Proportional Volume Sampling and Approximation Algorithms for A-Optimal Design
Mathematics of Operations Research(2022)
摘要
We study optimal design problems in which the goal is to choose a set of linear measurements to obtain the most accurate estimate of an unknown vector. We study the A-optimal design variant where the objective is to minimize the average variance of the error in the maximum likelihood estimate of the vector being measured. We introduce the proportional volume sampling algorithm to obtain nearly optimal bounds in the asymptotic regime when the number k of measurements made is significantly larger than the dimension d and obtain the first approximation algorithms whose approximation factor does not degrade with the number of possible measurements when k is small. The algorithm also gives approximation guarantees for other optimal design objectives such as D-optimality and the generalized ratio objective, matching or improving the previously best-known results. We further show that bounds similar to ours cannot be obtained for E-optimal design and that A-optimal design is NP-hard to approximate within a fixed constant when k=d.
更多查看译文
关键词
Primary: 90C27,secondary: 62J05,62K05,62K15,68W20,68W25,optimal design,design of experiments,A-optimal design,D-optimal design,generalized ratio objective,linear regression,volume sampling,proportional volume sampling,approximation algorithms,NP-hardness
AI 理解论文
溯源树
样例
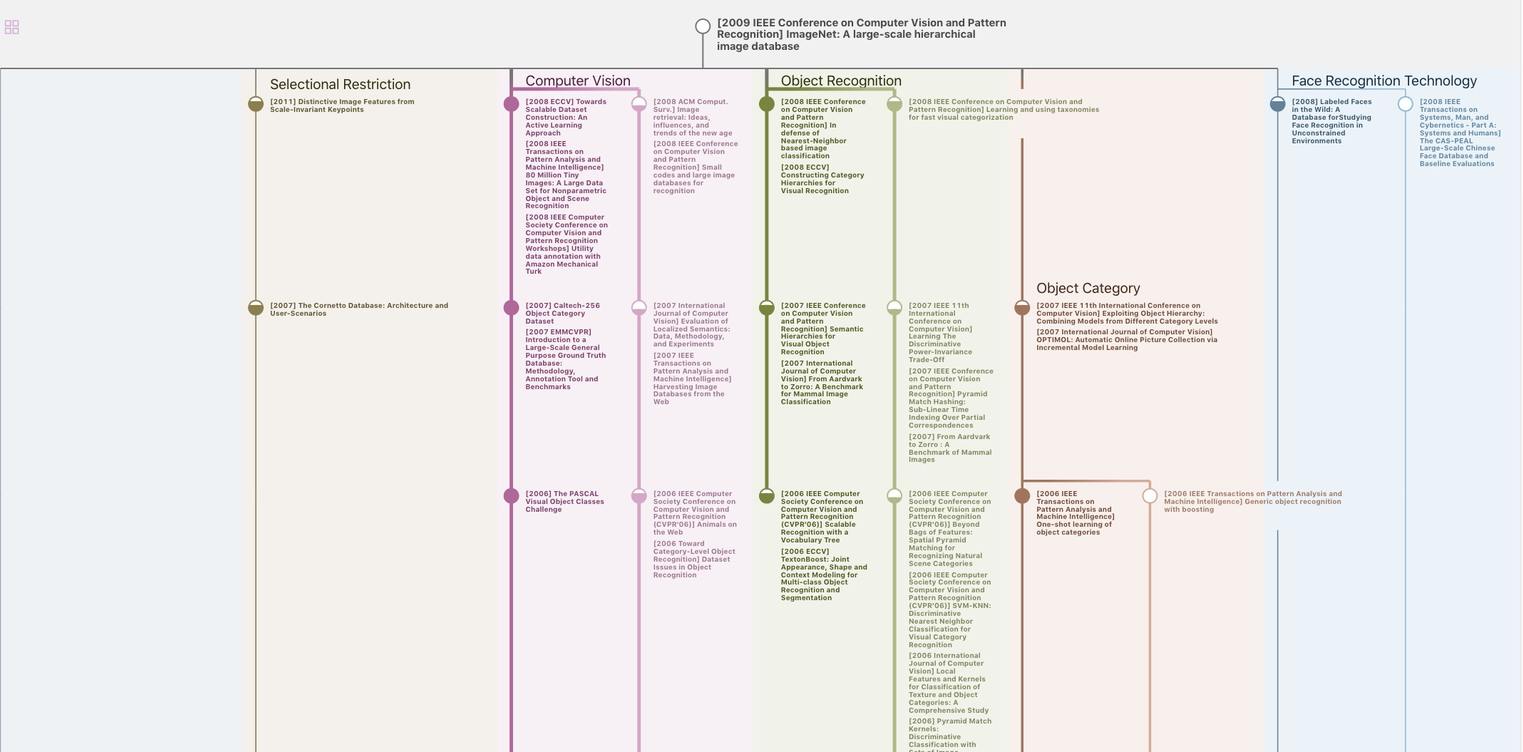
生成溯源树,研究论文发展脉络
Chat Paper
正在生成论文摘要