Radio Frequency Fingerprinting Identification of Few-Shot Wireless Signals Based on Deep Metric Learning
Wireless Communications & Mobile Computing(2023)
摘要
As a cross-protocol endogenous security mechanism, the physical layer-based radio frequency (RF) fingerprinting can effectively enhance the existing password-based application layer authentication utilizing the hardware differences of wireless devices, which is unique and cannot be counterfeited by a third party. However, the recognition performance of the deep learning physical layer fingerprint recognition algorithm drops sharply in the case of a small number of signal samples. This paper analyzes the feasibility and proposes the few-shot wireless signal classification network based on deep metric learning (FSig-Net). FSig-Net reduces the model’s dependence on big data by adaptively learning the feature distance metric. We use 8 mobile phones and 18 Internet of Things (IoT) modules as targets for identification. When the number of single-type samples is only 10, the recognition accuracy of mobile phones can reach 98.28%, and the recognition accuracy of IoT devices can reach 98.20%.
更多查看译文
关键词
radio,few-shot
AI 理解论文
溯源树
样例
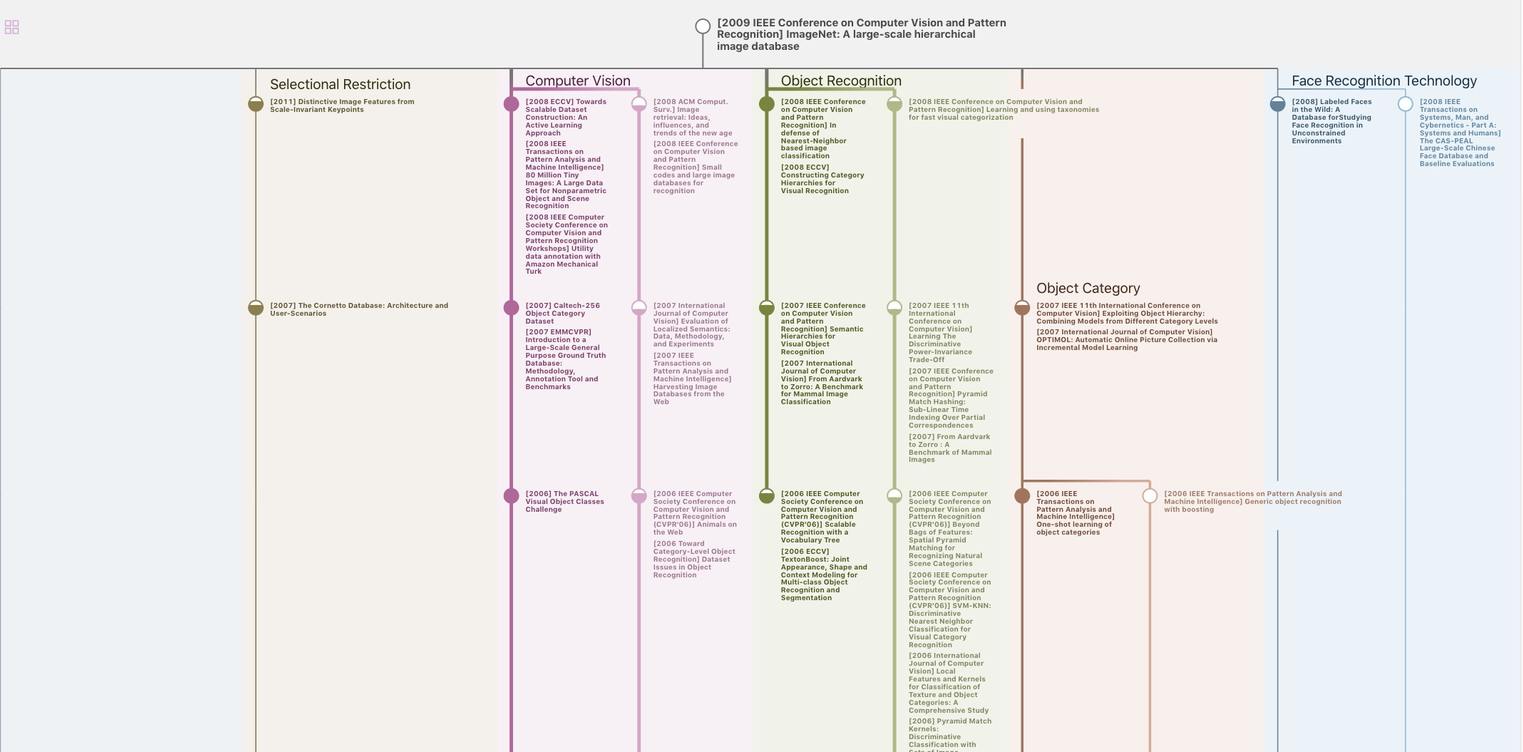
生成溯源树,研究论文发展脉络
Chat Paper
正在生成论文摘要