HungerGist: An Interpretable Predictive Model for Food Insecurity.
CoRR(2023)
摘要
The escalating food insecurity in Africa, caused by factors such as war,
climate change, and poverty, demonstrates the critical need for advanced early
warning systems. Traditional methodologies, relying on expert-curated data
encompassing climate, geography, and social disturbances, often fall short due
to data limitations, hindering comprehensive analysis and potential discovery
of new predictive factors. To address this, this paper introduces "HungerGist",
a multi-task deep learning model utilizing news texts and NLP techniques. Using
a corpus of over 53,000 news articles from nine African countries over four
years, we demonstrate that our model, trained solely on news data, outperforms
the baseline method trained on both traditional risk factors and human-curated
keywords. In addition, our method has the ability to detect critical texts that
contain interpretable signals known as "gists." Moreover, our examination of
these gists indicates that this approach has the potential to reveal latent
factors that would otherwise remain concealed in unstructured texts.
更多查看译文
关键词
food insecurity,food crisis,interpretability,deep learning
AI 理解论文
溯源树
样例
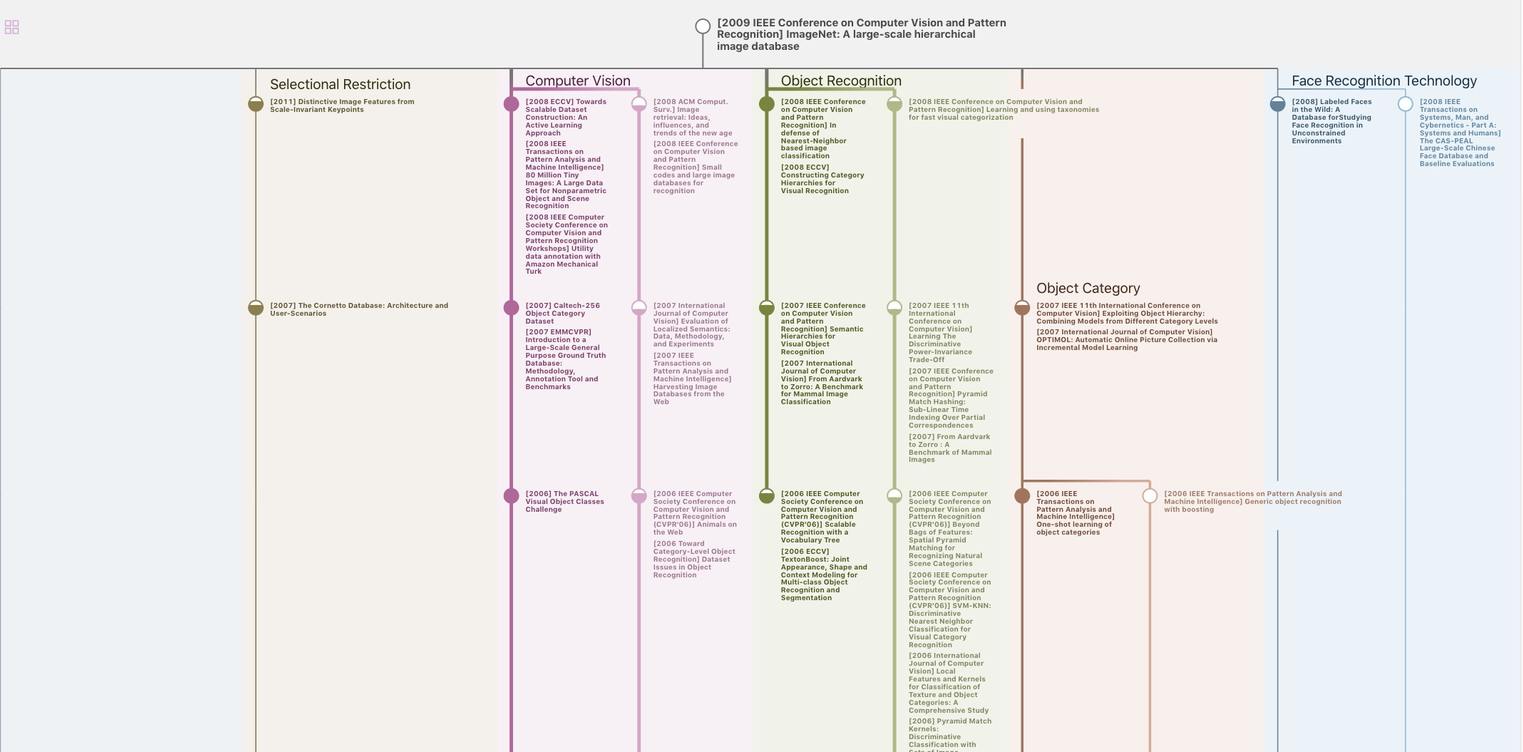
生成溯源树,研究论文发展脉络
Chat Paper
正在生成论文摘要