Spatial Confounding and Spatial+ for Nonlinear Covariate Effects
Journal of Agricultural, Biological and Environmental Statistics(2023)
摘要
Regression models for spatially varying data use spatial random effects to reflect spatial correlation structure. Such random effects, however, may interfere with the covariate effect estimates and make them unreliable. This problem, known as spatial confounding, is complex and has only been studied for models with linear covariate effects. However, as illustrated by a forestry example in which we assess the effect of soil, climate, and topography variables on tree health, the covariate effects of interest are in practice often unknown and nonlinear. We consider, for the first time, spatial confounding in spatial models with nonlinear effects implemented in the generalised additive models (GAMs) framework. We show that spatial+, a recently developed method for alleviating confounding in the linear case, can be adapted to this setting. In practice, spatial+ can then be used both as a diagnostic tool for investigating whether covariate effect estimates are affected by spatial confounding and for correcting the estimates for the resulting bias when it is present. Supplementary materials accompanying this paper appear online.
更多查看译文
关键词
Bias reduction,Forest health,Generalised additive models,Smoothing,Spatial regression
AI 理解论文
溯源树
样例
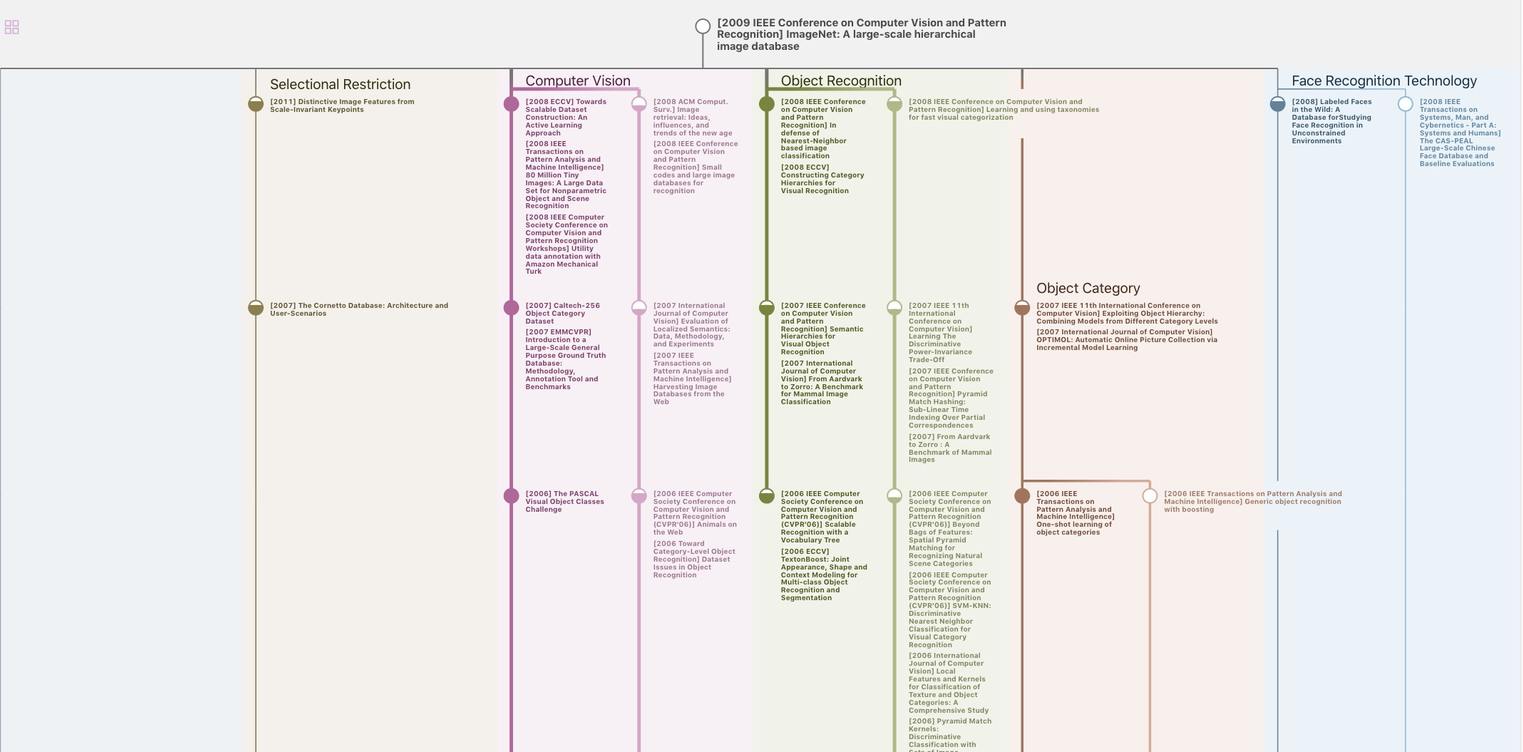
生成溯源树,研究论文发展脉络
Chat Paper
正在生成论文摘要