Online data-enabled predictive control
Automatica (Journal of IFAC)(2022)
摘要
We develop an online data-enabled predictive (ODeePC) control method for optimal control of unknown systems, building on the recently proposed DeePC (Coulson et al., 2019). Our proposed ODeePC method leverages a primal–dual algorithm with real-time measurement feedback to iteratively compute the corresponding real-time optimal control policy as system conditions change. The proposed ODeePC conceptual-wise resembles standard adaptive system identification and model predictive control (MPC), but it provides a new alternative for the standard methods. ODeePC is enabled by computationally efficient methods that exploit the special structure of the Hankel matrices in the context of DeePC with Fast Fourier Transform (FFT) and primal–dual algorithm We provide theoretical guarantees regarding the asymptotic behavior of ODeePC, and we demonstrate its performance through numerical examples.
更多查看译文
关键词
Data-driven control,Model predictive control,Online optimization
AI 理解论文
溯源树
样例
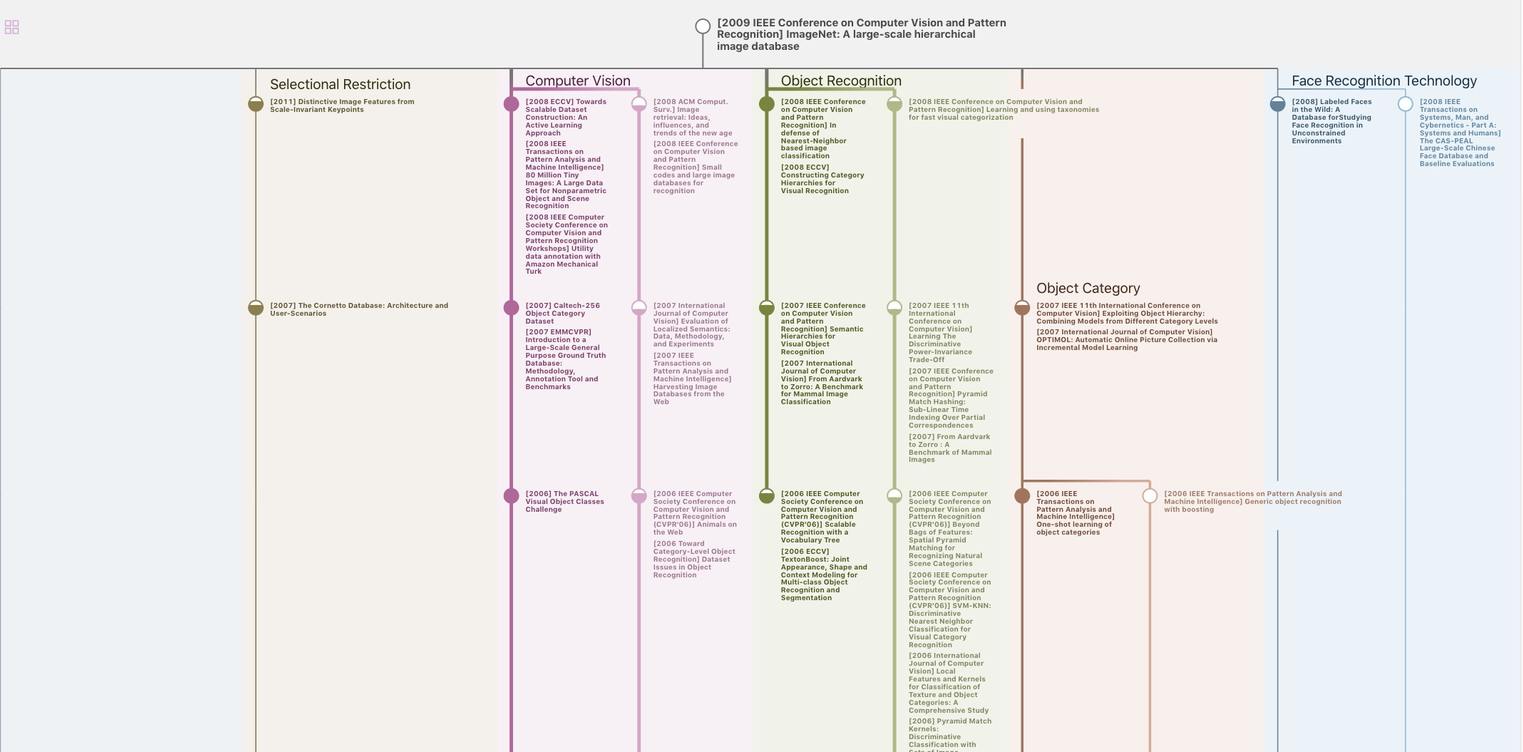
生成溯源树,研究论文发展脉络
Chat Paper
正在生成论文摘要