A random forest approach to improve estimates of tributary nutrient loading
WATER RESEARCH(2024)
摘要
Estimating constituent loads from discrete water quality samples coupled with stream discharge measurements is critical for management of freshwater resources. Nutrient loads calculated based on discharge-concentration relationships form the basis of government nutrient load targets and scientific studies of the response of receiving waters to external loads. In this study, a new model is developed using random forests and applied to estimate concentrations and loads of total phosphorus, dissolved phosphorus, total nitrogen, and chloride, using data from 17 tributaries to Lake Champlain monitored from 1992 to 2021. I benchmark this model against one of the most widespread models currently used to estimate nutrient loads, Weighted Regressions on Time, Discharge, and Season (WRTDS). The random forest model outperformed both the base WRTDS model and an extension of the WRTDS model using Kalman filtering in the great majority of cases, likely due to the inclusion of rate-ofchange in discharge and antecedent discharge over different leading windows as predictors, and to the flexibility of the random forest to model predictor-response relationships. The random forest also had useful visualization capabilities which provided important process insights. WRTDS remains a useful model for many applications, but this study represents a promising new approach for load estimation which can be applied easily to existing datasets, and which is easy to customize for different applications.
更多查看译文
关键词
Nutrient load estimation,Random forest,WRTDS
AI 理解论文
溯源树
样例
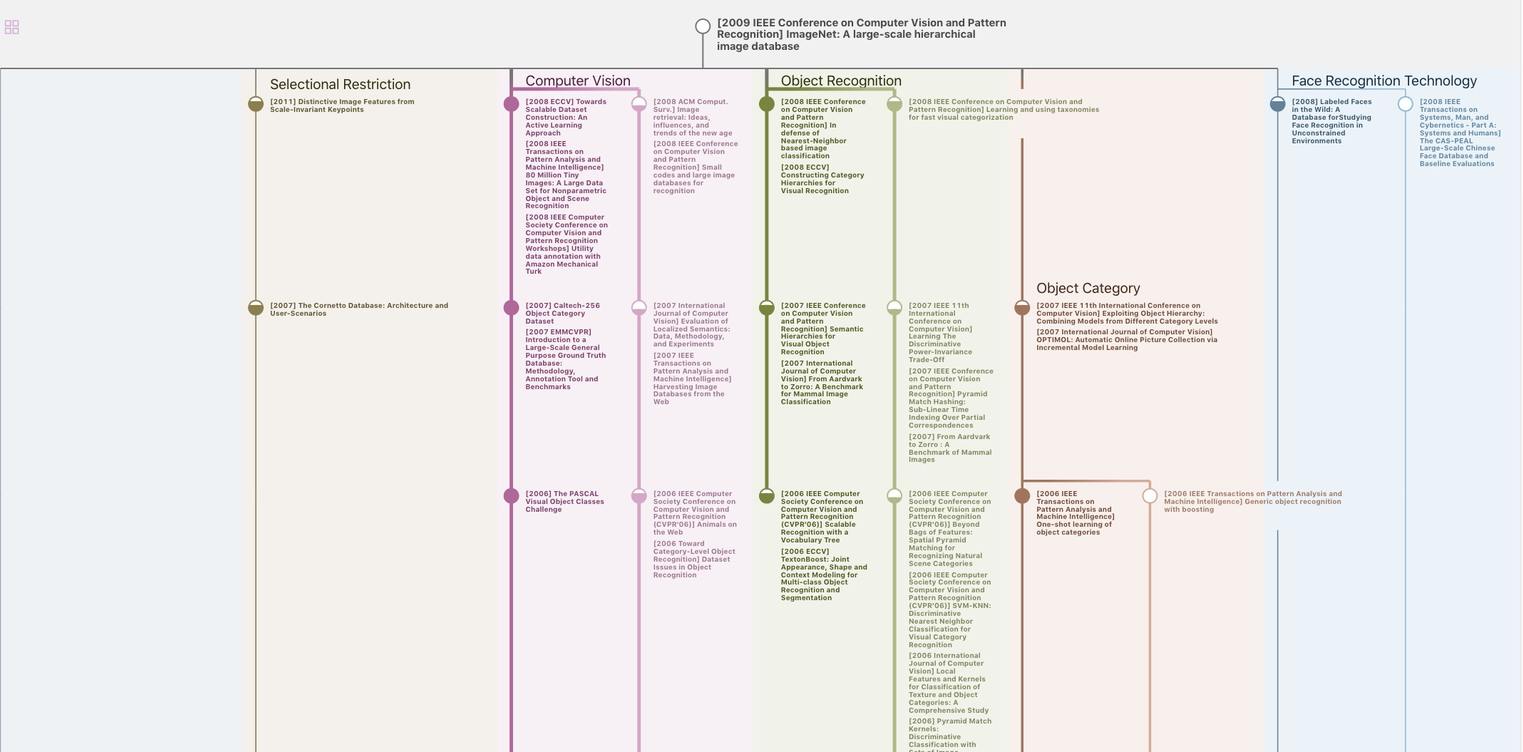
生成溯源树,研究论文发展脉络
Chat Paper
正在生成论文摘要