Deep Object Detection for Complex Architectural Floor Plans with Efficient Receptive Fields
CVIPPR '23: Proceedings of the 2023 Asia Conference on Computer Vision, Image Processing and Pattern Recognition(2023)
摘要
Architectural floor plans play an important role in sharing the building information among engineers, designers, and clients. Automatic floor plan analysis can help in improving work efficiency and accuracy. Object detection and recognition are critical in understanding and analyzing a floor plan document. However, few research works have been conducted to date for automatic object detection in architectural floor plans. In this paper, a convolutional neural network, namely ArchNet, is proposed to detect various visual objects, such as door, window, and stairs. The ArchNet is a modified version of YOLO network, and consists of five modules: backbone, multiscale receptive fields, neck, head, and non-maximal suppression. In this paper, ArchNet is used to detect 13 object classes commonly found in architectural floor plans. Experimental results show that the proposed architecture can achieve a mean average precision of 75% which is superior compared to the state-of-the-art techniques.
更多查看译文
AI 理解论文
溯源树
样例
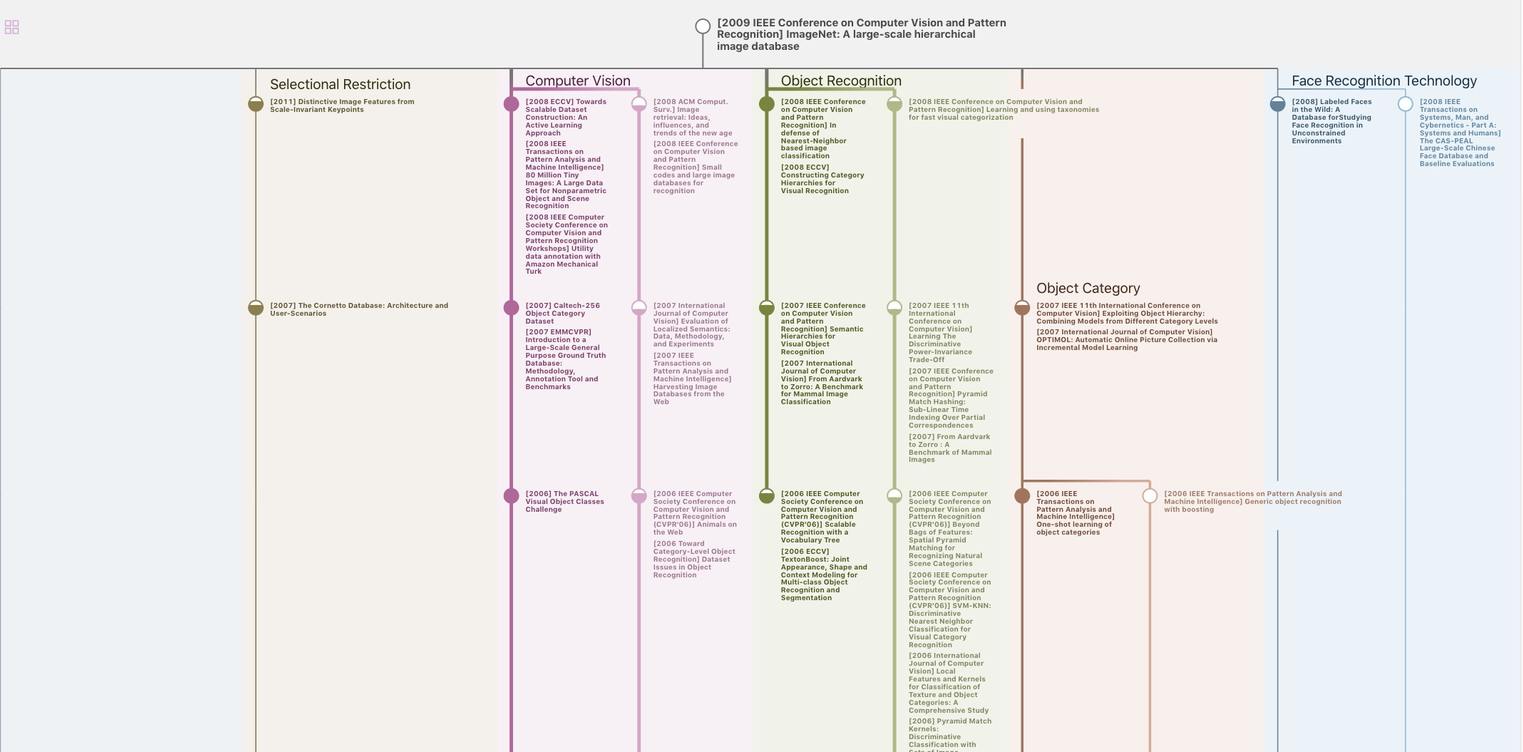
生成溯源树,研究论文发展脉络
Chat Paper
正在生成论文摘要