A deep learning LSTM-based approach for forecasting annual pollen curves: Olea and Urticaceae pollen types as a case study
COMPUTERS IN BIOLOGY AND MEDICINE(2024)
摘要
Airborne pollen can trigger allergic rhinitis and other respiratory diseases in the synthesised population, which makes it one of the most relevant biological contaminants. Therefore, implementing accurate forecast systems is a priority for public health. The current forecast models are generally useful, but they falter when long time series of data are managed. The emergence of new computational techniques such as the LSTM algorithms could constitute a significant improvement for the pollen risk assessment. In this study, several LSTM variants were applied to forecast monthly pollen integrals in Malaga (southern Spain) using meteorological variables as predictors. Olea and Urticaceae pollen types were modelled as proxies of different annual pollen curves, using data from the period 1992-2022. The aims of this study were to determine the LSTM variants with the highest accuracy when forecasting monthly pollen integrals as well as to compare their performance with the traditional pollen forecast methods. The results showed that the CNN-LSTM were the most accurate when forecasting the monthly pollen integrals for both pollen types. Moreover, the traditional forecast methods were outperformed by all the LSTM variants. These findings highlight the importance of implementing LSTM models in pollen forecasting for public health and research applications.
更多查看译文
关键词
Pollen prediction,LSTM,Random forest,Neural network
AI 理解论文
溯源树
样例
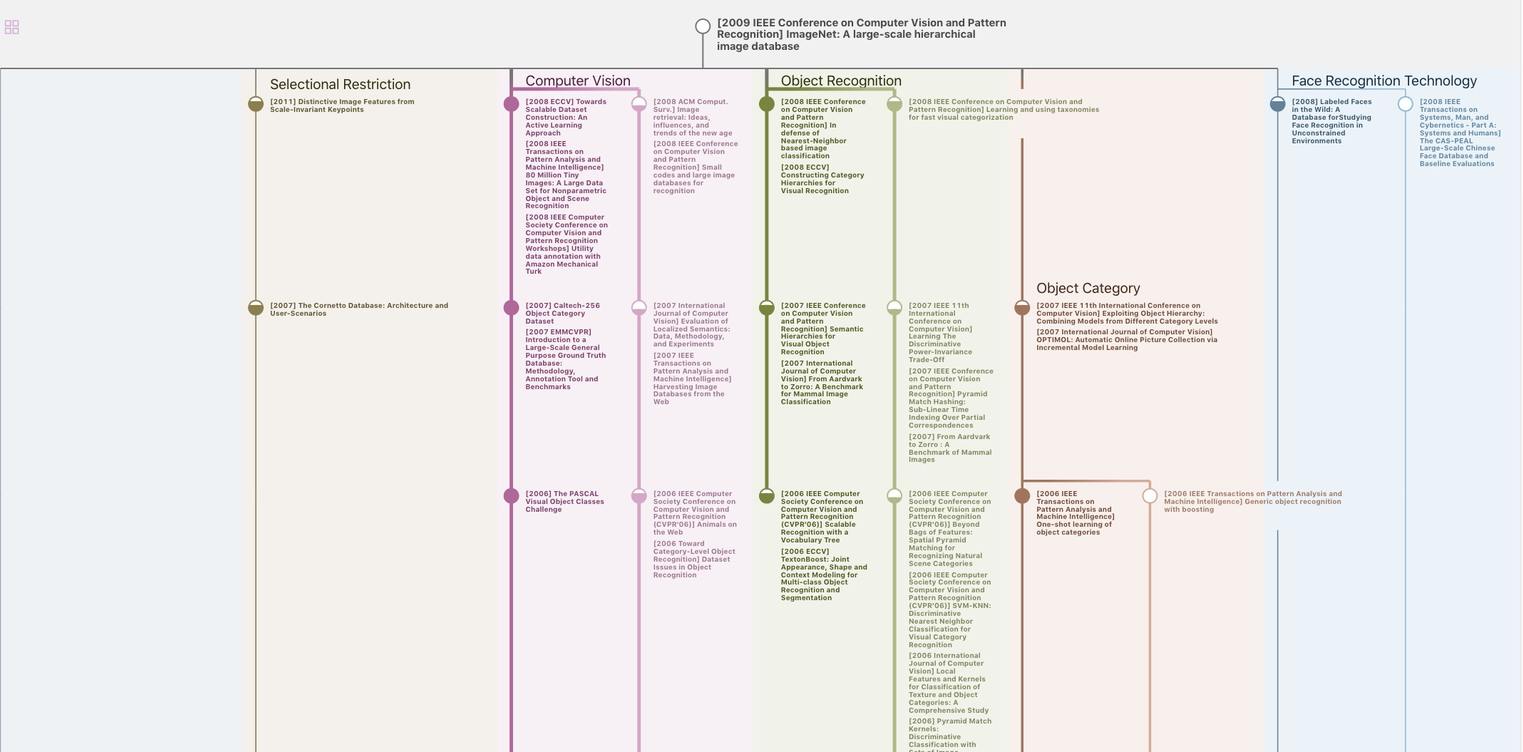
生成溯源树,研究论文发展脉络
Chat Paper
正在生成论文摘要