Robust
Speech Communication(2020)
摘要
• The proposed f 0 estimation method performs reasonably well for neutral speech, songs and emotional speech. Whereas the existing f 0 estimation methods are confined to either speech or songs. • The RNN-LSTM based framework is introduced in the proposed f 0 estimation method for detecting the voicing/unvoicing frames. • The proposed sub-band structure ensures in deriving the mono-component signal (equivalent to f 0 ) for both speech and songs. • The applicability of the proposed f 0 estimation method is demonstrated by developing the Tonic-independent automatic SARGAM learning system. Fundamental frequency ( f 0 ) extraction plays an important role in processing of monophonic signals such as speech and song. It is essential in various real-time applications such as emotion recognition, speech/singing voice discrimination and so on. Several f 0 extraction methods have been proposed over the years, but no one algorithm works well for both speech and song. In this paper, we propose a novel approach that can accurately estimate f 0 from speech as well as songs. First, voiced/unvoiced detection is performed using a novel RNN-LSTM based approach. Then, each voiced frame is decomposed into several sub-bands. From each sub-band of a voiced frame, the candidate pitch periods are identified using autocorrelation and non-linear operations. Finally, Viterbi decoding is used to form the final pitch contours. The performance of the proposed method is evaluated using popular speech (Keele, CMU-ARCTIC), and song (MIR-1K, LYRICS) databases. The evaluation results show that the proposed method performs equally well for speech and monophonic songs, and is better than the state-of-the-art methods. Further, the efficacy of proposed f 0 extraction method is demonstrated by developing an interactive SARGAM learning tool.
更多查看译文
关键词
Fundamental frequency,Speech,Song,Non-linear filtering,Autocorrelation,LSTM,SARGAM
AI 理解论文
溯源树
样例
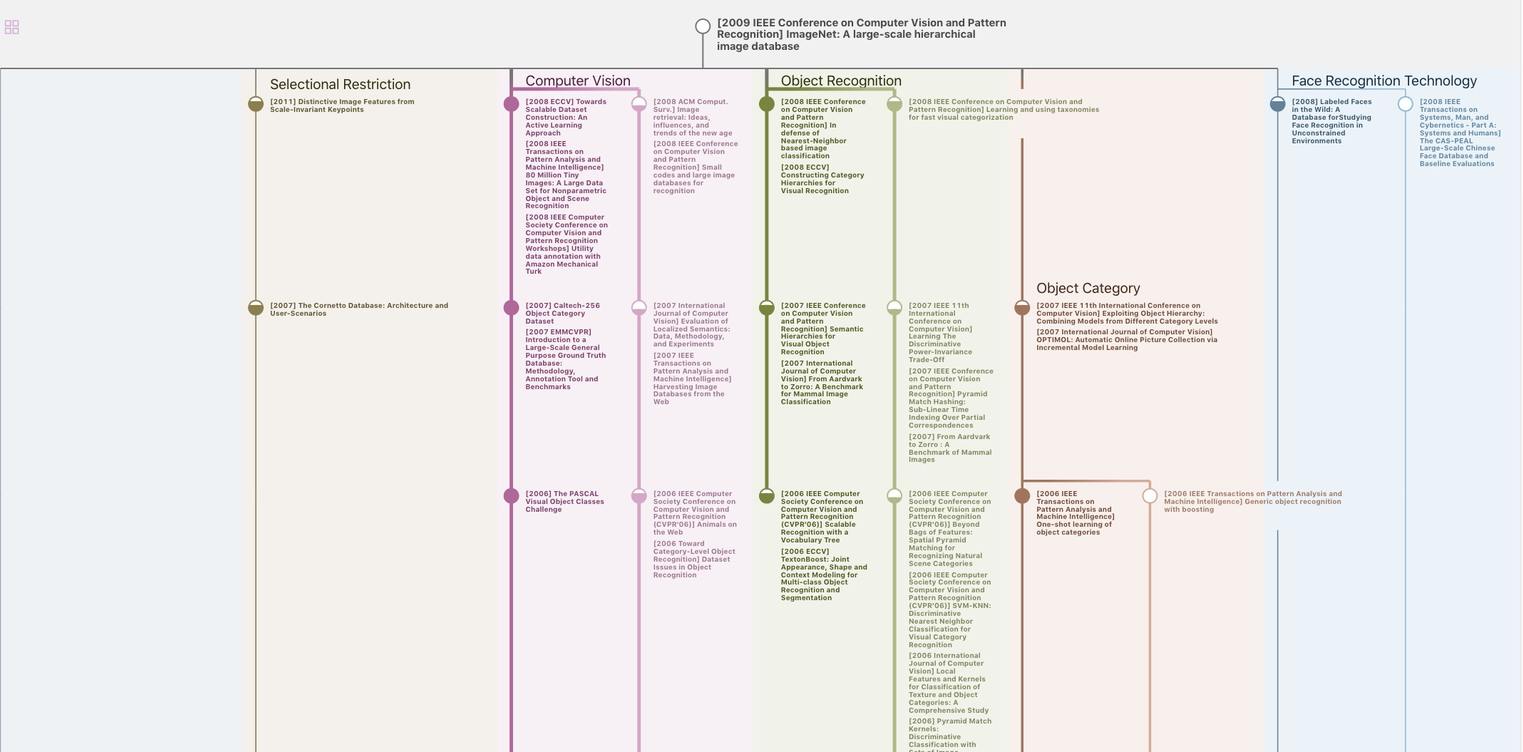
生成溯源树,研究论文发展脉络
Chat Paper
正在生成论文摘要