FEDGUARD: Selective Parameter Aggregation for Poisoning Attack Mitigation in Federated Learning
2023 IEEE INTERNATIONAL CONFERENCE ON CLUSTER COMPUTING, CLUSTER(2023)
摘要
Minimizing the attack surface of Federated Learning (FL) systems is a field of active research. FL turns out to be highly vulnerable to various threats coming from the edge of the network. Current approaches rely on robust aggregation, anomaly detection and generative models for defending against poisoning attacks. Yet, they either have limited defensive capabilities due to their underlying design or are impractical to use as they rely on constraining building blocks. We introduce FEDGUARD, a novel FL framework that utilizes the generative capabilities of Conditional Variational AutoEncoders (CVAE) to effectively defend against poisoning attacks with tuneable overhead in communication and computation. Whilst the idea of hardening a FL system using generative models is not entirely new, FEDGUARD's original contribution is in its selective parameter aggregation operator with parameter selection being driven by synthetic validation data sampled from the CVAEs trained locally by each participating party. Experimental evaluations in a 100-client setup demonstrates FEDGUARD to be more effective than previous approaches against several types of attacks (label and sign flipping, additive noise, same value attacks). FEDGUARD successfully defends in scenarios with up to 50% malicious peers where other strategies fail. In addition, FEDGUARD does not require auxiliary datasets or centralized (pre-) training. It provides resilience against poisoning attacks from the very first round of federated training.
更多查看译文
关键词
federated learning,malicious peer detection,robust federated learning,adversarial attacks,generative models
AI 理解论文
溯源树
样例
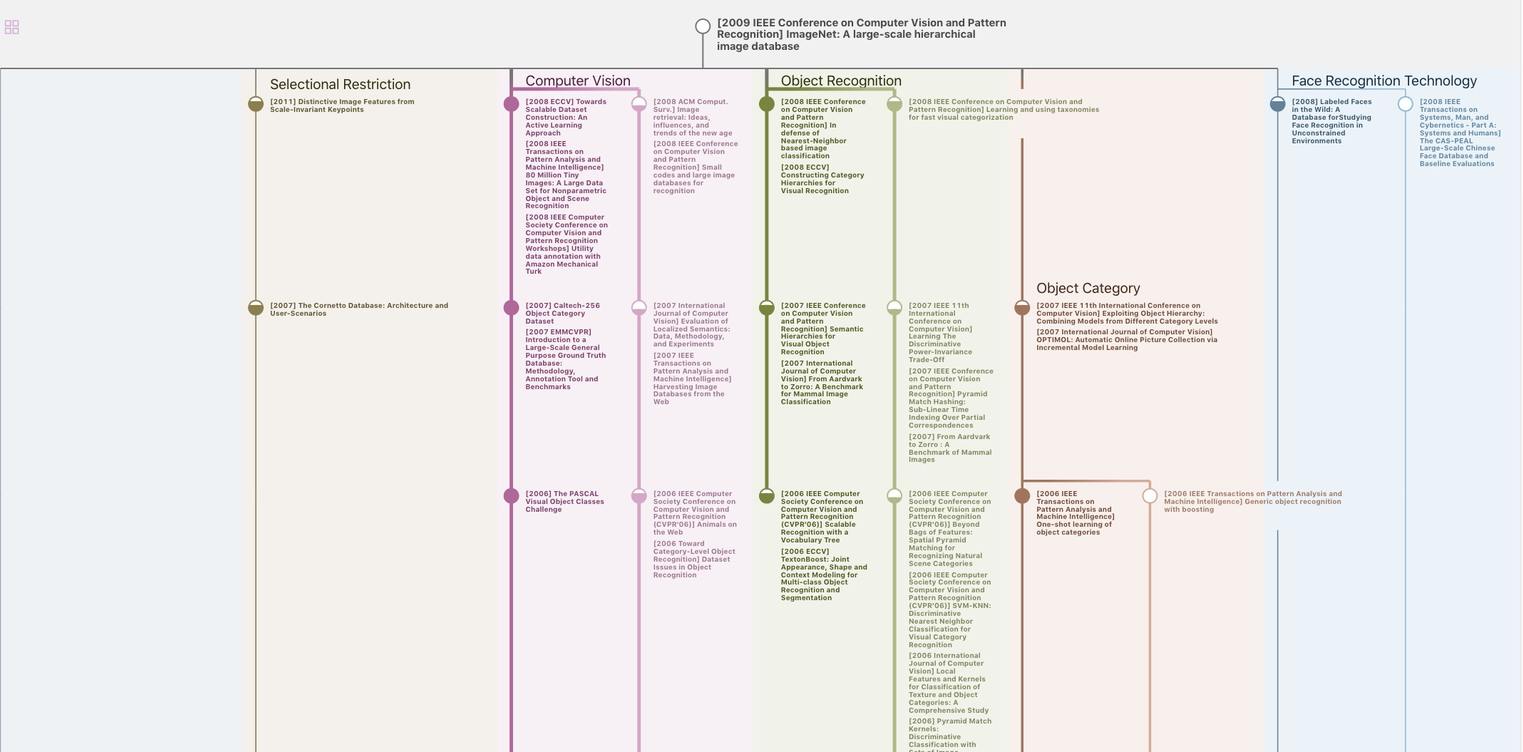
生成溯源树,研究论文发展脉络
Chat Paper
正在生成论文摘要