Phase-Amplitude Coordinate-Based Neural Networks for Inferring Oscillatory Dynamics
Journal of Nonlinear Science(2024)
摘要
The dynamics of a periodic nonlinear system can be represented accurately beyond the limit cycle in a reduced-order phase-amplitude coordinate-based model reduction framework. When only observable time series data is available, data-driven strategies must be employed for model inference. In this work, we propose a data-driven approach that can predict the unknown, periodic terms of a phase-amplitude coordinate-based reduced-order model by considering their Fourier series expansions and reframing the terms as a composition of a known nonlinear function with an unknown linear function. These linear functions can be structured as weights of a feed-forward neural network and learned to obtain a reduced-order model representation valid to arbitrary orders of accuracy in an expansion of amplitude coordinates by training the network on observable data. The proposed approach can be used in conjunction with other recently developed reduced-order modeling approaches to yield very high accuracy reduced-order models. The proposed strategy is illustrated in a variety of examples that consider the dynamics of a synaptically coupled neuronal population.
更多查看译文
关键词
Model identification,Periodic orbit,Artificial neural networks,Phase-isostable coordinates,Dynamical systems,Model order reduction
AI 理解论文
溯源树
样例
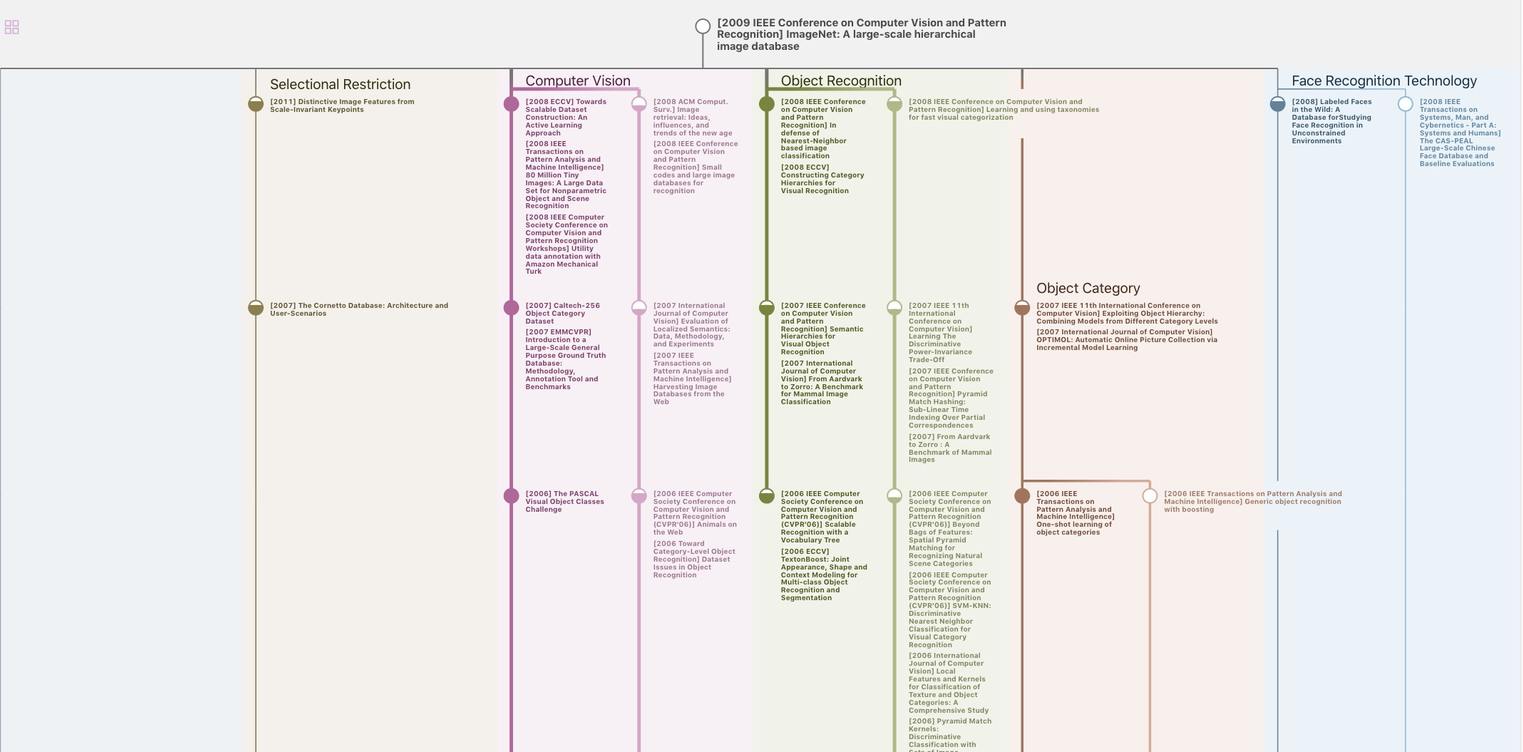
生成溯源树,研究论文发展脉络
Chat Paper
正在生成论文摘要