Enabling fast deep learning on tiny energy-harvesting IoT devices
DATE '22: Proceedings of the 2022 Conference & Exhibition on Design, Automation & Test in Europe(2022)
摘要
Energy harvesting (EH) IoT devices that operate intermittently without batteries, coupled with advances in deep neural networks (DNNs), have opened up new opportunities for enabling sustainable smart applications. Nevertheless, implementing those computation and memory-intensive intelligent algorithms on EH devices is extremely difficult due to the challenges of limited resources and intermittent power supply that causes frequent failures. To address those challenges, this paper proposes a methodology that enables fast deep learning with low-energy accelerators for tiny energy harvesting devices. We first propose RAD , a resource-aware structured DNN training framework, which employs block circulant matrix and structured pruning to achieve high compression for leveraging the advantage of various vector operation accelerators. A DNN implementation method, ACE , is then proposed that employs low-energy accelerators to profit maximum performance with small energy consumption. Finally, we further design F LEX , the system support for intermittent computation in energy harvesting situations. Experimental results from three different DNN models demonstrate that RAD, ACE , and FLEX can enable fast and correct inference on energy harvesting devices with up to 4.26X runtime reduction, up to 7.7X energy reduction with higher accuracy over the state-of-the-art.
更多查看译文
关键词
Deep Learning,Low-energy Accelerator,Energy Harvesting,IoT
AI 理解论文
溯源树
样例
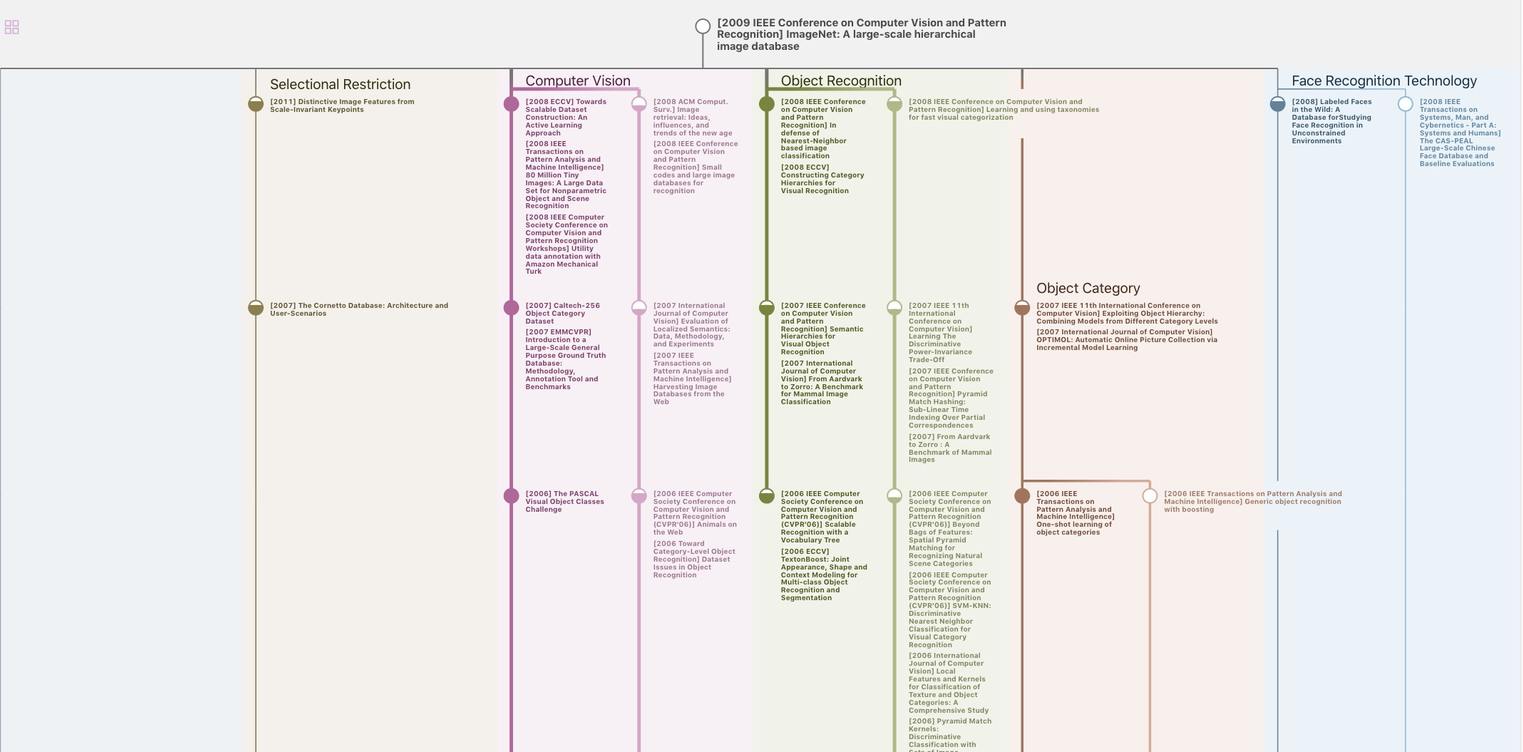
生成溯源树,研究论文发展脉络
Chat Paper
正在生成论文摘要