Universal Heterogeneous Face Analysis via Multi-Domain Feature Disentanglement
IEEE TRANSACTIONS ON INFORMATION FORENSICS AND SECURITY(2024)
摘要
Heterogeneous face analysis is an important and challenge problem in face recognition community, because of the large modality discrepancy between heterogeneous face images. Existing methods either focus on transforming heterogeneous faces into the same style via face synthesis process, or intend to directly recognize heterogeneous face via modality invariant descriptors. However, the tasks of cross modality face synthesis and face recognition share a common purpose, which is to disentangle an inherent explainable representation. To this end, we propose a novel universal heterogenous face analysis method via multi-domain feature disentanglement, which does not need any face domain label. The proposed method explores to disentangle factors of variations of cross modality faces in an unsupervised manner. Then we could translate cross modality faces through modifying semantic factors, and the extracted inherent explainable representation still maintains being discriminative for heterogeneous face recognition. Experimental results on multiple cross modality face databases demonstrate the effectiveness of the proposed method. These experimental results also inspire us that the unsupervised disentangled module could help to analyze the interpretability of heterogenous face representation.
更多查看译文
关键词
Face recognition,Feature extraction,Task analysis,Semantics,Intserv networks,Image recognition,Faces,Cross modality face,disentangled representation,heterogeneous face analysis
AI 理解论文
溯源树
样例
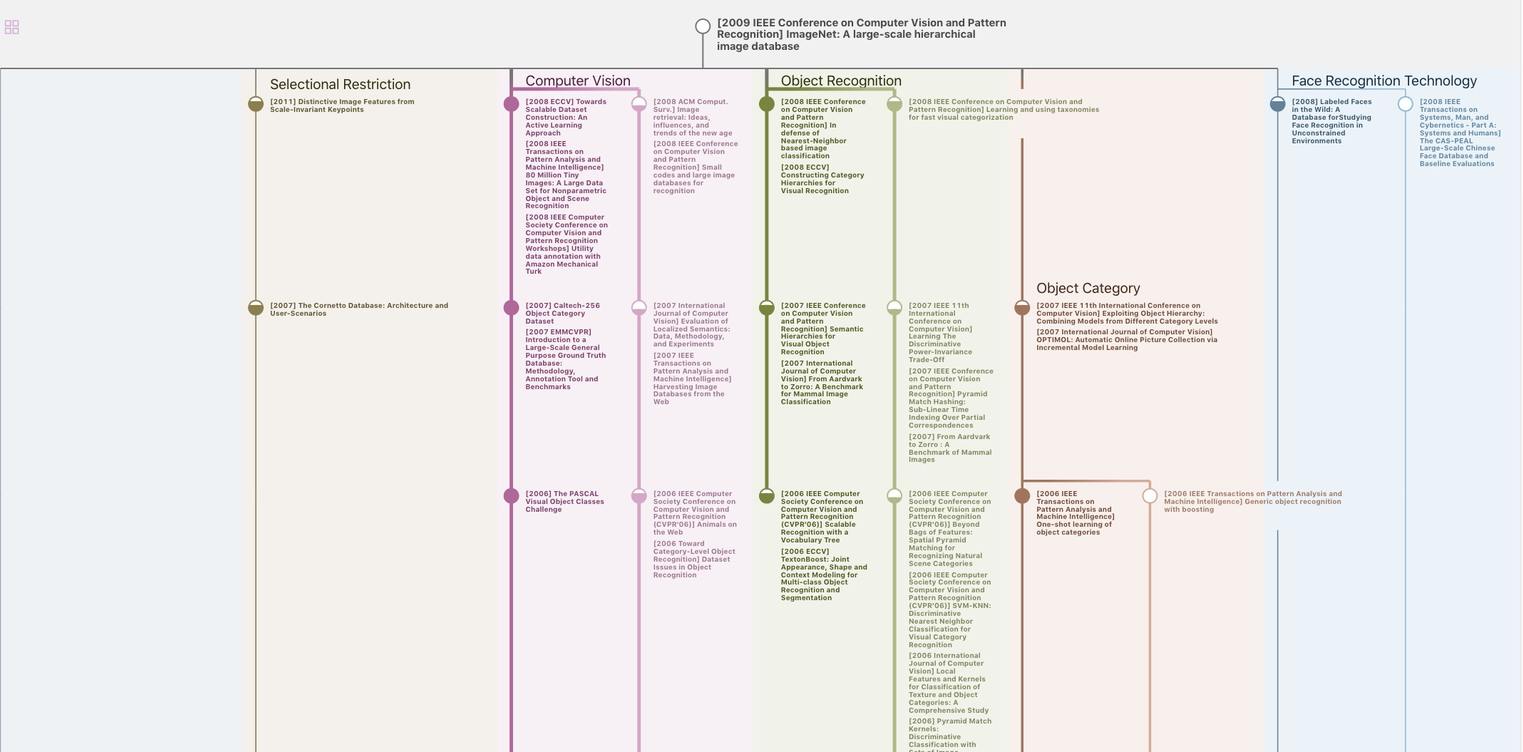
生成溯源树,研究论文发展脉络
Chat Paper
正在生成论文摘要