Modeling volatility for high-frequency data with rounding error: a nonparametric Bayesian approach
Statistics and Computing(2023)
摘要
Rounding is a pivotal source of market microstructure noise which should be carefully addressed in high-frequency data analysis. This study incorporates available market information in modeling rounding errors and proposes a Rounding Error-Trading Information model from the Bayesian perspective. We assign a thresholded Gaussian process prior to the instantaneous volatility of the log-price process and adopt a fully Bayesian approach with an efficient Markov chain Monte Carlo algorithm for model inference, based on which a novel Trading Information-based estimator for the integrated volatility is provided. Simulation studies show that the proposed method can effectively recover the true latent log-prices, instantaneous volatility, and integrated volatility with multiple volatility shapes and rounding mechanisms, even under model misspecification. Extensive empirical studies show that the rounding mechanism in the Shanghai A-share market is likely to be random and the trading directions have a greater impact on the rounding results of the asset prices than the true latent log-prices, which is a consistent finding with that in the literature.
更多查看译文
关键词
Bayesian,High frequency data,Integrated volatility,Market microstructure noise,Market trading information
AI 理解论文
溯源树
样例
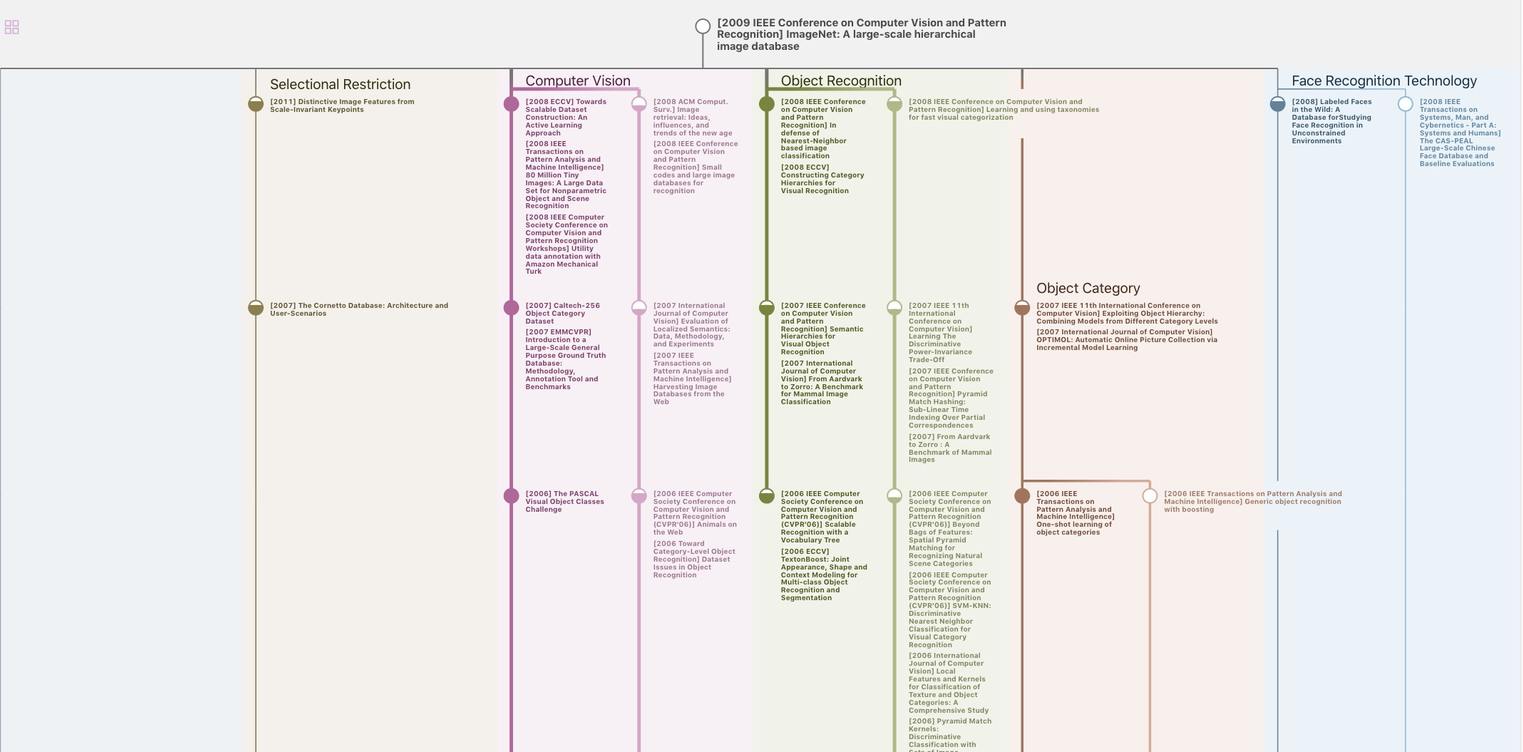
生成溯源树,研究论文发展脉络
Chat Paper
正在生成论文摘要