GGN: a model-free, data-driven deep learning framework for reconstructing gene regulatory networks
2023 13TH INTERNATIONAL CONFERENCE ON BIOSCIENCE, BIOCHEMISTRY AND BIOINFORMATICS, ICBBB 2023(2023)
摘要
Reconstructing gene regulatory networks based on time-series gene expression data is a huge challenge in the field of systems biology. However, the accuracy of traditional methods can be further improved. In practical situations, the gene regulatory network topology and the dynamic rules of genes are not observable. Therefore, reconstructing the underlying network structure and dynamics from observed time-series gene expression data is an important task. In this work, we introduce a new framework, Gumbel Graph Network (GGN), a model-free, data-driven deep learning framework for accomplishing the reconstruction of gene regulatory networks and the reconstruction of gene dynamics. Our model consists of two co-trained parts: a network generator, which generates a discrete network using Gumbel Softmax technique; and a dynamic learner, which uses the generated network and single-step trajectory values to predict the state at the next moment. According to the experimental results on multiple simulated and real datasets, GGN has better performance and stability compared with other state-of-the-art algorithms.
更多查看译文
关键词
Gumbel Graph Network,Network Generator,Gumbel Softmax Technique,Gynamic Learner,Gene Regulatory Network
AI 理解论文
溯源树
样例
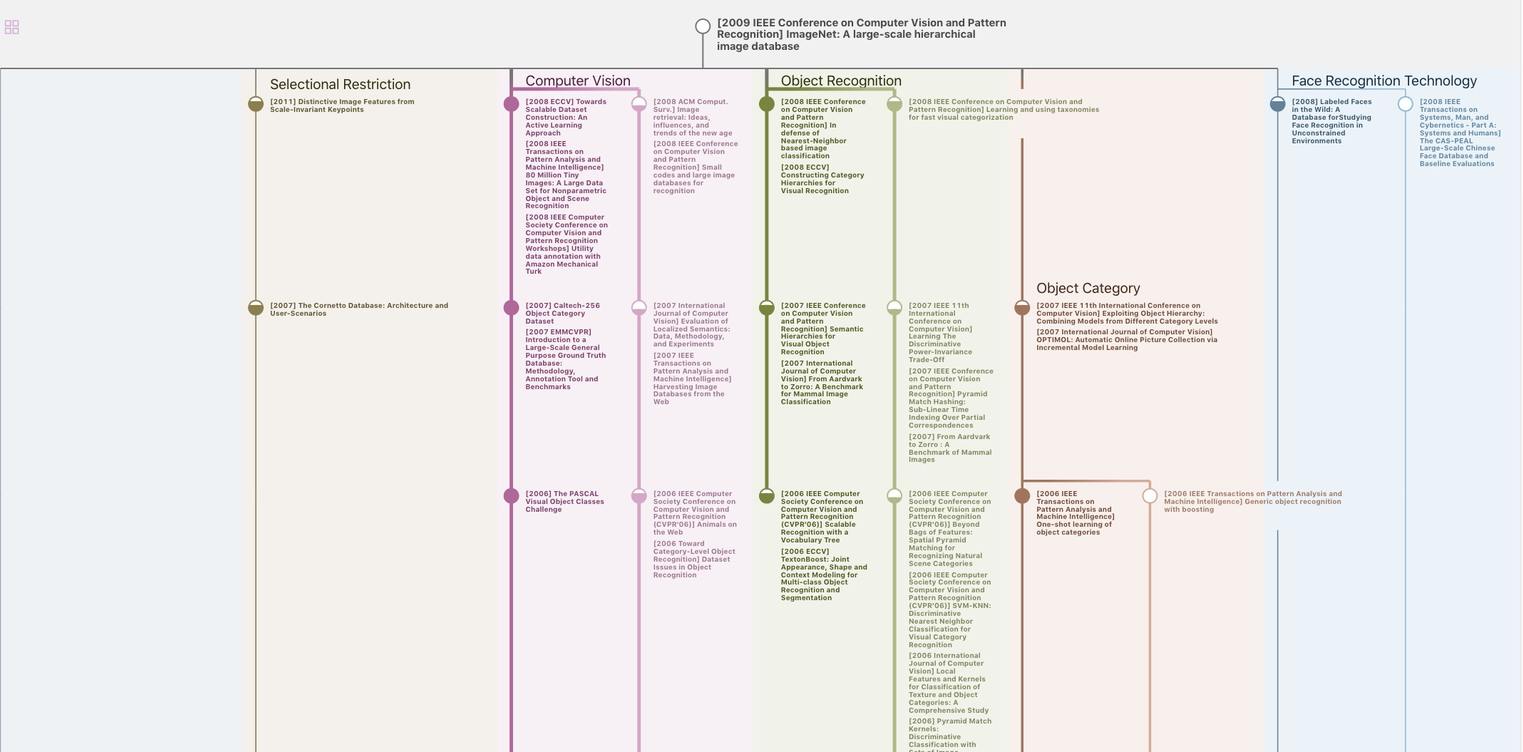
生成溯源树,研究论文发展脉络
Chat Paper
正在生成论文摘要