When Papers Choose Their Reviewers: Adversarial Machine Learning in Peer Review
ARTMAN '23: Proceedings of the 2023 Workshop on Recent Advances in Resilient and Trustworthy ML Systems in Autonomous Networks(2023)
摘要
Academia is thriving like never before. Thousands of papers are submitted to conferences on hot research topics, such as artificial intelligence and computer vision. To handle this growth, systems for automatic paper-reviewer assignments are increasingly used during the reviewing process. These systems employ statistical topic models from machine learning to characterize the content of papers and automate their assignment to reviewers. In this keynote talk, we explore the attack surface introduced by entrusting the matching of reviewers to machine-learning algorithms. In particular, we introduce an attack that modifies a given paper so that it selects its own reviewers. Technically, this attack builds on a novel optimization strategy that alternates between fooling the topic model and preserving the semantics of the document. In an empirical evaluation with a (simulated) conference, our attack successfully selects and removes reviewers in different scenarios, while the tampered papers remain indistinguishable from innocuous submissions to human readers. The talk is based on a paper by Eisenhofer & Quiring et al. [1] published at the USENIX Security Symposium in 2023.
更多查看译文
关键词
Adversarial Examples,Conference Management Systems,Problemspace Attacks,Statistical Topic Models
AI 理解论文
溯源树
样例
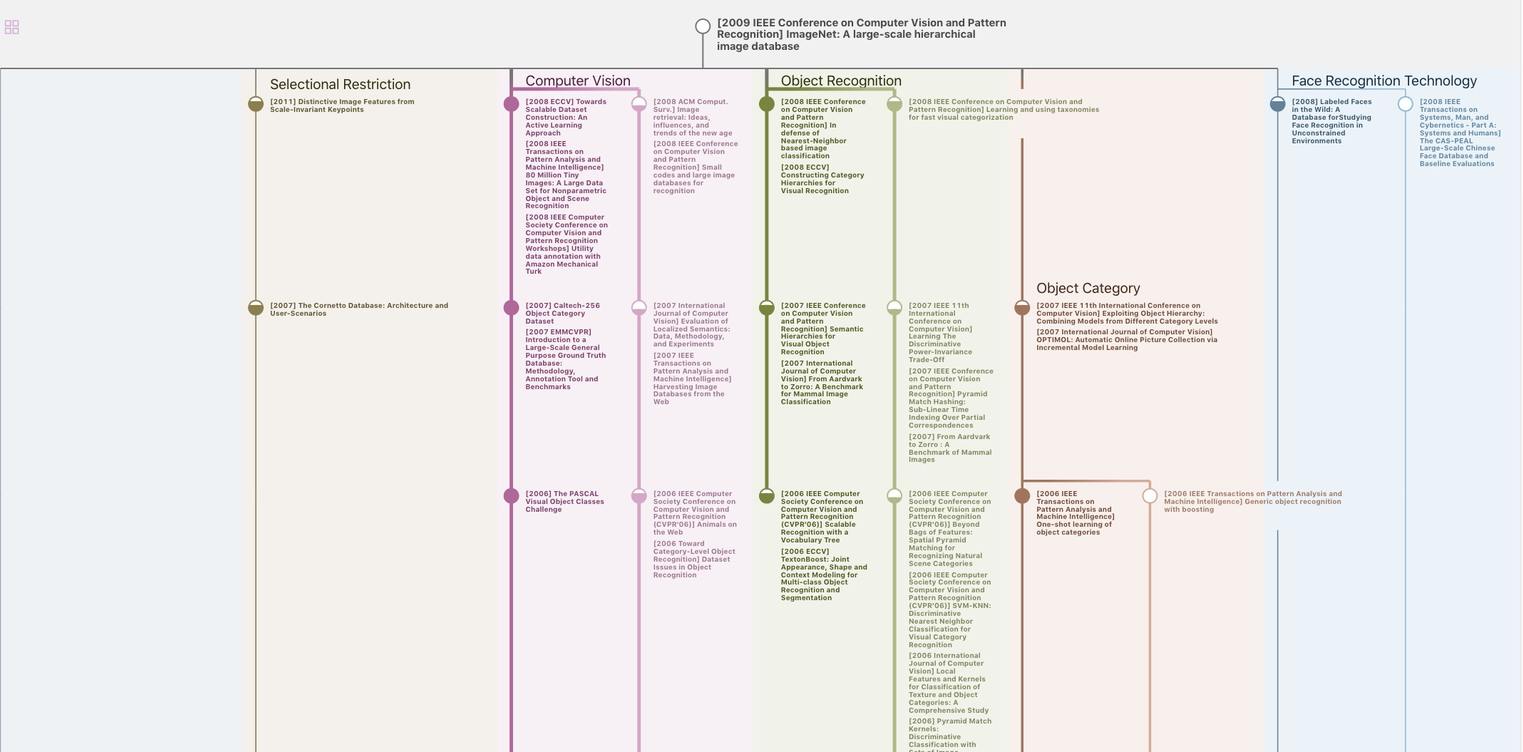
生成溯源树,研究论文发展脉络
Chat Paper
正在生成论文摘要