Self-Supervised On-Device Federated Learning From Unlabeled Streams
IEEE TRANSACTIONS ON COMPUTER-AIDED DESIGN OF INTEGRATED CIRCUITS AND SYSTEMS(2023)
摘要
The ubiquity of edge devices has led to a growing amount of unlabeled data produced at the edge. Deep learning models deployed on edge devices are required to learn from these unlabeled data to continuously improve accuracy. Self-supervised representation learning has achieved promising performances using centralized unlabeled data. However, the increasing awareness of privacy protection limits centralizing the distributed unlabeled image data on edge devices. While federated learning has been widely adopted to enable distributed machine learning with privacy preservation, without a data selection method to efficiently select streaming data, the traditional federated learning framework fails to handle these huge amounts of decentralized unlabeled data with limited storage resources on edge. To address these challenges, we propose a self-supervised on-device federated learning framework with coreset selection, which we call SOFed, to automatically select a coreset that consists of the most representative samples into the replay buffer on each device. It preserves data privacy as each client does not share raw data while learning good visual representations. Experiments demonstrate the effectiveness and significance of the proposed method in visual representation learning.
更多查看译文
关键词
Federated contrastive learning (FCL),learning representations,on-device learning,self-supervised learning
AI 理解论文
溯源树
样例
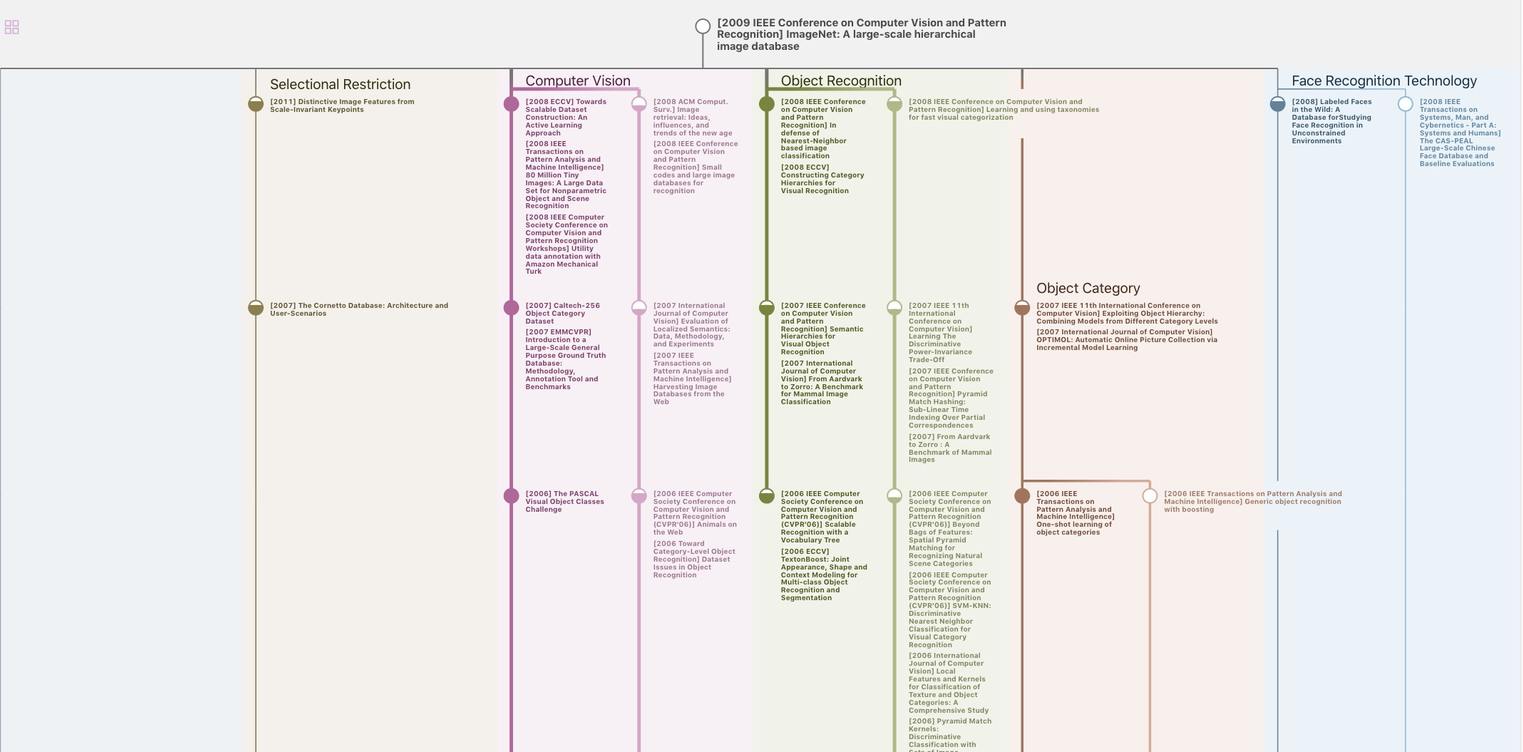
生成溯源树,研究论文发展脉络
Chat Paper
正在生成论文摘要