Reinforcement Learning-Based Power Control for Reliable Mission-Critical Wireless Transmission
IEEE INTERNET OF THINGS JOURNAL(2023)
摘要
In this article, we investigate sequential power allocation over fast varying channels for mission-critical applications, aiming to minimize the expected sum power while guaranteeing the transmission success probability. In particular, a reinforcement learning framework is constructed with appropriate reward design so that the optimal policy maximizes the Lagrangian of the primal problem, where the maximizer of the Lagrangian is shown to have several good properties. For the model-based case, a fast converging algorithm is proposed to find the optimal Lagrange multiplier and thus the corresponding optimal policy. For the model-free case, we develop a three-stage strategy, composed in order of online sampling, offline learning, and online operation, where a backward Q-learning with full exploitation of sampled channel realizations is designed to accelerate the learning process. According to our simulation, the proposed reinforcement learning framework can solve the primal optimization problem from the dual perspective. Moreover, the model-free strategy achieves a performance close to that of the optimal model-based algorithm.
更多查看译文
关键词
Policy optimization,power control,Q-learning,reinforcement learning,reliability
AI 理解论文
溯源树
样例
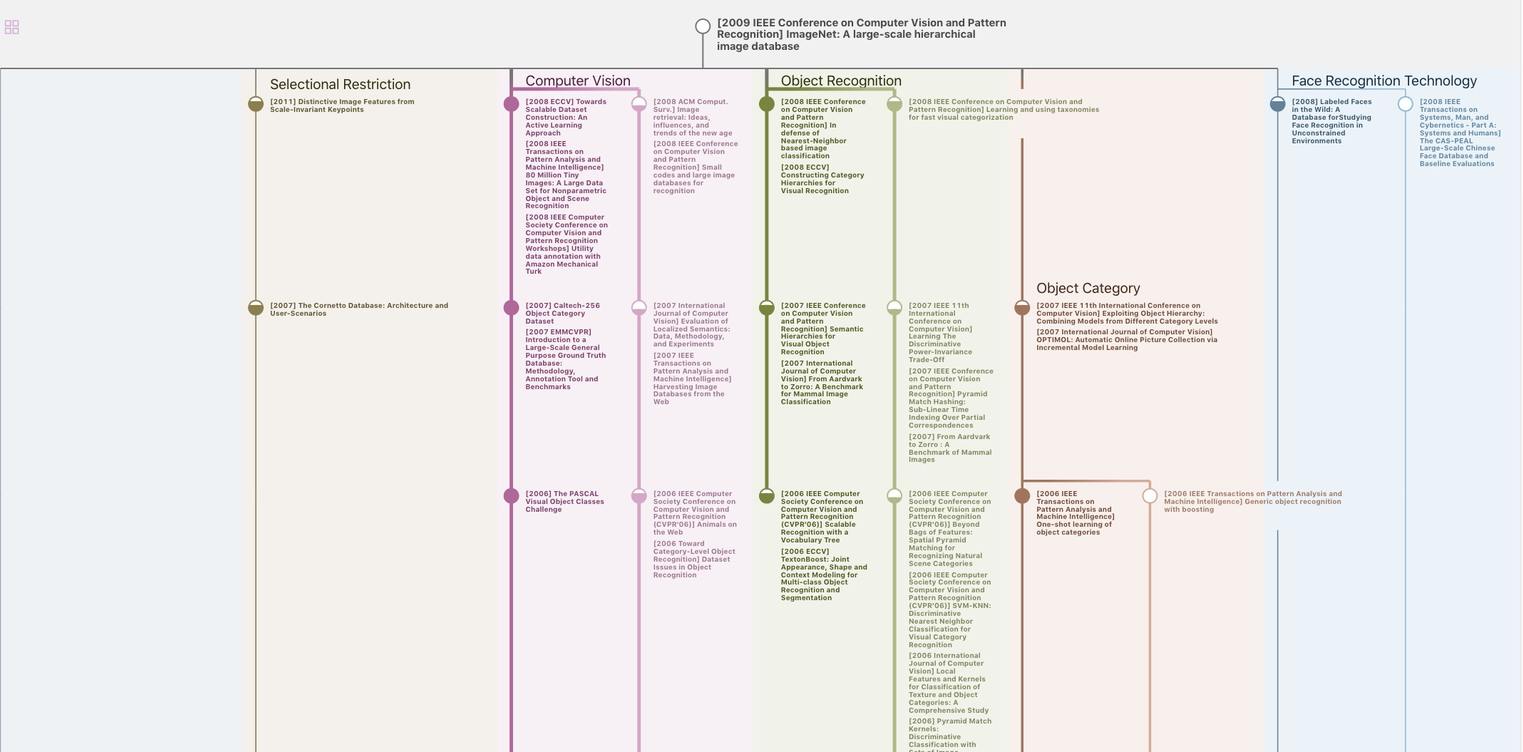
生成溯源树,研究论文发展脉络
Chat Paper
正在生成论文摘要