BioPRO: Context-Infused Prompt Learning for Biomedical Entity Linking
IEEE-ACM TRANSACTIONS ON AUDIO SPEECH AND LANGUAGE PROCESSING(2024)
摘要
Recent research tends to address the biomedical entity linking problem in a unified framework solely based on surface form matching between mentions and entities. Specifically, these methods focus on addressing the variety challenge of the heterogeneous naming of biomedical concepts. Yet, the ambiguity challenge that the same word under different contexts can be used to refer to distinct concepts is usually ignored. To address this challenge, we propose BioPRO, a two-stage entity linking algorithm to enhance the biomedical entity representations based on context-infused prompt learning. The first stage includes a coarse-grained retrieval from a representation space defined by a bi-encoder that independently embeds the mention and entity's surface forms. Unlike previous one-model-fits-all systems, each candidate is then re-ranked with a fine-grained encoder based on prompt-tuning that sufficiently stimulates knowledge in contextual information of mentions and entities. Furthermore, the trained fine-grained encoder can be utilized to generate deep representations of bio-entities and boost candidate retrieval in the first stage. Extensive experiments show that our model achieves promising performance improvements compared with several state-of-the-art (SOTA) techniques on 4 biomedical corpora. We also observe by cases that the proposed context-infused prompt-tuning strategy is effective in solving both the variety and ambiguity challenges in the linking task.
更多查看译文
关键词
Biological system modeling,Task analysis,Unified modeling language,Context modeling,Surface treatment,Speech processing,Semantics,Biomedical entity linking,biomedical entity representations,context-infused prompt learning,deep learning
AI 理解论文
溯源树
样例
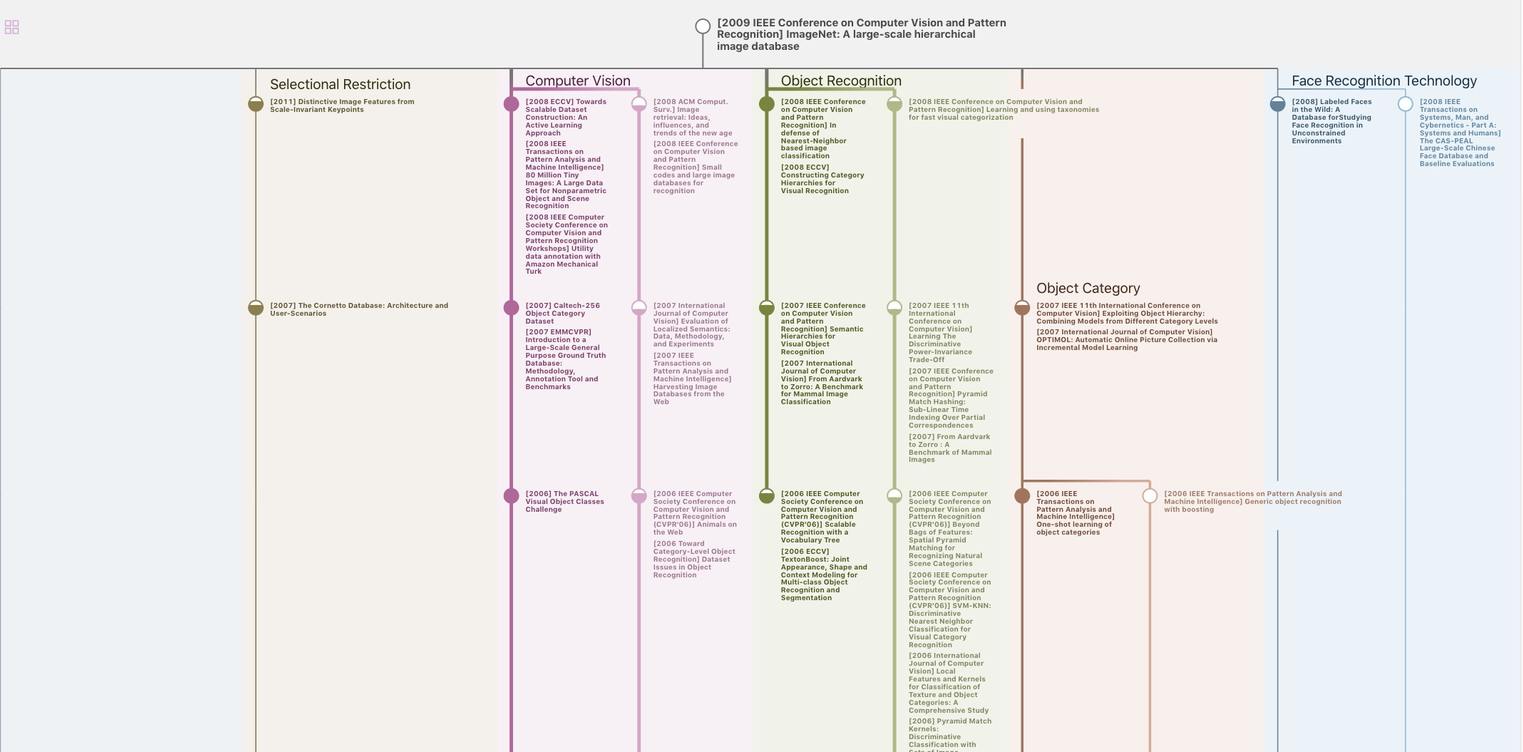
生成溯源树,研究论文发展脉络
Chat Paper
正在生成论文摘要