Semi-supervised
Neurocomputing(2023)
摘要
• A semi-supervised framework based on similarity preservation for dimensionality reduction is presented. • Class-label and data structure insights are coupled for neighbourhood preservation based on the well-known t-SNE approach. • Our SS. t -SNE is a well-founded generalization of the t -SNE method from multi-scale neighborhood preservation and class-label coupling within a divergence-based loss. • Visualization, rank, and classification performance criteria are tested on synthetic and real-world datasets devoted to dimensionality reduction and data discrimination. Unsupervised dimensionality reduction (DR) aims to preserve input data structure in a low-dimensional (LD) space based on neighborhood information. In contrast, supervised DR intends to improve the learning performance, i.e., classification and regression, in an LD representation. Unfortunately, obtaining the complete label outputs of a data set for real-world applications is hard. Here, we introduce a novel DR framework coupling both available class labels and input feature similarities to extend the well-known t -distributed Stochastic Neighbor Embedding (SNE) for semi-supervised scenarios. Our proposal, termed Semi-Supervised t -SNE (SS. t -SNE), properly fixes the widths of Gaussian neighborhoods to reveal the salient local and global data structures in an LD space. Indeed, our approach is presented as a generalization of unsupervised and supervised versions of t -SNE. SS. t -SNE outperforms other semi-supervised DR methods in data visualization and classification tasks in LD embeddings.
更多查看译文
关键词
Dimensionality reduction,Stochastics neighbor embedding,Semi-supervised learning,Neighborhood preservation,Data visualization
AI 理解论文
溯源树
样例
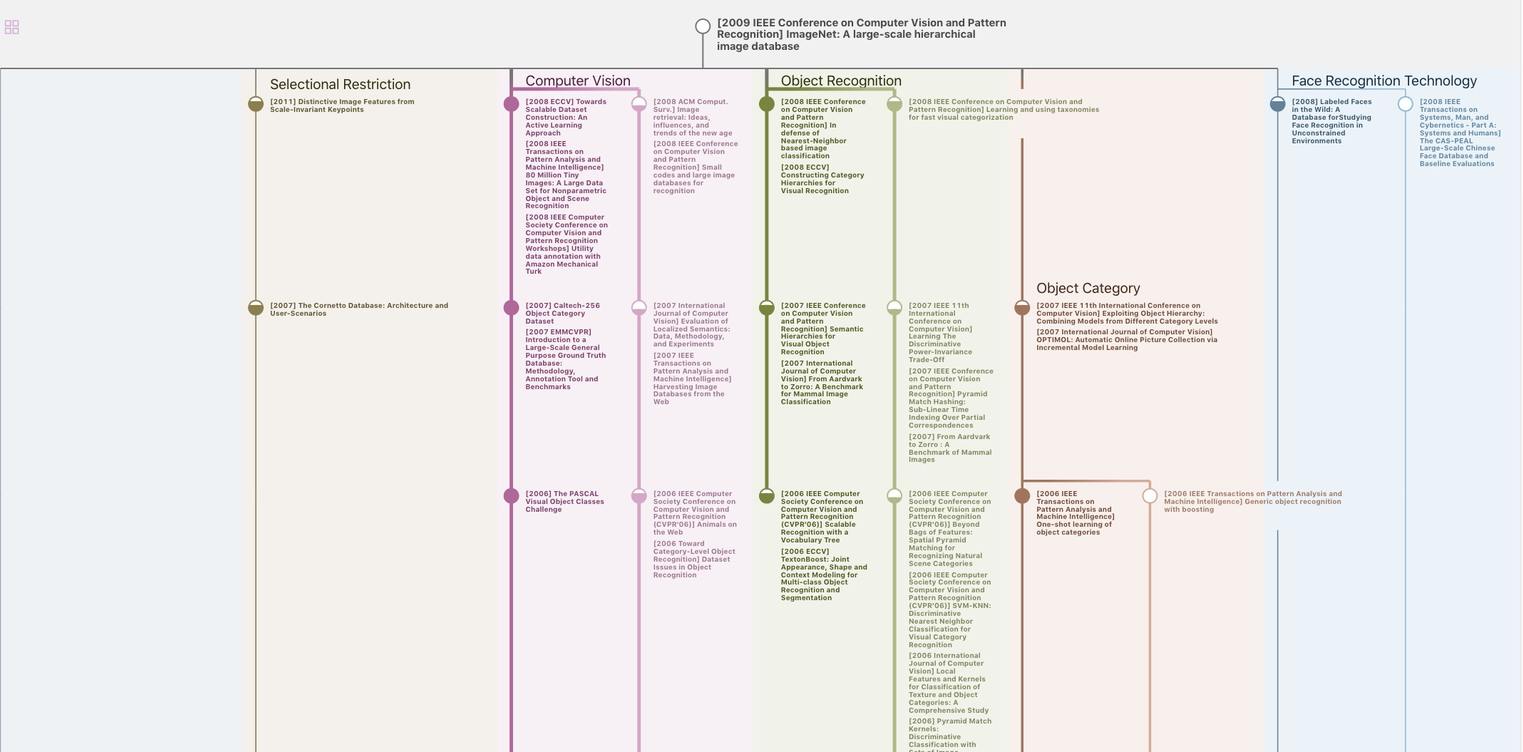
生成溯源树,研究论文发展脉络
Chat Paper
正在生成论文摘要