A Hybrid Artificial Intelligence Approach for Early Stroke Prediction
2023 5th International Conference on Pattern Analysis and Intelligent Systems (PAIS)(2023)
摘要
This research aims to explore the application of Machine Learning (ML) models, specifically ensemble methods, in conjunction with Genetic Algorithms (GA) for the early prediction of strokes using real-world data from Kaggle. The primary focus is on enhancing the performance of the stacking ensemble method.Stacking leverages the strengths of various ML models while mitigating their weaknesses, creating a meta-model that aggregates predictions from base models. We conducted extensive testing involving multiple meta-models and compared their performance. The Multi-Layer Perceptron (MLP) meta-model, with tuned parameters, outperformed others. It was trained on selected dataset features and data from base models selected via GA. Subsequently, we assessed its performance using an independent test set. We employed various metrics for evaluation, including accuracy, F1 score, recall, precision, AUC-ROC, and Matthews Correlation Coefficient (MCC). Our assessment also encompassed measuring time complexity by calculating execution time and prediction time. The results underscore the superior performance of this meta-model across all metrics, including accuracy (99.69%), F1-score (99.68%), recall (99.78%), precision (99.57%), AUC-ROC (99.69%), MCC (99.38%), execution time (2.20 minutes), and prediction time (0.0020 seconds).
更多查看译文
关键词
stroke,machine learning,hybrid model,ensemble methods,stacking,multi-Layer perceptron,genetic algorithm,attribute selection,early prediction
AI 理解论文
溯源树
样例
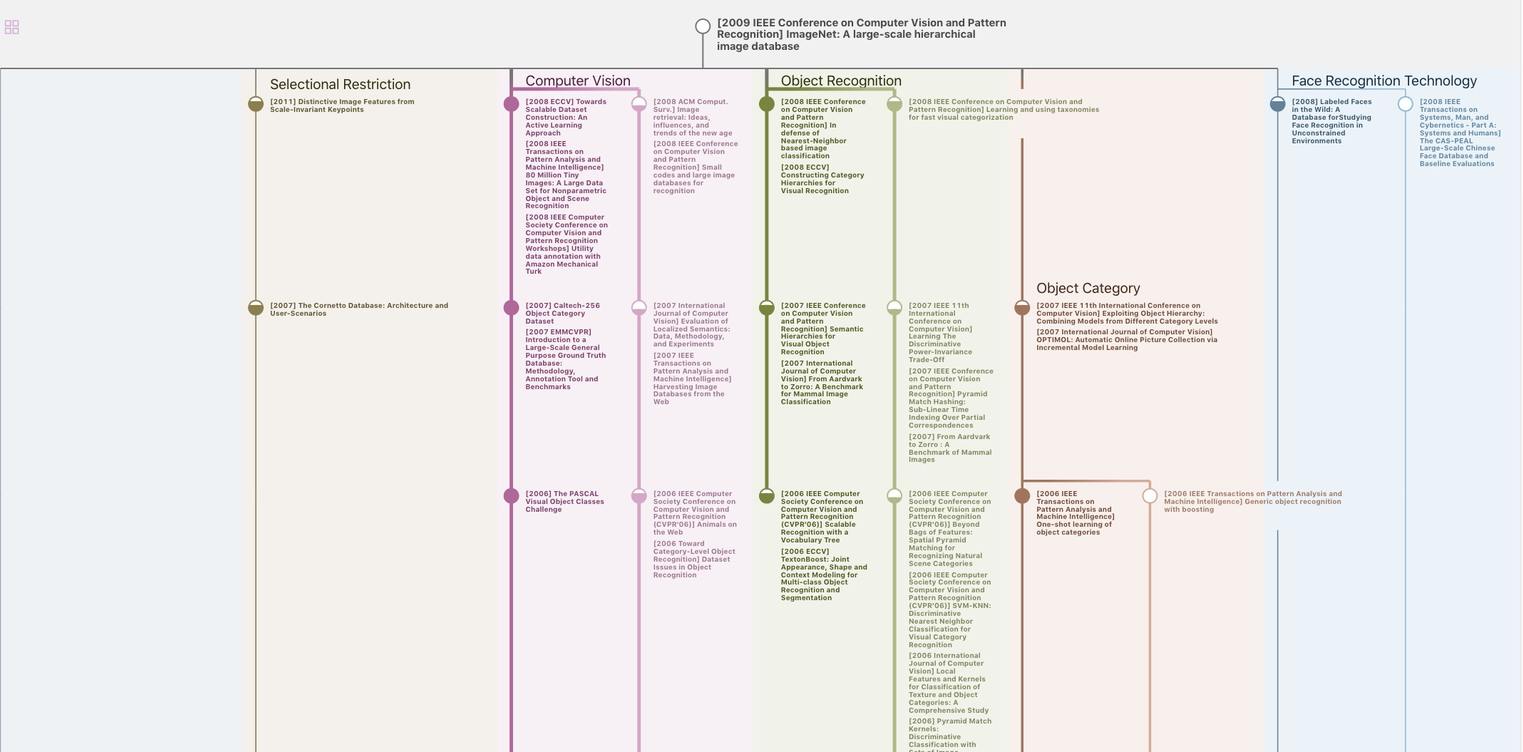
生成溯源树,研究论文发展脉络
Chat Paper
正在生成论文摘要