Self-Supervised Deconfounding Against Spatio-Temporal Shifts: Theory and Modeling
arxiv(2023)
摘要
As an important application of spatio-temporal (ST) data, ST traffic
forecasting plays a crucial role in improving urban travel efficiency and
promoting sustainable development. In practice, the dynamics of traffic data
frequently undergo distributional shifts attributed to external factors such as
time evolution and spatial differences. This entails forecasting models to
handle the out-of-distribution (OOD) issue where test data is distributed
differently from training data. In this work, we first formalize the problem by
constructing a causal graph of past traffic data, future traffic data, and
external ST contexts. We reveal that the failure of prior arts in OOD traffic
data is due to ST contexts acting as a confounder, i.e., the common cause for
past data and future ones. Then, we propose a theoretical solution named
Disentangled Contextual Adjustment (DCA) from a causal lens. It differentiates
invariant causal correlations against variant spurious ones and deconfounds the
effect of ST contexts. On top of that, we devise a Spatio-Temporal
sElf-superVised dEconfounding (STEVE) framework. It first encodes traffic data
into two disentangled representations for associating invariant and variant ST
contexts. Then, we use representative ST contexts from three conceptually
different perspectives (i.e., temporal, spatial, and semantic) as
self-supervised signals to inject context information into both
representations. In this way, we improve the generalization ability of the
learned context-oriented representations to OOD ST traffic forecasting.
Comprehensive experiments on four large-scale benchmark datasets demonstrate
that our STEVE consistently outperforms the state-of-the-art baselines across
various ST OOD scenarios.
更多查看译文
AI 理解论文
溯源树
样例
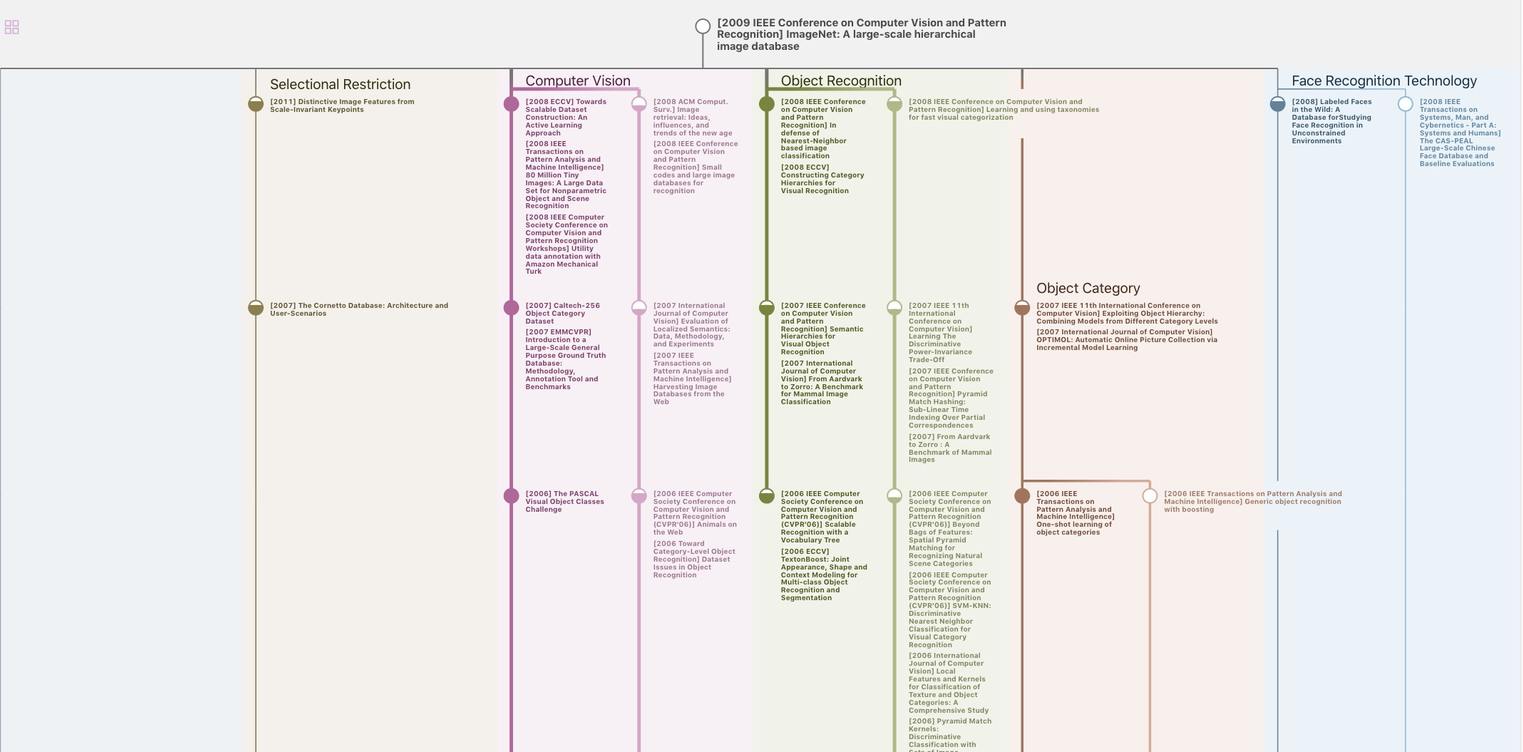
生成溯源树,研究论文发展脉络
Chat Paper
正在生成论文摘要