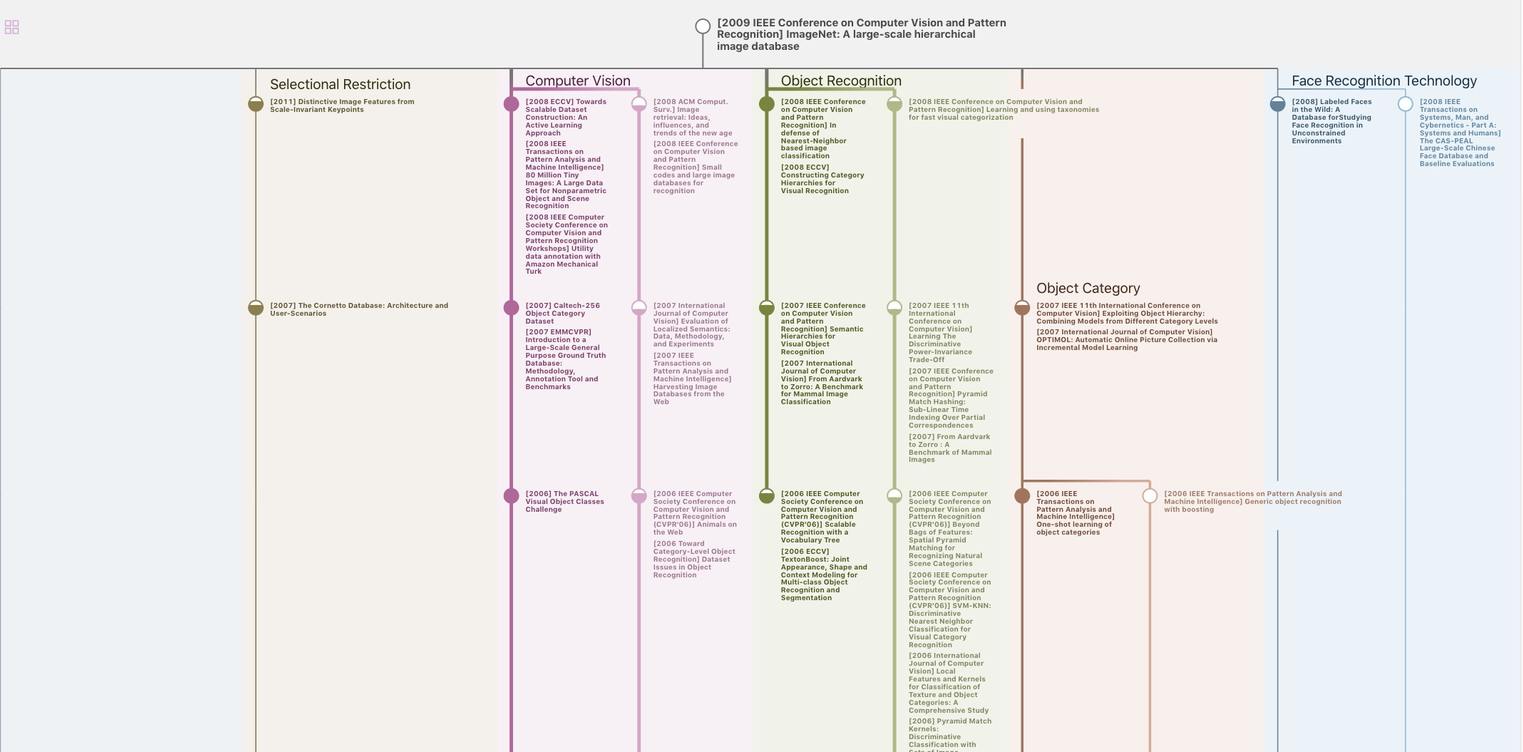
生成溯源树,研究论文发展脉络
Chat Paper
正在生成论文摘要