Quantifying the potential benefits of early detection for pancreatic cancer through a counterfactual simulation modeling analysis
Scientific Reports(2023)
摘要
Abstract The benefits of cancer early detection depend on various factors, including cancer type, screening method performance, stage at diagnosis, and subsequent treatment. Although numerous studies have evaluated the effectiveness of screening interventions for identifying cancer at earlier stages, there is no quantitative analysis that studies the optimal early detection time interval that results in the greatest mortality benefit; such data could serve as a target and benchmark for cancer early detection strategies. In this study, we focus on pancreatic ductal adenocarcinoma (PDAC), a cancer known for its lack of early symptoms. Consequently, it is most often detected at late stages when the 5-year survival rate is only 3%. We developed a PDAC population model that simulates an individual patient's age and stage at diagnosis, while replicating overall US cancer incidence and mortality rates. The model includes “cancer sojourn time,” serving as a proxy for the speed of cancer progression, with shorter times indicating rapid progression and longer times indicating slower progression. In our PDAC model, our hypothesis was that earlier cancer detection, potentially through a hypothetical screening intervention in the counterfactual analysis, would yield reduced mortality as compared to a no-screening group. We found that the benefits of early detection, such as increased life-years gained, are greater when the sojourn time is shorter, reaching their maximum when identification is made 4–6 years prior to clinical diagnosis (e.g., when a symptomatic diagnosis is made). However, when early detection occurs even earlier, for example 6–10 years prior to clinical diagnosis, the benefits significantly diminish for shorter sojourn time cancers, and level off for longer sojourn time cancers. Our study clarifies the potential benefits of PDAC early detection that explicitly incorporates individual patient heterogeneity in cancer progression and identifies quantitative benchmarks for future interventions.
更多查看译文
AI 理解论文
溯源树
样例
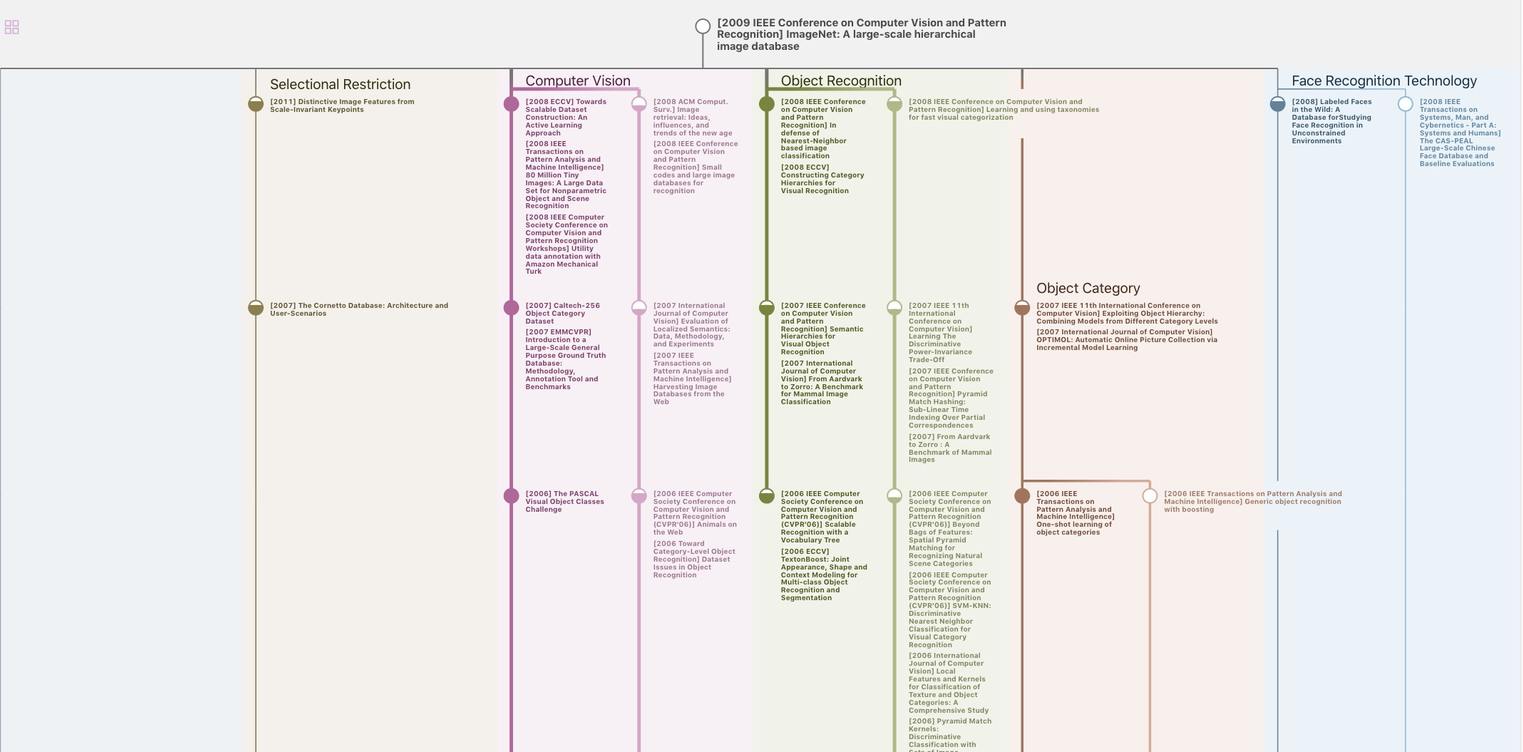
生成溯源树,研究论文发展脉络
Chat Paper
正在生成论文摘要