An Efficient and Accurate Compression Ratio Estimation Model for SZx
2023 IEEE International Conference on Cluster Computing Workshops (CLUSTER Workshops)(2023)
摘要
Modern large-scale HPC applications generate enormous volumes of data for subsequent storage or transfer. Error-controlled lossy compression effectively reduces data sizes and preserves data fidelity based on user-defined error bounds, but compression ratios are unknown until after compression. Many use cases, however, require knowledge of compression ratios a priori to pre-allocate memory for the compressed data at runtime and avoid simulation crashes caused by lack of storage space. We propose Surrogate-based Error-controlled Lossy Compression Ratio Estimation Framework (SECRE), which estimates the true compression ratio via data sampling and a lightweight compression surrogate. Results for SZx on 4 real-world scientific datasets show an extremely low estimation error (e.g., similar to 1% estimation errors for SZx) and low execution overhead (e.g., similar to 2% estimation cost for SZx).
更多查看译文
关键词
error-controlled lossy compression,scientific datasets,compression ratio estimation,sampling
AI 理解论文
溯源树
样例
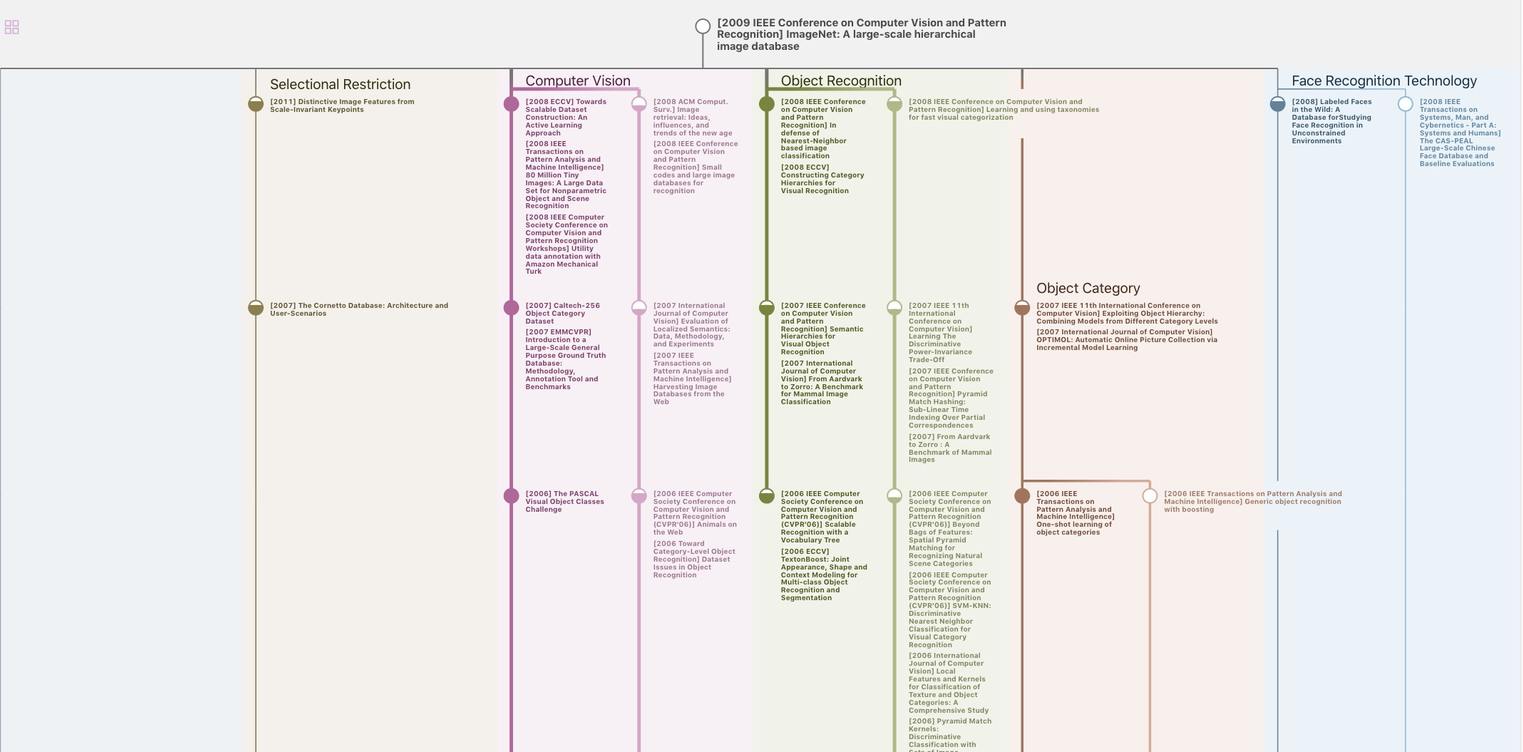
生成溯源树,研究论文发展脉络
Chat Paper
正在生成论文摘要