Full automation of point defect detection in transition metal dichalcogenides through a dual mode deep learning algorithm
MATERIALS HORIZONS(2024)
摘要
Point defects often appear in two-dimensional (2D) materials and are mostly correlated with physical phenomena. The direct visualisation of point defects, followed by statistical inspection, is the most promising way to harness structure-modulated 2D materials. Here, we introduce a deep learning-based platform to identify the point defects in 2H-MoTe2: synergy of unit cell detection and defect classification. These processes demonstrate that segmenting the detected hexagonal cell into two unit cells elaborately cropped the unit cells: further separating a unit cell input into the Te2/Mo column part remarkably increased the defect classification accuracies. The concentrations of identified point defects were 7.16 x 1020 cm2 of Te monovacancies, 4.38 x 1019 cm2 of Te divacancies and 1.46 x 1019 cm2 of Mo monovacancies generated during an exfoliation process for TEM sample-preparation. These revealed defects correspond to the n-type character mainly originating from Te monovacancies, statistically. Our deep learning-oriented platform combined with atomic structural imaging provides the most intuitive and precise way to analyse point defects and, consequently, insight into the defect-property correlation based on deep learning in 2D materials. We advocate for the development of expertise in visualizing and identifying point defects in two-dimensional (2D) materials, a skillset intimately linked to a wide array of physical phenomena.
更多查看译文
AI 理解论文
溯源树
样例
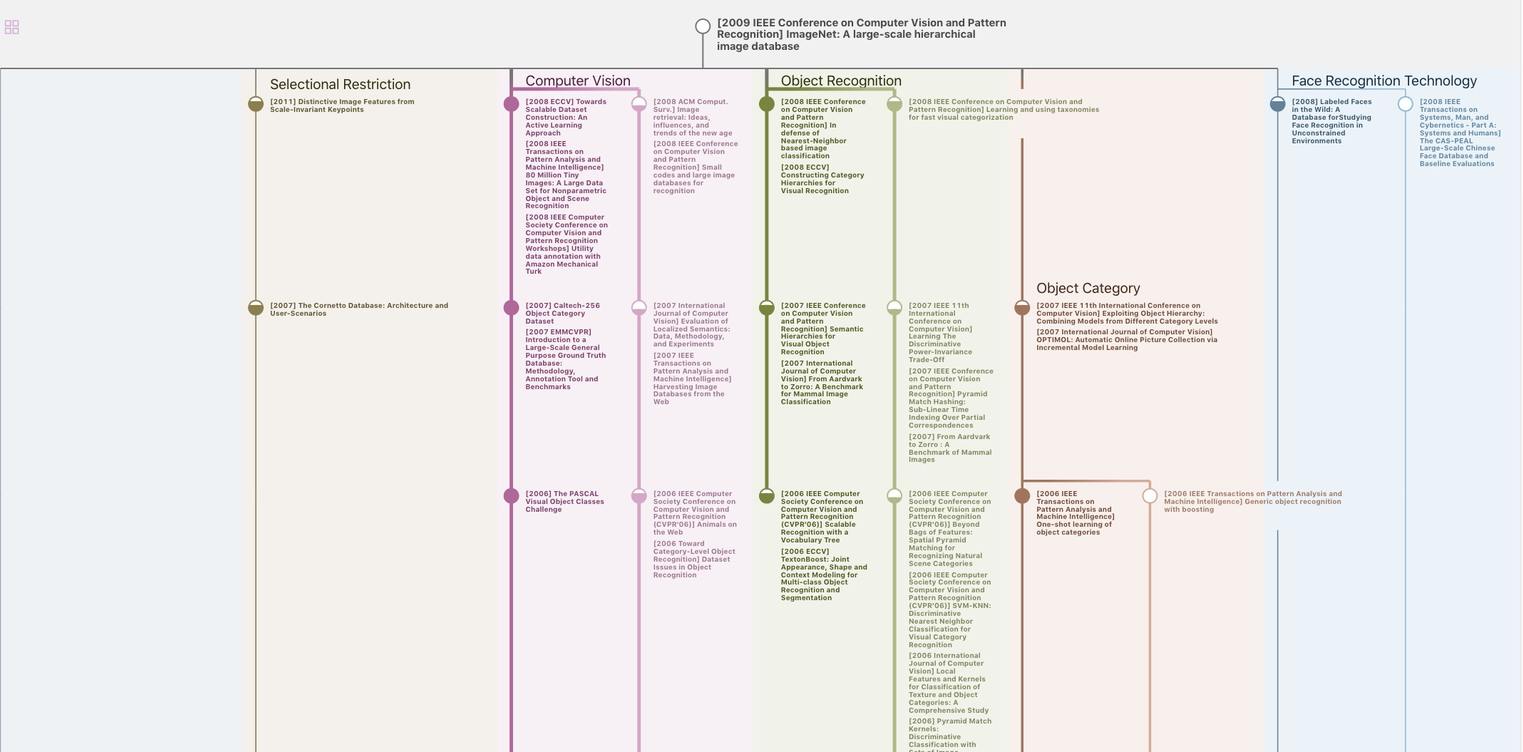
生成溯源树,研究论文发展脉络
Chat Paper
正在生成论文摘要