Predicting Water Quality Estimates Using Satellite Images in Coastal and Estuarine Environments
Journal of Computing Sciences in Colleges(2022)
摘要
Water is integral to all environments. As such, its quality is continuously monitored by sensors and satellites. Satellite imagery is very useful in monitoring water quality in deep waters, further offshore. However, shallow bodies of water, such as coastal areas and estuaries prove more difficult to measure due to the variability in the environment and their considerably lesser depth. To mitigate this shortfall, sensors are used to monitor water quality in coastal areas and estuaries; though, this application is limited due to its high cost. Nevertheless, we aimed to use satellite images alone in order to predict water quality in coastal and estuarine ecosystems, specifically, Zeke's Basin in Fort Fisher, North Carolina (-77.93500, 33.95470). We built multiple machine learning algorithms with this aim in mind. Our dataset was a combination of Landsat 8 image data and NOAA's National Estuaries Research Reserve System (NERRS). We used principal component analysis to find correlations within our dataset. Then we trained 4 models, a Decision Tree Regressor, AdaBoost Regressor, Bagging Regressor, and a Random Forest Regressor. The model that created the most accurate turbidity estimates was the AdaBoost Regressor with a Decision Tree Regressor estimator. Using the Ababoost Regressor, we achieved an r-squared of 0.9751.
更多查看译文
AI 理解论文
溯源树
样例
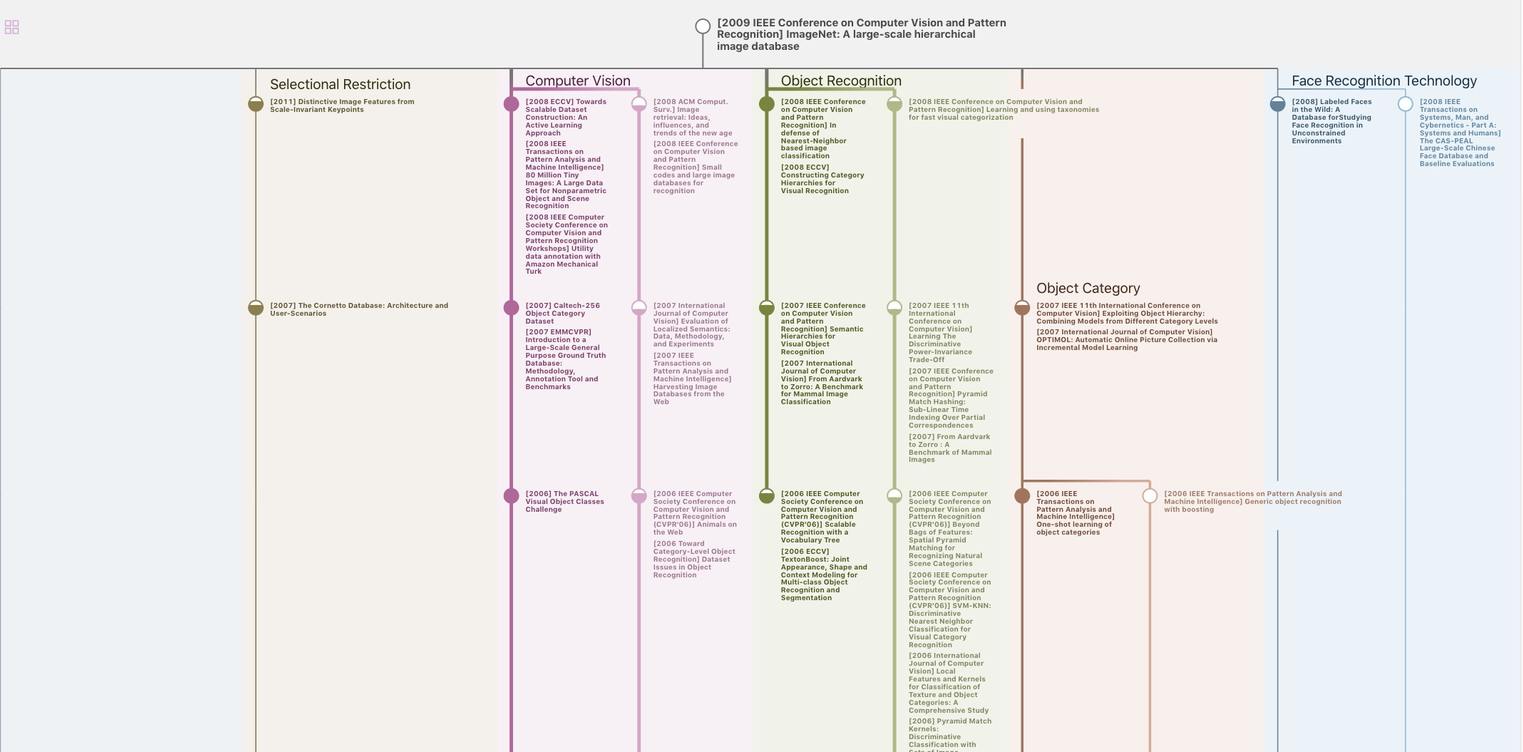
生成溯源树,研究论文发展脉络
Chat Paper
正在生成论文摘要