Solving General Ranking and Selection Problems with Risk-aversion.
2023 IEEE International Conference on Networking, Sensing and Control (ICNSC)(2023)
摘要
In simulation optimization, a ranking and selection (R&S) problem aims to select the best from candidate solutions, subject to a limited budget of simulation runs. Existing R&S literature focuses on selecting the best solution, based on a ranking criterion defined by the mean performance. Ignoring performance variance in the ranking criterion definition, however, may lead to selecting a very risky solution, with low average performance but high variation. In this paper, we address a new risk-averse R&S problem, which is a generalization of the classic (risk-neutral) R&S problem, by ranking the solutions via the weighted sum of the mean and variance of the performance. For this novel problem, a new approach is developed based on Karush-Kuhn-Tucker conditions, which is a generalization of optimal computing budget allocation (OCBA). Numerical experiments are conducted to show its efficiency.
更多查看译文
关键词
Ranking and selection,Risk-averse,Simulation,Optimal computing budget allocation
AI 理解论文
溯源树
样例
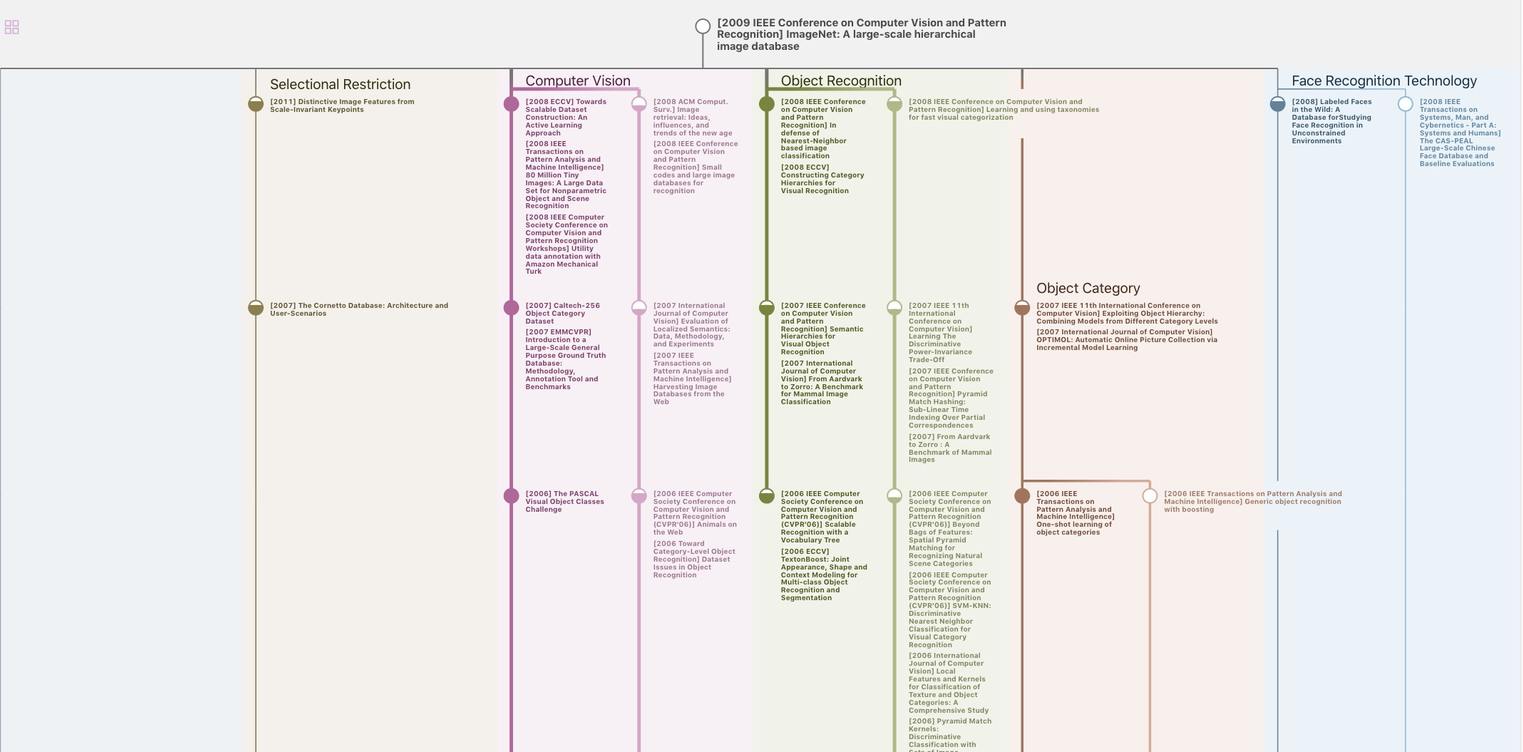
生成溯源树,研究论文发展脉络
Chat Paper
正在生成论文摘要