Stolen Risks of Models with Security Properties
PROCEEDINGS OF THE 2023 ACM SIGSAC CONFERENCE ON COMPUTER AND COMMUNICATIONS SECURITY, CCS 2023(2023)
摘要
Verifiable robust machine learning, as a new trend of ML security defense, enforces security properties (e.g., Lipschitzness, Monotonicity) on machine learning models and achieves satisfying accuracy-security trade-off. Such security properties identify a series of evasion strategies of ML security attackers and specify logical constraints on their effects on a classifier (e.g., the classifier is monotonically increasing along some feature dimensions). However, little has been done so far to understand the side effect of those security properties on the model privacy. In this paper, we aim at better understanding the privacy impacts on security properties of robust ML models. Particularly, we report the first measurement study to investigate the model stolen risks of robust models satisfying four security properties (i.e., Local-Invariance, Lipschitzness, SmallNeighborhood, and Monotonicity). Our findings bring to light the factors that influence model stealing attacks and defense performance on models trained with security properties. In addition, to train an ML model satisfying goals in accuracy, security, and privacy, we propose a novel technique, called BoundaryFuzz, which introduces a privacy property into verifiable robust training frameworks to defend against model stealing attacks on robust models. Experimental results demonstrate the defense effectiveness of BoundaryFuzz.
更多查看译文
关键词
Adversarial Machine Learning,Model Stealing Attacks and Defenses,Security Properties
AI 理解论文
溯源树
样例
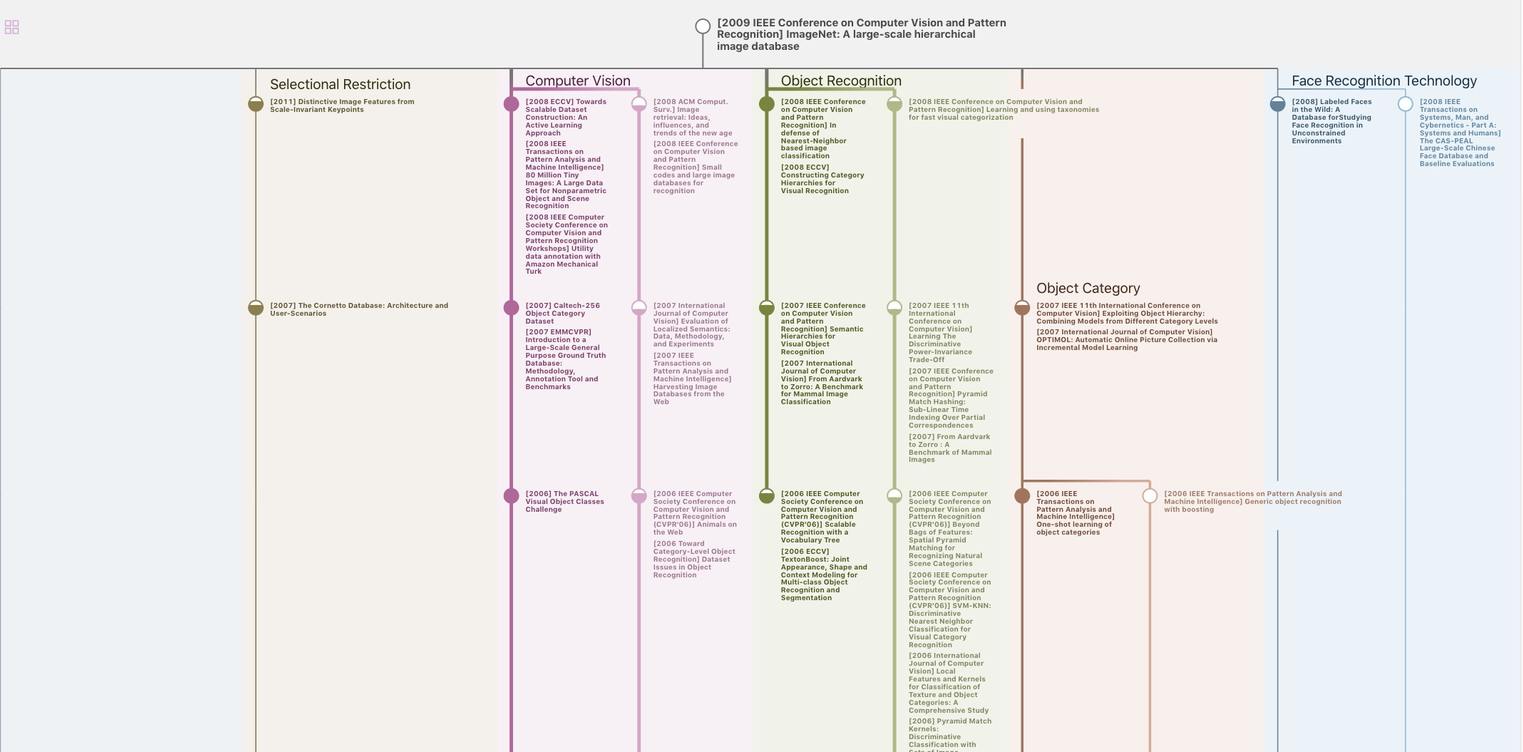
生成溯源树,研究论文发展脉络
Chat Paper
正在生成论文摘要