ADAPTER-RL: Adaptation of Any Agent using Reinforcement Learning.
CoRR(2023)
摘要
Deep Reinforcement Learning (DRL) agents frequently face challenges in
adapting to tasks outside their training distribution, including issues with
over-fitting, catastrophic forgetting and sample inefficiency. Although the
application of adapters has proven effective in supervised learning contexts
such as natural language processing and computer vision, their potential within
the DRL domain remains largely unexplored. This paper delves into the
integration of adapters in reinforcement learning, presenting an innovative
adaptation strategy that demonstrates enhanced training efficiency and
improvement of the base-agent, experimentally in the nanoRTS environment, a
real-time strategy (RTS) game simulation. Our proposed universal approach is
not only compatible with pre-trained neural networks but also with rule-based
agents, offering a means to integrate human expertise.
更多查看译文
AI 理解论文
溯源树
样例
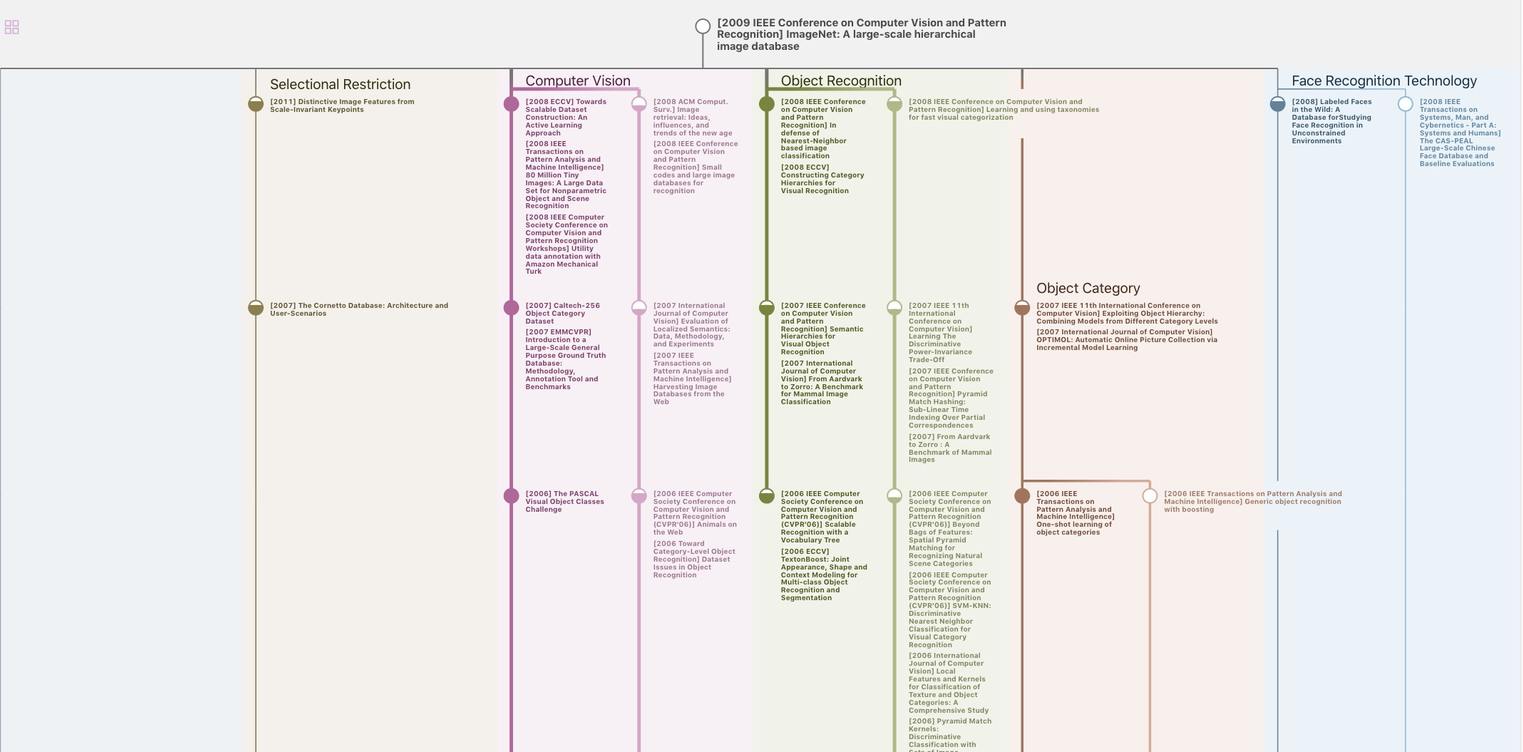
生成溯源树,研究论文发展脉络
Chat Paper
正在生成论文摘要