MGAM: Mixed-Gaussian Attention Module for Convolutional Neural Networks.
AIMLR '23: Proceedings of the 2023 Asia Conference on Artificial Intelligence, Machine Learning and Robotics(2023)
摘要
Abstract—Spatial attention holds great potential for enhancing the performance of convolutional neural networks (CNNs). This technique aims to select important spatial regions by generating attention masks across spatial domains. However, the majority of existing spatial attention works rely on convolutional blocks to compute attention weights, disregarding the modeling of spatial key positions. To address this limitation, this paper proposes a Mixed-Gaussian Attention Module (MGAM). The MGAM generates spatial attention masks that follow mixed Gaussian distribution, effectively highlighting the most discriminative semantic features and minimizing the influence of background noise while maintaining low model complexity. Our method based on ResNet50 achieves a 1.34% improvement on the CIFAR100 benchmark and a 1% improvement on the ImageNet-200 benchmark.
更多查看译文
AI 理解论文
溯源树
样例
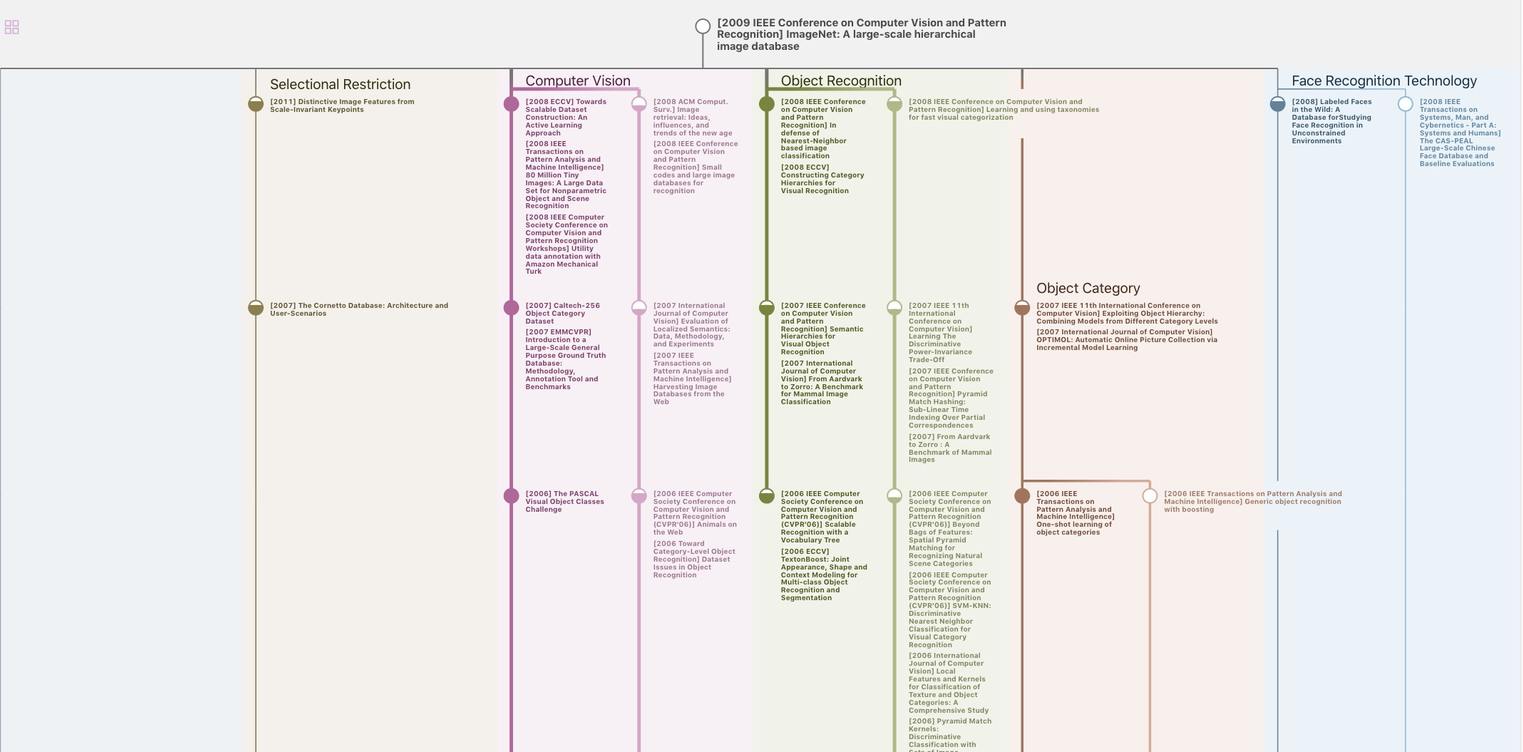
生成溯源树,研究论文发展脉络
Chat Paper
正在生成论文摘要