The YOLO model that still excels in document layout analysis
SIGNAL IMAGE AND VIDEO PROCESSING(2023)
摘要
Document layout analysis can help people better understand and use the information in a document. However, the diversity of document layouts and considerable variation in aspect ratios among document objects pose significant challenges. In this study, we designed the multi-convolutional deformable separation (MCDS) module as the main structure of the network, using the YOLO model as a baseline. Integration of this module into the backbone and neck layers enhances the image feature extraction process significantly. Moreover, we incorporate ParNet-Attention to direct the network's focus toward document objects through parallel networks, thereby facilitating a more exhaustive feature extraction. To optimize the model's predictive potential, the decouple fusion head is employed within the head layer. This technique leverages multi-scale features based on the decoupled head, thereby enhancing the accuracy of predictions. Our proposed model achieves remarkable performance on three distinct public datasets with varying characteristics, namely ICDAR-POD, PubLayNet, and IIIT-AR-13K. Notably, in ICDAR-POD, both IoU(0.6) and IoU(0.8) achieve the optimal mean Average Precision (mAP), 96.2 and 94.4, respectively.
更多查看译文
关键词
Document layout analysis,Document object detection,Deep learning,Feature extraction,Multi-scale features
AI 理解论文
溯源树
样例
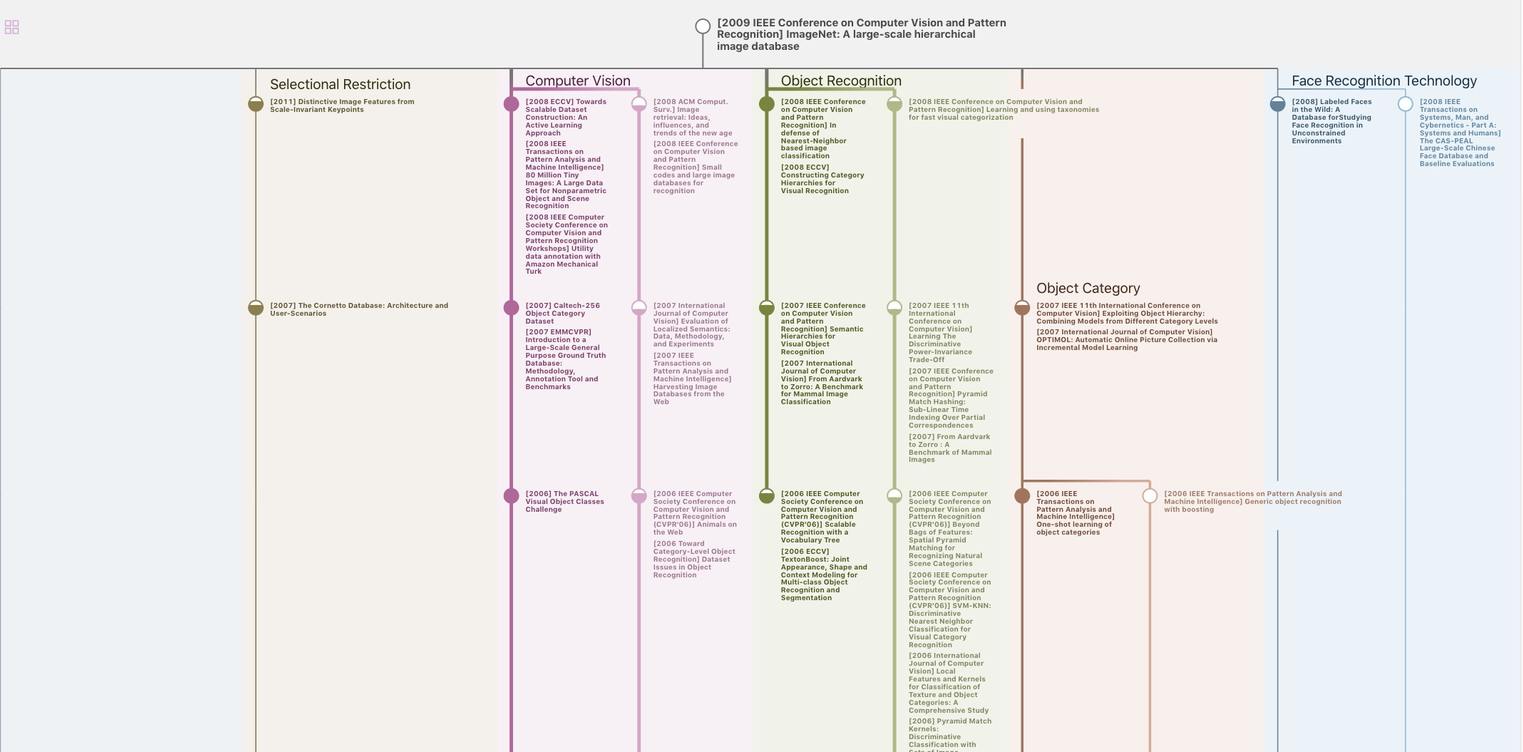
生成溯源树,研究论文发展脉络
Chat Paper
正在生成论文摘要