Chameleon
Computers and Security(2022)
摘要
• An approach for efficient feature selection on networking and intrusion detection datasets, using swarm intelligence and ensemble methods. • The impact of feature selection on the performance of anomaly detection models using neural networks. • Improvement of deep learning anomaly detection performance on IDS benchmark datasets compared with state-of-the art approaches. In this paper, we propose an optimization approach by leveraging swarm intelligence and ensemble methods to solve the non-deterministic feature selection problem. The proposed approach is validated on two benchmark datasets, namely, NSL-KDD and UNSW-NB15, in addition to a third dataset, called IoT-Zeek dataset, which consists of Zeek network-based intrusion detection connection logs. We build the IoT-Zeek dataset by employing ensemble classification and deep learning models using publicly available malicious and benign threat intelligence on the Zeek connection logs of IoT devices. Moreover, we deploy and validate a deep learning-based anomaly detection model using autoencoders on each of the aforementioned datasets by utilizing the selected features obtained from the proposed optimization approach. The obtained results demonstrate that our approach outperform the existing state-of-the-art machine learning models in terms of f 1 score results, with 92.092% f 1 score on NSL-KDD dataset, 92.904 f 1 score on UNSW-NB15 dataset, and 97.302 f 1 score on IoT-Zeek dataset.
更多查看译文
关键词
Feature selection,Swarm intelligence,Particle swarm optimization (PSO),Ensemble methods,Internet of things (IoT),Network anomaly detection,Deep learning
AI 理解论文
溯源树
样例
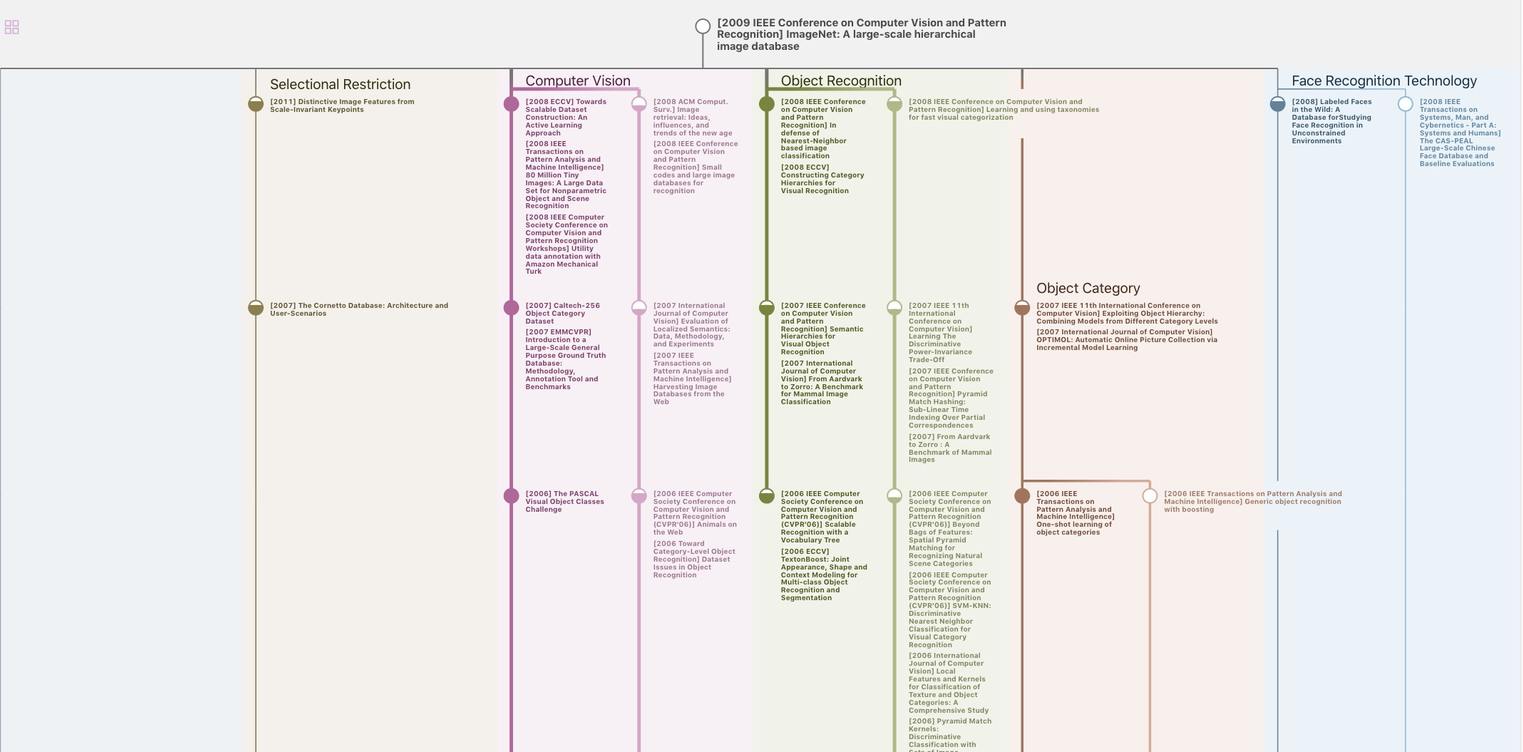
生成溯源树,研究论文发展脉络
Chat Paper
正在生成论文摘要