Energy-Efficient Continual Learning for Autonomous Driving
2023 15th International Conference on Information Technology and Electrical Engineering (ICITEE)(2023)
摘要
Our work highlighted the primary challenges of Autonomous Driving (AD), namely the Catastrophic Forgetting (CF) of previous knowledge by the AD system upon new scenario encounters. Considering the infeasible model retraining with past data given computational, power, and storage constraints on the embedded device, we proposed an experiment featuring Avalanche Continual Learning (CL) training strategies to investigate which strategies excel in this task and combine the promising ones in the hope for a more balanced and efficient trade-off between performance and energy consumption. Our experiment unprecedentedly validated the candidates against a new benchmark introducing natural distribution change and time correlation between input images. We found that although a synergy of CL strategies yields higher resistance towards CF, the slight accuracy gain is not worth the additional computation when we account for energy consumption, rendering a simple Replay strategy the best solution for the Continual Learning benchmark for Autonomous Driving: Online Continual Classification (CLAD-C). Our proposal delivers a 65.80% improvement over the baseline at our proposed accuracy-power ratio metric.
更多查看译文
关键词
Autonomous driving,continual learning,catastrophic forgetting,energy efficiency,lifelong learning
AI 理解论文
溯源树
样例
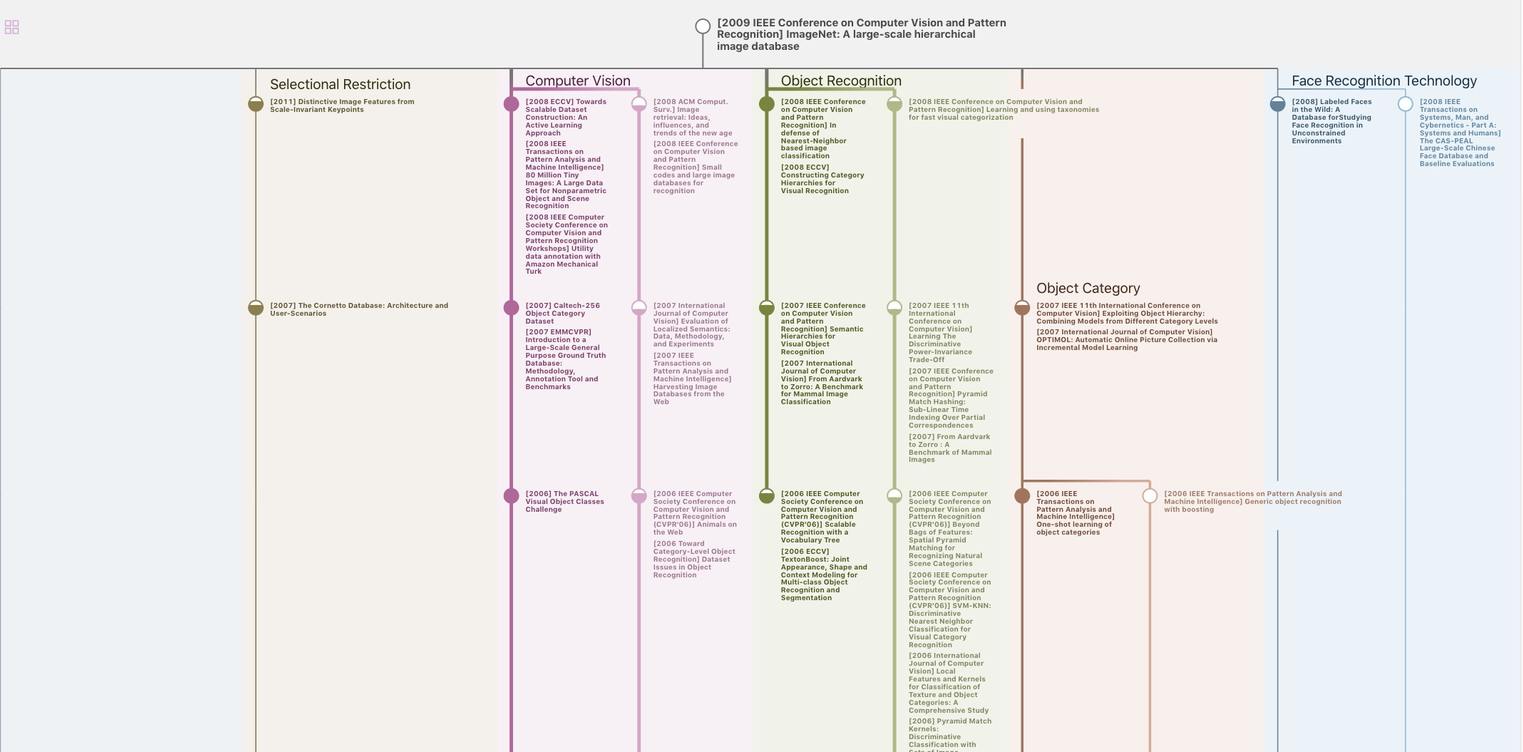
生成溯源树,研究论文发展脉络
Chat Paper
正在生成论文摘要