HIDRO-VQA: High Dynamic Range Oracle for Video Quality Assessment
2024 IEEE/CVF Winter Conference on Applications of Computer Vision Workshops (WACVW)(2023)
摘要
We introduce HIDRO-VQA, a no-reference (NR) video quality assessment model
designed to provide precise quality evaluations of High Dynamic Range (HDR)
videos. HDR videos exhibit a broader spectrum of luminance, detail, and color
than Standard Dynamic Range (SDR) videos. As HDR content becomes increasingly
popular, there is a growing demand for video quality assessment (VQA)
algorithms that effectively address distortions unique to HDR content. To
address this challenge, we propose a self-supervised contrastive fine-tuning
approach to transfer quality-aware features from the SDR to the HDR domain,
utilizing unlabeled HDR videos. Our findings demonstrate that self-supervised
pre-trained neural networks on SDR content can be further fine-tuned in a
self-supervised setting using limited unlabeled HDR videos to achieve
state-of-the-art performance on the only publicly available VQA database for
HDR content, the LIVE-HDR VQA database. Moreover, our algorithm can be extended
to the Full Reference VQA setting, also achieving state-of-the-art performance.
Our code is available publicly at https://github.com/avinabsaha/HIDRO-VQA.
更多查看译文
关键词
Quality Assessment,Dynamic Range,High Dynamic Range,Video Quality,Video Quality Assessment,Model Quality Assessment,Temporal Features,Data Preparation,Video Clips,Color Space,Quality Of Experience,Temperature Parameters,User-generated Content,Unlabeled Data,Support Vector Regression,Self-supervised Learning,CRT Monitor,Spearman Rank-order Correlation,Mean Opinion Score,Source Video,Natural Scene Statistics,ImageNet Pre-trained Model,Bitrate,Color Gamut,YouTube,Fine-tuning Process,Multilayer Perceptron,Projector
AI 理解论文
溯源树
样例
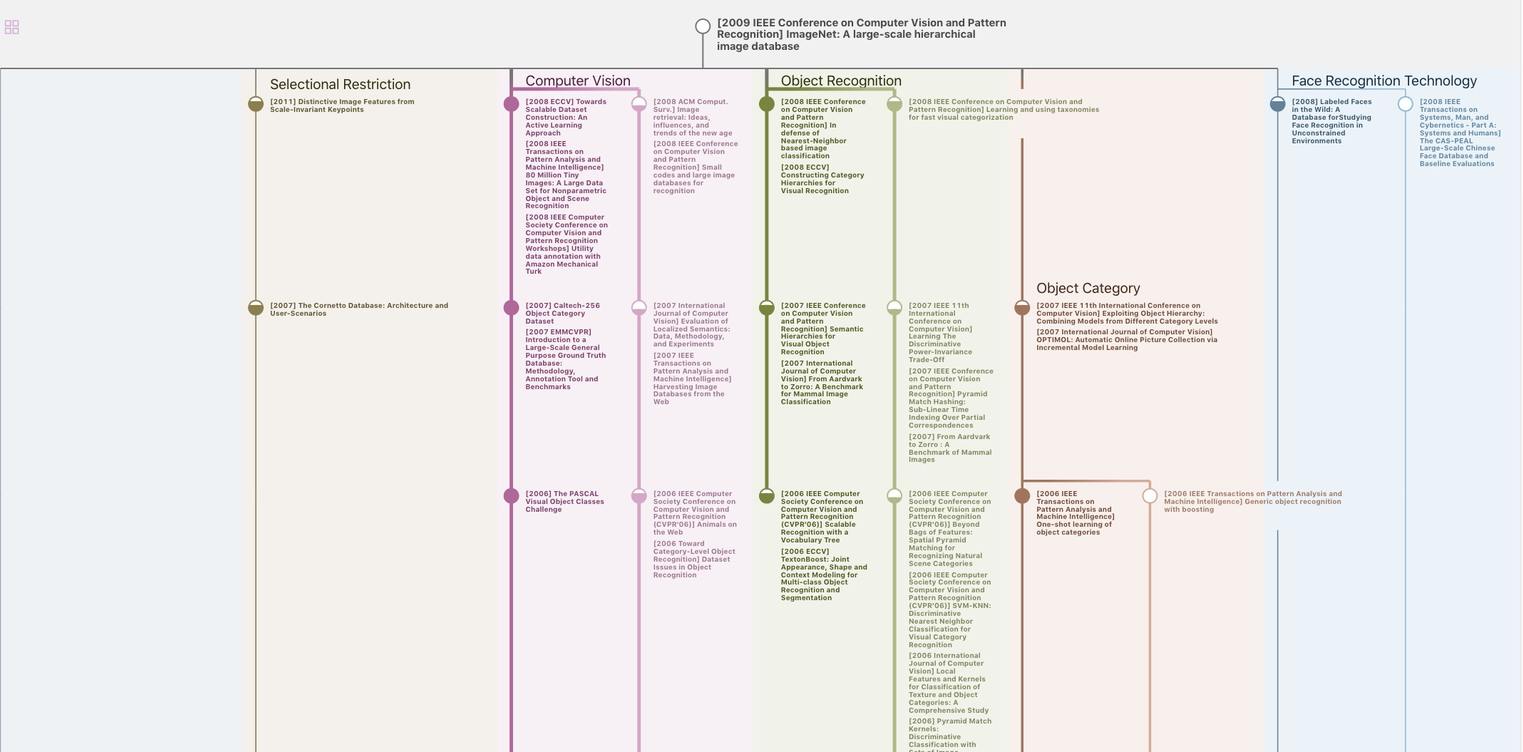
生成溯源树,研究论文发展脉络
Chat Paper
正在生成论文摘要